Equivariant Representation Learning in the Presence of Stabilizers
MACHINE LEARNING AND KNOWLEDGE DISCOVERY IN DATABASES: RESEARCH TRACK, ECML PKDD 2023, PT IV(2023)
摘要
We introduce Equivariant Isomorphic Networks (EquIN) a method for learning representations that are equivariant with respect to general group actions over data. Differently from existing equivariant representation learners, EquIN is suitable for group actions that are not free, i.e., that stabilize data via nontrivial symmetries. EquIN is theoretically grounded in the orbit-stabilizer theorem from group theory. This guarantees that an ideal learner infers isomorphic representations while trained on equivariance alone and thus fully extracts the geometric structure of data. We provide an empirical investigation on image datasets with rotational symmetries and show that taking stabilizers into account improves the quality of the representations.
更多查看译文
关键词
Representation Learning,Equivariance,Lie Groups
AI 理解论文
溯源树
样例
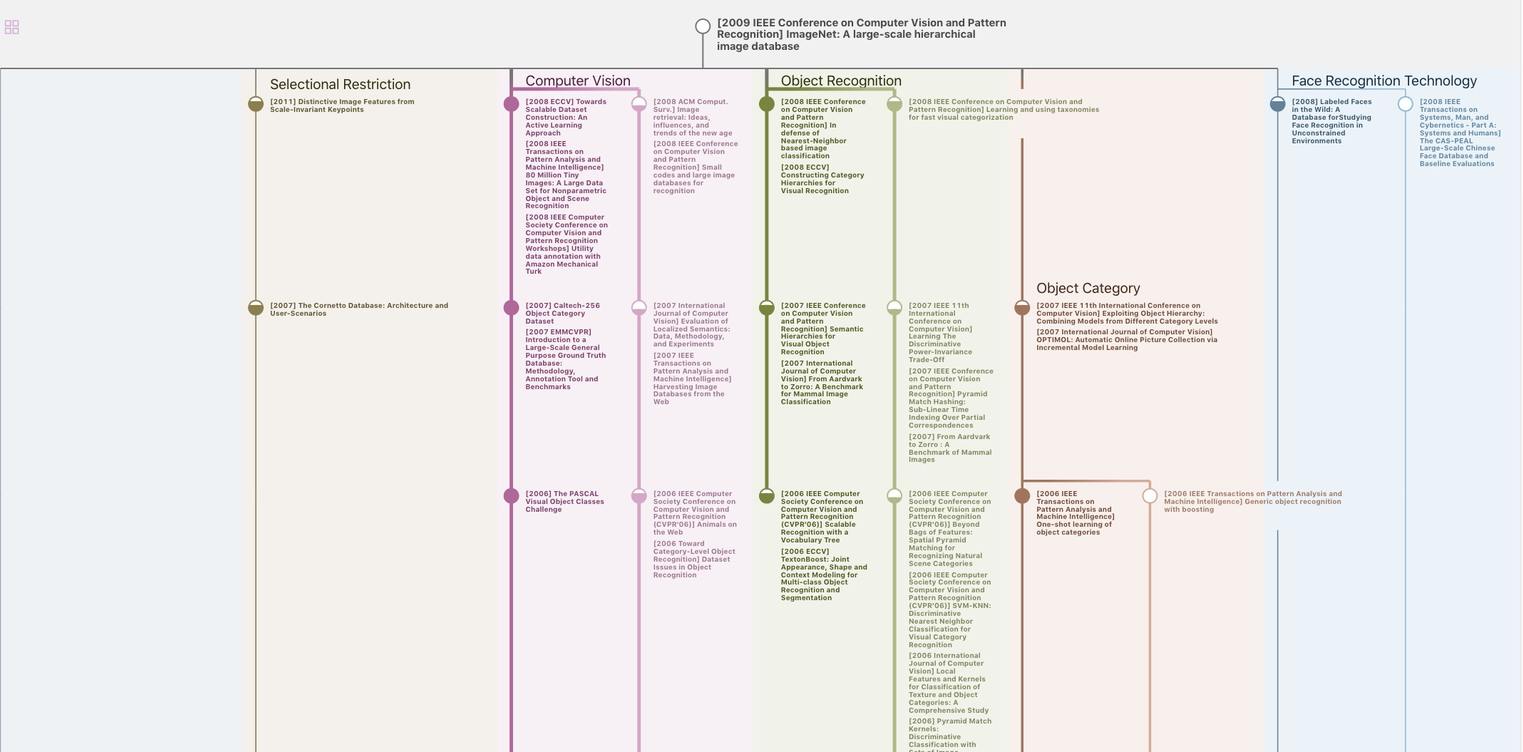
生成溯源树,研究论文发展脉络
Chat Paper
正在生成论文摘要