Clifford Embeddings - A Generalized Approach for Embedding in Normed Algebras
MACHINE LEARNING AND KNOWLEDGE DISCOVERY IN DATABASES: RESEARCH TRACK, ECML PKDD 2023, PT III(2023)
摘要
A growing number of knowledge graph embedding models exploit the characteristics of division algebras (e.g., R, C, H, and O) to learn embeddings. Yet, recent empirical results suggest that the suitability of algebras is contingent upon the knowledge graph being embedded. In this work, we tackle the challenge of selecting the algebra within which a given knowledge graph should be embedded by exploiting the fact that Clifford algebras Cl-p,Cl-q generalize over R, C, H, and O. Our embedding approach, KECI, is the first knowledge graph embedding model that can parameterize the algebra within which it operates. With KECI, the selection of an underlying algebra becomes a part of the learning process. Specifically, KECI starts the training process by learning real-valued embeddings for entities and relations in R-m = Cl-0,0(m). At each mini-batch update, KECI can steer the training process from Cl-p,q(m) to Cl-p+1,q(m) or Cl-p,q+1(m) by processing the training loss. In this way, KECI can decide the algebra within which it operates in a data-driven fashion. Consequently, KECI is a generalization of previous approaches such as DistMult, ComplEx, QuatE, and OMult. Our evaluation suggests that KECI outperforms state-of-the-art embedding approaches on seven benchmark datasets. We provide an open-source implementation of KECI, including pre-trained models, training and evaluation scripts (https://github.com/dice-group/dice-embeddings).
更多查看译文
关键词
Knowledge Graphs,Embeddings,Theory Unification
AI 理解论文
溯源树
样例
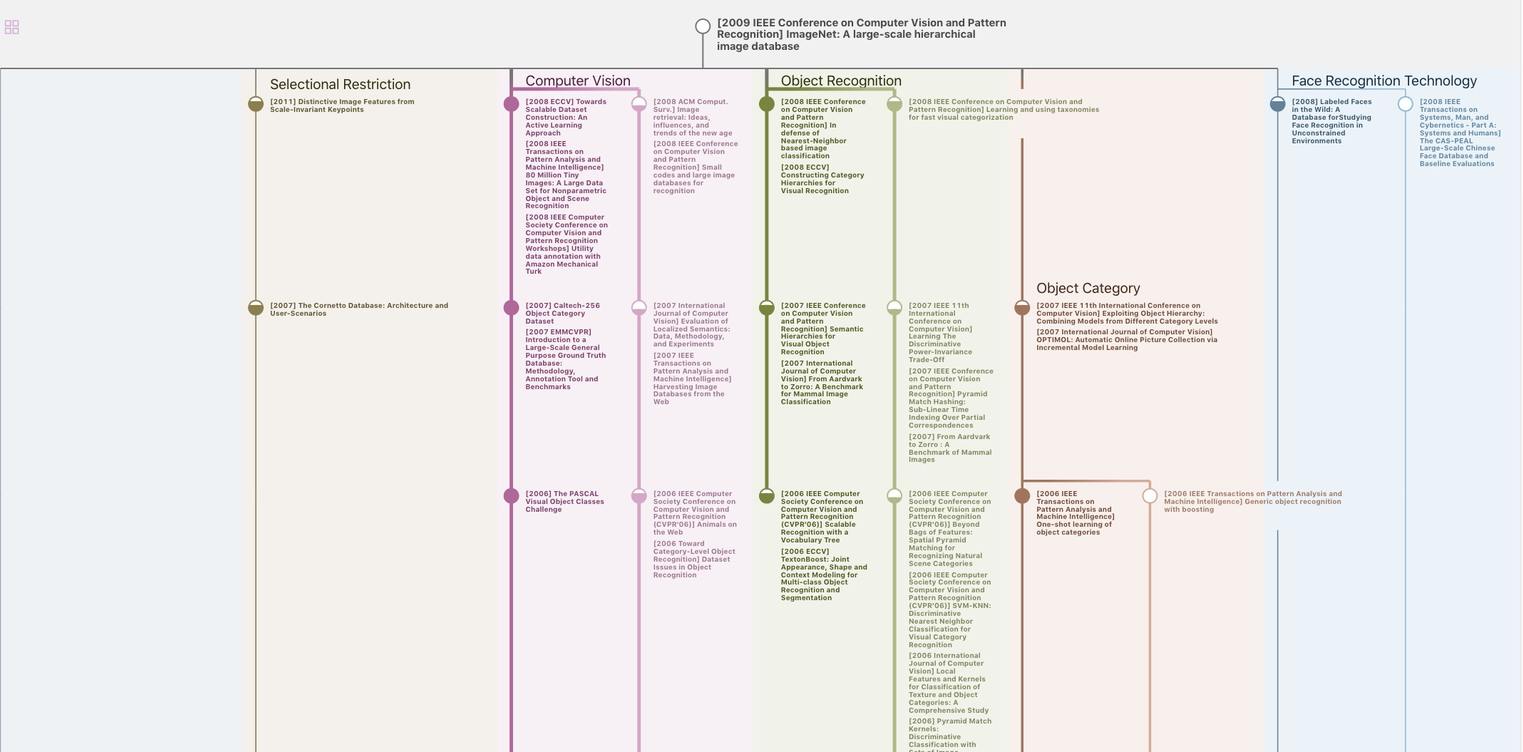
生成溯源树,研究论文发展脉络
Chat Paper
正在生成论文摘要