Is My Neural Net Driven by the MDL Principle?
MACHINE LEARNING AND KNOWLEDGE DISCOVERY IN DATABASES: RESEARCH TRACK, ECML PKDD 2023, PT II(2023)
摘要
The Minimum Description Length principle (MDL) is a formalization of Occam's razor for model selection, which states that a good model is one that can losslessly compress the data while including the cost of describing the model itself. While MDL can naturally express the behavior of certain models such as autoencoders (that inherently compress data) most representation learning techniques do not rely on such models. Instead, they learn representations by training on general or, for self-supervised learning, pretext tasks. In this paper, we propose a new formulation of the MDL principle that relies on the concept of signal and noise, which are implicitly defined by the learning task at hand. Additionally, we introduce ways to empirically measure the complexity of the learned representations by analyzing the spectra of the point Jacobians. Under certain assumptions, we show that the singular values of the point Jacobians of Neural Networks driven by the MDL principle should follow either a power law or a lognormal distribution. Finally, we conduct experiments to evaluate the behavior of the proposed measure applied to deep neural networks on different datasets, with respect to several types of noise. We observe that the experimental spectral distribution is in agreement with the spectral distribution predicted by our MDL principle, which suggests that neural networks trained with gradient descent on noisy data implicitly abide the MDL principle.
更多查看译文
关键词
Neural Networks,MDL,Signal-Noise,Point Jacobians
AI 理解论文
溯源树
样例
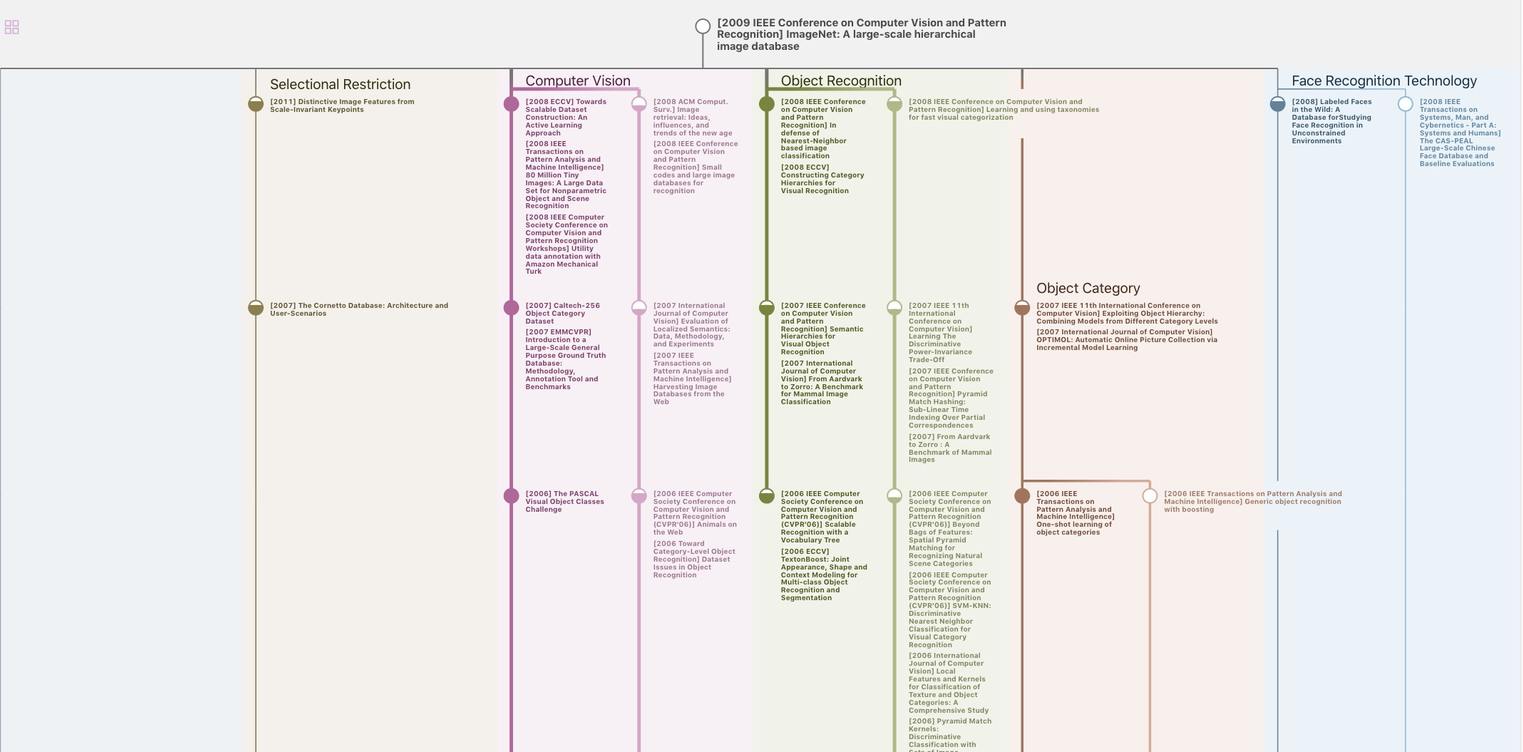
生成溯源树,研究论文发展脉络
Chat Paper
正在生成论文摘要