Not All Tasks Are Equal: A Parameter-Efficient Task Reweighting Method for Few-Shot Learning
MACHINE LEARNING AND KNOWLEDGE DISCOVERY IN DATABASES: RESEARCH TRACK, ECML PKDD 2023, PT II(2023)
摘要
Meta-learning has emerged as an effective and popular approach for few-shot learning (FSL) due to its fast adaptation to novel tasks. However, this kind of method assumes that the meta-training and testing tasks come from the same task distribution and assigns equal weights to all tasks during meta-training. This assumption limits their ability to perform well in real-world scenarios where some meta-training tasks contribute more to the testing tasks than others. To address this issue, we propose a parameter-efficient task reweighting (PETR) method, which assigns proper weights to meta-training tasks according to their contribution to the testing tasks while using few parameters. Specifically, we formulate a bi-level optimization problem to jointly learn the few-shot learning model and the task weights. In the inner loop, the meta-parameters of the few-shot learning model are updated based on a weighted training loss. In the outer loop, the task weight parameters are updated with the implicit gradient. Additionally, to address the challenge of a large number of task weight parameters, we introduce a hypothesis that significantly reduces the required parameters by considering the factors that influence the importance of each meta-training task. Empirical evaluation results on both traditional FSL and FSL with out-of-distribution (OOD) tasks show that our PETR method outperforms state-of-the-art meta-learning-based FSL methods by assigning proper weights to different meta-training tasks.
更多查看译文
关键词
Few-shot Learning,Meta Learning,Task Reweighting,Bi-level Optimization
AI 理解论文
溯源树
样例
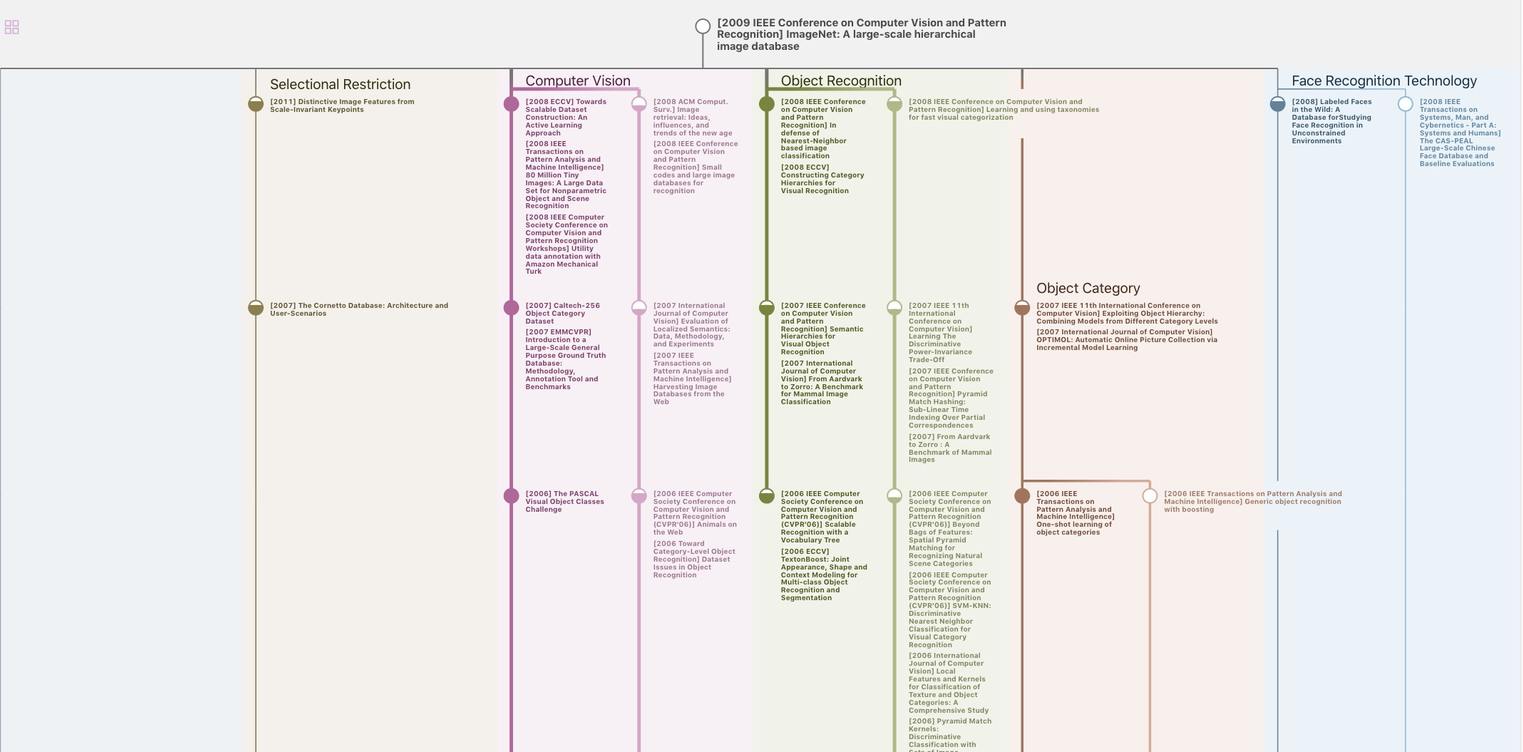
生成溯源树,研究论文发展脉络
Chat Paper
正在生成论文摘要