Future Augmentation with Self-distillation in Recommendation
MACHINE LEARNING AND KNOWLEDGE DISCOVERY IN DATABASES: APPLIED DATA SCIENCE AND DEMO TRACK, ECML PKDD 2023, PT VI(2023)
摘要
Sequential recommendation (SR) aims to provide appropriate items a user will click according to the user's historical behavior sequence. Conventional SR models are trained under the next item prediction task, and thus should deal with two challenges, including the data sparsity of user feedback and the variability and irregularity of user behaviors. Different from natural language sequences in NLP, user behavior sequences in recommendation are much more personalized, irregular, and unordered. Therefore, the current user preferences extracted from user historical behaviors may also have correlations with the next-k (i.e., future clicked) items besides the classical next-1 (i.e., current clicked) item to be predicted. Inspired by this phenomenon, we propose a novel Future augmentation with self-distillation in recommendation (FASRec). It considers future clicked items as augmented positive signals of the current clicks in training, which addresses both data sparsity and behavior irregularity and variability issues. To denoise these augmented future clicks, we further adopt a self-distillation module with the exponential moving average strategy, considering soft labels of self-distillation as confidence for more accurate augmentations. In experiments, FASRec achieves significant and consistent improvements on both offline and online evaluations with different base SR models, confirming its effectiveness and universality. FASRec has been deployed on a widely-used recommendation feed in Tencent. The source codes are in https://github.com/FASRec/FASRec.
更多查看译文
关键词
Future augmentation,Self-distillation,Recommendation
AI 理解论文
溯源树
样例
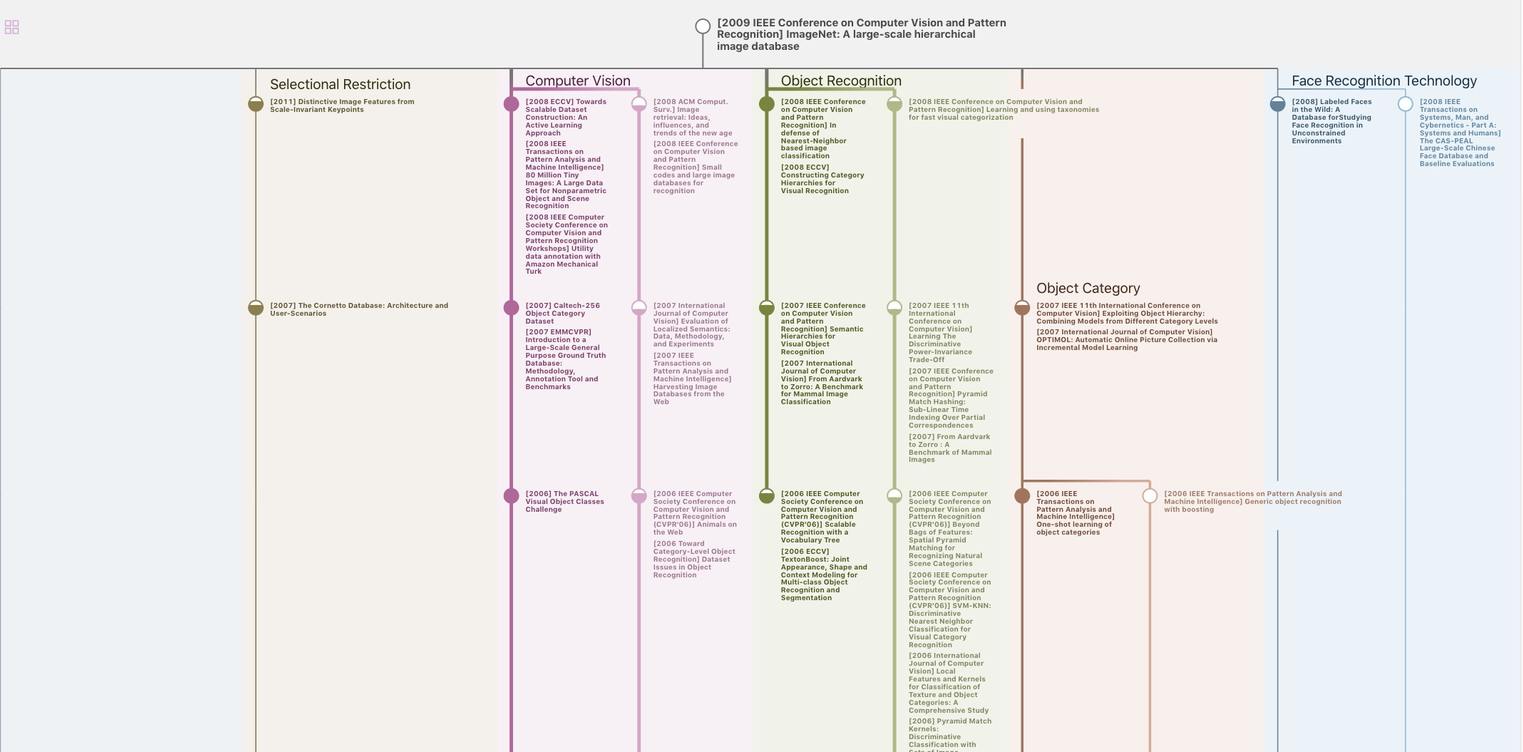
生成溯源树,研究论文发展脉络
Chat Paper
正在生成论文摘要