Multivariate Time-Series Anomaly Detection with Temporal Self-supervision and Graphs: Application to Vehicle Failure Prediction
MACHINE LEARNING AND KNOWLEDGE DISCOVERY IN DATABASES: APPLIED DATA SCIENCE AND DEMO TRACK, ECML PKDD 2023, PT VII(2023)
摘要
Failure prediction is key to ensuring the reliable operation of vehicles, especially for organizations that depend on a fleet of vehicles. However, traditional approaches often rely on rule-based or heuristic methods that may not be effective in detecting subtle anomalies, rare events, or in more modern vehicles containing a complex sensory network. This paper presents a novel approach to vehicle failure prediction, called mVSG-VFP, which employs self-supervised learning and graphbased techniques. The proposed method realizes the failure prediction task by exploring information hidden in the time-series data recorded through the sensors embedded in the vehicle. mVSG-VFP includes two main components: a graph-based autoencoder that learns representations of normal data while considering the relationship between different sensors and a self-supervised component that maps temporally-adjacent data to similar representations. We propose a novel approach to define the notion of adjacency in vehicle temporal data. To evaluate mVSG-VFP, we apply it to a dataset comprised of vehicle sensor recordings to identify the abnormal data samples that signal a potential future failure. We performed a flurry of experiments to verify the accuracy of our model and demonstrate it outperforms state-of-the-art models in this task. Overall, the method is robust and intuitive, making it a useful tool for real-world applications.
更多查看译文
关键词
Self-Supervised Learning,Failure Prediction,Time-Series Anomaly Detection,Graph Neural Networks,Predictive Maintenance
AI 理解论文
溯源树
样例
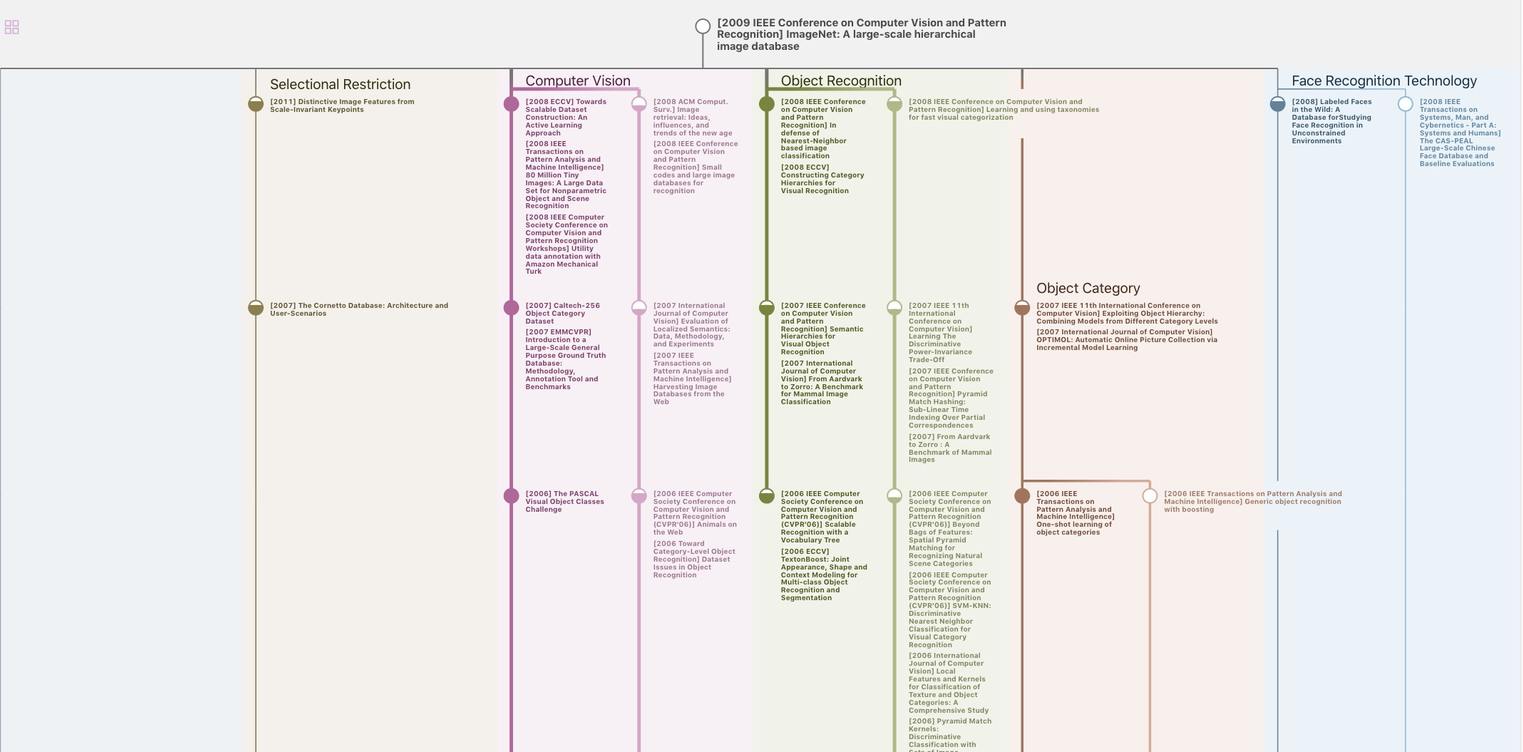
生成溯源树,研究论文发展脉络
Chat Paper
正在生成论文摘要