XAI with Machine Teaching When Humans Are (Not) Informed About the Irrelevant Features
MACHINE LEARNING AND KNOWLEDGE DISCOVERY IN DATABASES: RESEARCH TRACK, ECML PKDD 2023, PT III(2023)
摘要
Exemplar-based explainable artificial intelligence (XAI) aims at creating human understanding about the behaviour of an AI system, usually a machine learning model, through examples. The advantage of this approach is that the human creates their own explanation in their own internal language. However, what examples should be chosen? Existing frameworks fall short in capturing all the elements that contribute to this process. In this paper, we propose a comprehensive XAI framework based on machine teaching. The traditional trade-off between the fidelity and the complexity of the explanation is transformed here into a trade-off between the complexity of the examples and the fidelity the human achieves about the behaviour of the ML system to be explained. We analyse a concept class of Boolean functions that is learned by a convolutional neural network classifier over a dataset of images of possibly rotated and resized letters. We assume the human learner has a strong prior (Karnaugh maps over Boolean functions). Our explanation procedure then behaves like a machine teaching session optimising the trade-off between examples and fidelity. We include an experimental evaluation and several human studies where we analyse the capacity of teaching humans these Boolean function by means of the explanatory examples generated by our framework. We explore the effect of telling the essential features to the human and the priors, and see that the identification is more successful than by randomly sampling the examples.
更多查看译文
AI 理解论文
溯源树
样例
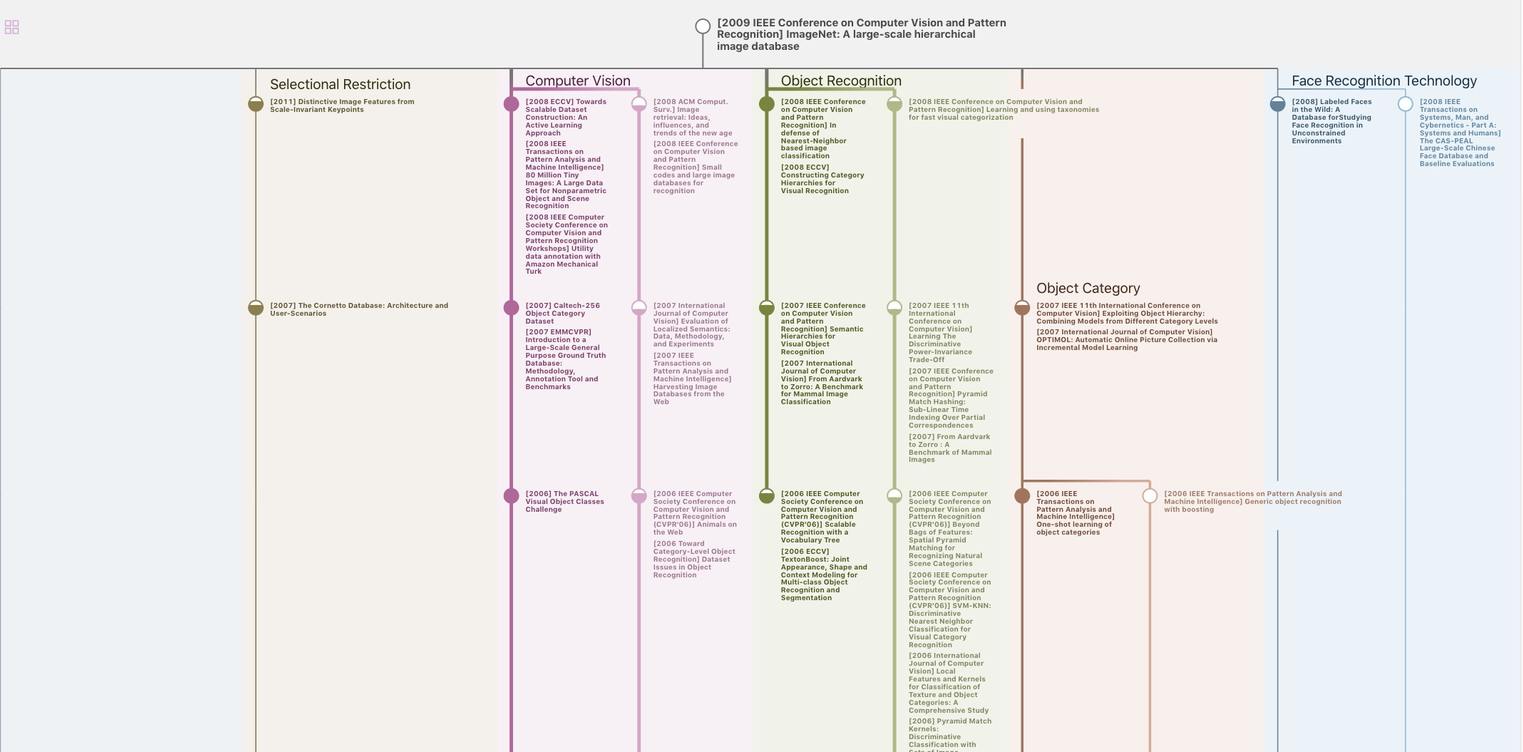
生成溯源树,研究论文发展脉络
Chat Paper
正在生成论文摘要