Estimating Dynamic Time Warping Distance Between Time Series with Missing Data
MACHINE LEARNING AND KNOWLEDGE DISCOVERY IN DATABASES: RESEARCH TRACK, ECML PKDD 2023, PT V(2023)
摘要
Many techniques for analyzing time series rely on some notion of similarity between two time series, such as Dynamic Time Warping (DTW) distance. ButDTWcannot handlemissing values, and simple fixes (e.g., dropping missing values, or interpolating) fail when entire intervals are missing, as is often the case with, e.g., temporary sensor or communication failures. There is hardly any research on how to address this problem. In this paper, we propose two hyperparameter-free techniques to estimate the DTWdistance between time series with missing values. The first technique, DTW-AROW, significantly decreases the impact of missing values on the DTW distance by modifying the optimization problem in the DTW algorithm. The second technique, DTW-CAI, can further improve upon DTW-AROW by exploiting additional contextual information when that is available (more specifically, more time series from the same population). We show that, on multiple datasets, the proposed techniques outperform existing techniques in estimating pairwise DTW distances as well as in classification and clustering tasks based on these distances. The proposed techniques can enable many machine learning algorithms to more accurately handle time series with missing values.
更多查看译文
关键词
dynamic time warping distance,time series
AI 理解论文
溯源树
样例
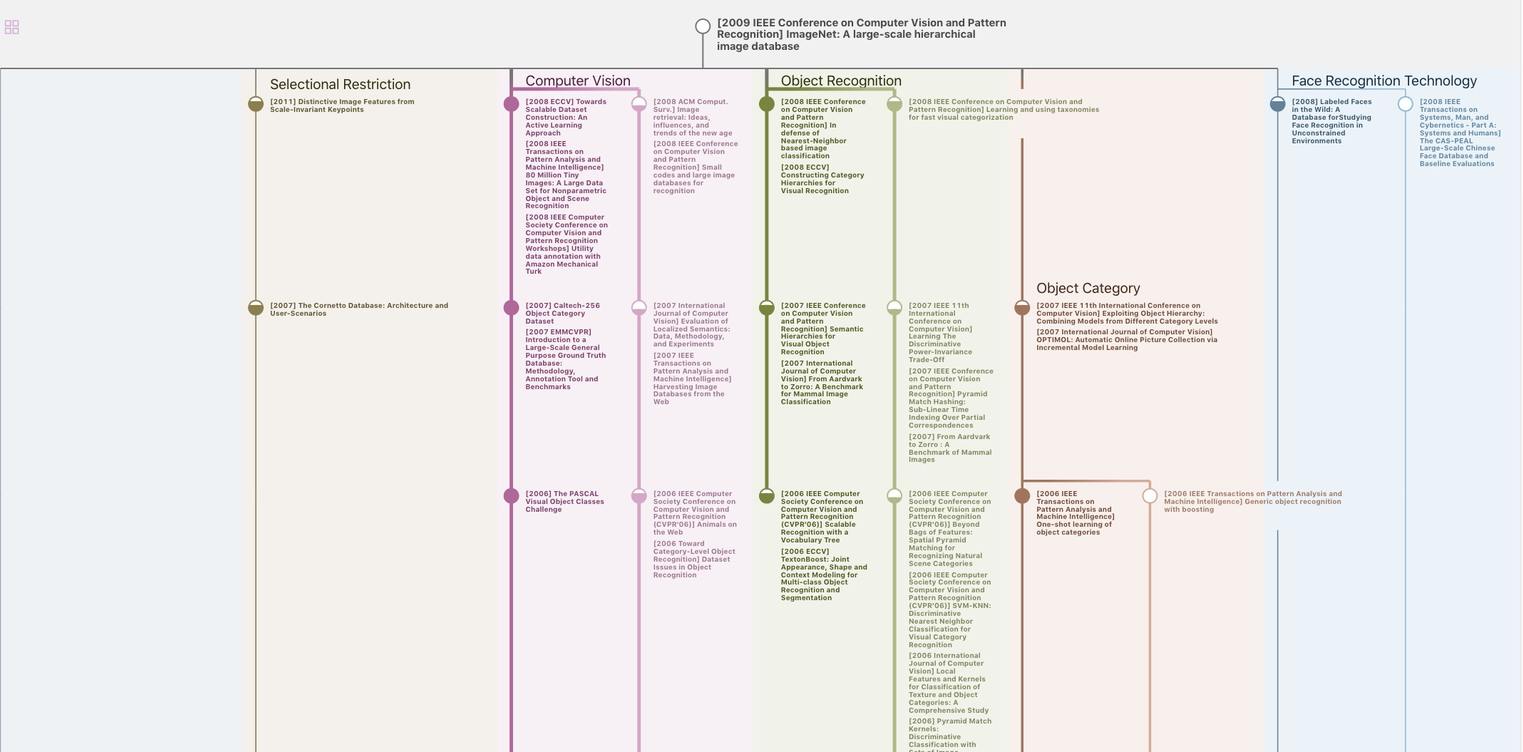
生成溯源树,研究论文发展脉络
Chat Paper
正在生成论文摘要