A Transductive Forest for Anomaly Detection with Few Labels
MACHINE LEARNING AND KNOWLEDGE DISCOVERY IN DATABASES: RESEARCH TRACK, ECML PKDD 2023, PT I(2023)
摘要
Extensive labeled training data for anomaly detection is enormously expensive and often unavailable in data-sensitive applications due to privacy constraints. We propose TransForest, a transductive forest for anomaly detection, in the semi-supervised setting where few labels are available. Guided by little label information, TransForest pushes classification boundaries toward sensitive areas where abnormal and normal points are located, increasing learning capacity. Empirically, TransForest is competitive with other unsupervised and semi-supervised representative detectors given a small number of labeled points. TransForest also offers a feature importance ranking consistent with the rankings provided by popular supervised forests on low-dimensional data sets. Our code is available at https://github.com/jzha968/transForest.
更多查看译文
AI 理解论文
溯源树
样例
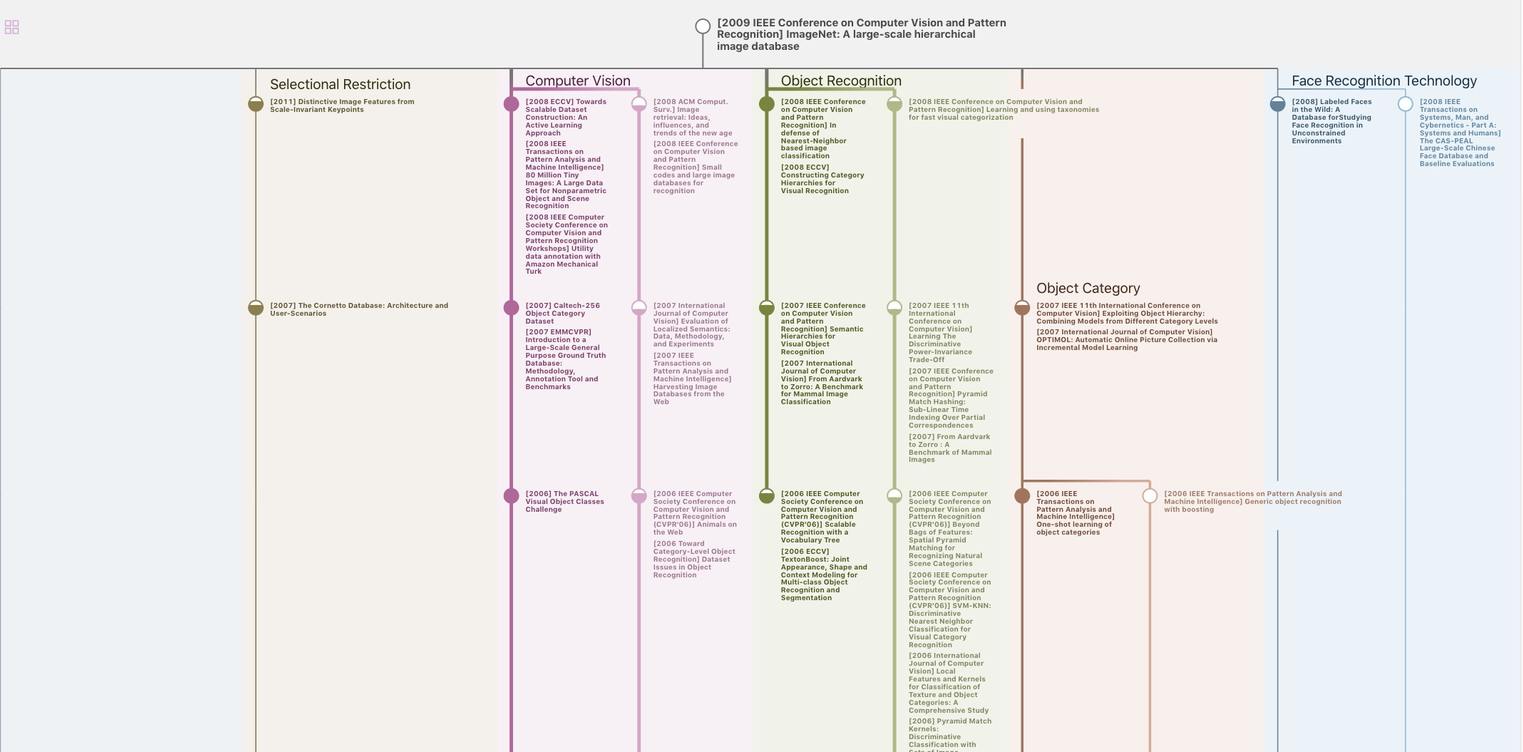
生成溯源树,研究论文发展脉络
Chat Paper
正在生成论文摘要