PCDF: A Parallel-Computing Distributed Framework for Sponsored Search Advertising Serving
MACHINE LEARNING AND KNOWLEDGE DISCOVERY IN DATABASES: APPLIED DATA SCIENCE AND DEMO TRACK, ECML PKDD 2023, PT VI(2023)
摘要
Traditional online advertising systems for sponsored search follow a cascade paradigm with retrieval, pre-ranking, ranking, respectively. Constrained by strict requirements on online inference efficiency, it tend to be difficult to deploy useful but computationally intensive modules in the ranking stage. Moreover, ranking models currently used in the industry assume the user click only relies on the advertisements itself, which results in the ranking stage overlooking the impact of organic search results on the predicted advertisements (ads). In this work, we propose a novel framework PCDF (Parallel-Computing Distributed Framework), allowing to split the computation cost into three parts and to deploy them in the pre-module in parallel with the retrieval stage, the middle-module for ranking ads, and the post-module for re-ranking ads with external items. Our PCDF effectively reduces the overall inference latency compared with the classic framework. The whole module is end-to-end offline training and adapt for the online learning paradigm. To our knowledge, we are the first to propose an end-to-end solution for online training and deployment on complex CTR models from the system framework side.
更多查看译文
关键词
Parallel and Distributed Mining,Advertising System,Online Serving
AI 理解论文
溯源树
样例
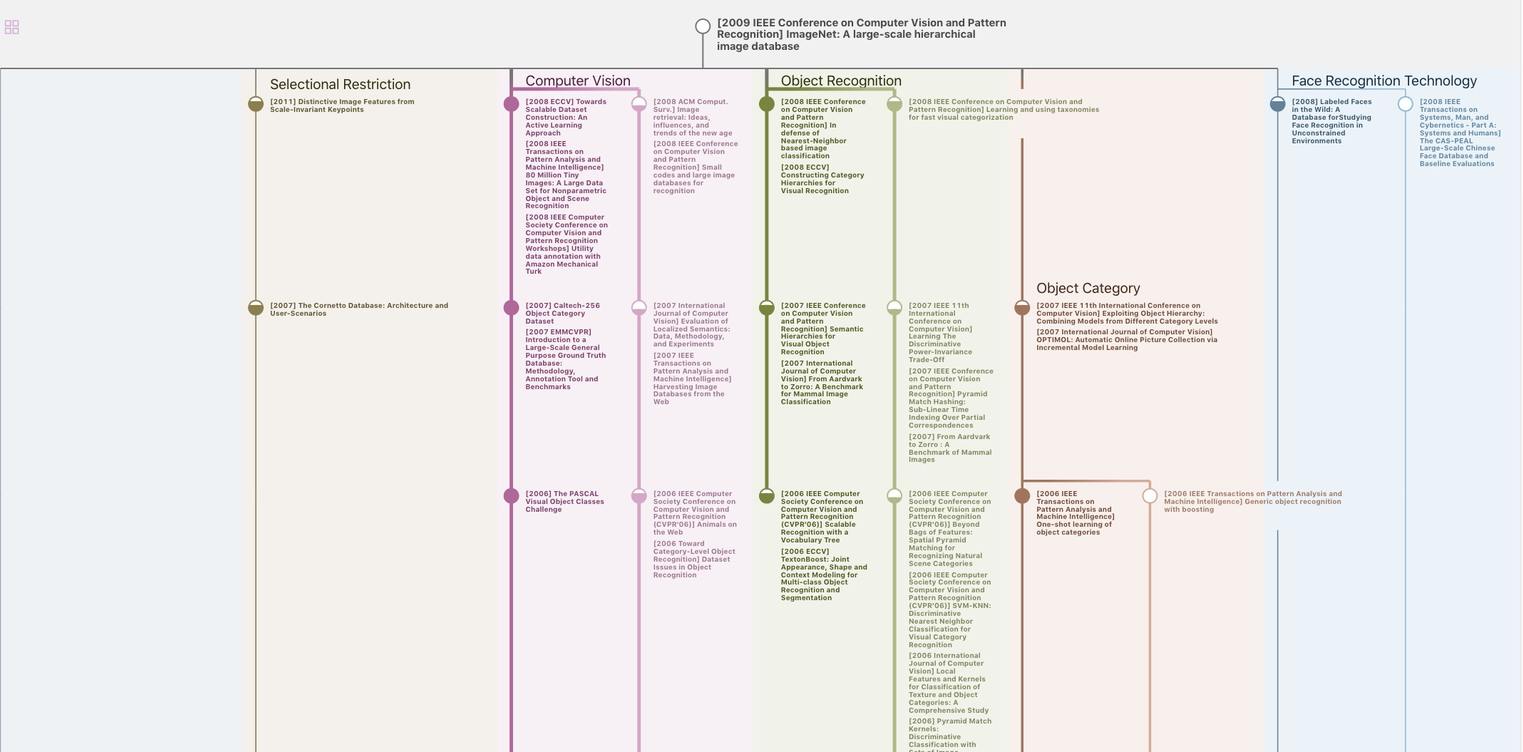
生成溯源树,研究论文发展脉络
Chat Paper
正在生成论文摘要