Conformal Prediction and Uncertainty Wrapper: What Statistical Guarantees Can You Get for Uncertainty Quantification in Machine Learning?
COMPUTER SAFETY, RELIABILITY, AND SECURITY, SAFECOMP 2023 WORKSHOPS(2023)
摘要
With the increasing use of Artificial Intelligence (AI), the dependability of AI-based software components becomes a key factor, especially in the context of safety-critical applications. However, as current AI-based models are data-driven, there is an inherent uncertainty associated with their outcomes. Some in-model uncertainty quantification (UQ) approaches integrate techniques during model construction to obtain information about the uncertainties during inference, e.g., deep ensembles, but do not provide probabilistic guarantees. Two model-agnostic UQ approaches that both provide probabilistic guarantees are conformal prediction (CP), and uncertainty wrappers (UWs). Yet, they differentiate in the type of quantifications they provide. CP provides sets or regions containing the intended outcome with a given probability, UWs provide uncertainty estimates for point predictions. To investigate how well they perform compared to each other and a baseline in-model UQ approach, we provide a side-by-side comparison based on their key characteristics. Additionally, we introduce an approach combining UWs with CP. The UQ approaches are benchmarked with respect to point uncertainty estimates, and to prediction sets. Regarding point uncertainty estimates, the UW shows the best reliability as CP was not designed for this task. For the task of providing prediction sets, the combined approach of UWs with CP outperforms the other approaches with respect to adaptivity and conditional coverage.
更多查看译文
关键词
Dependable AI,Benchmarking Study,Traffic Sign Recognition,Automated Driving,Model Agnostic Uncertainty Estimation,Reliability
AI 理解论文
溯源树
样例
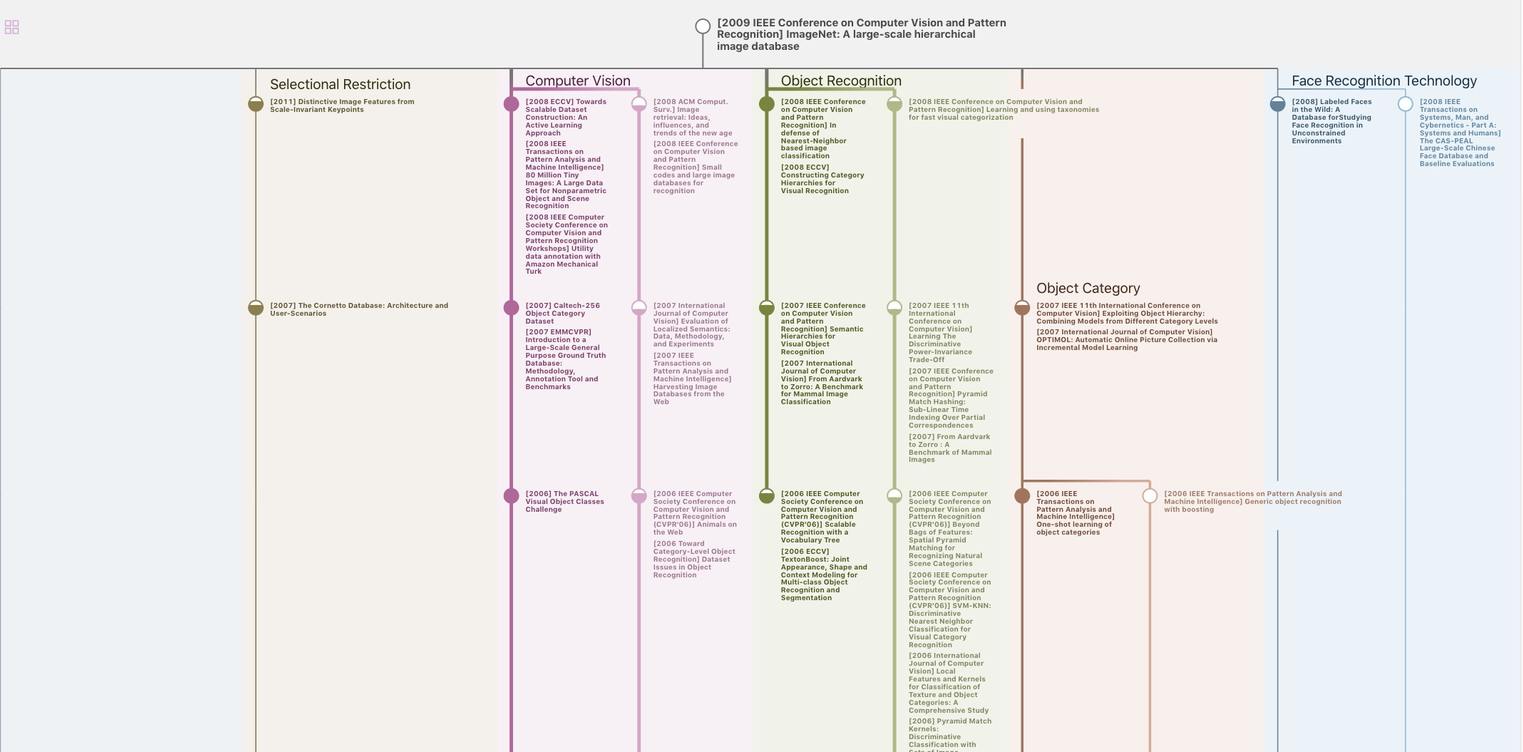
生成溯源树,研究论文发展脉络
Chat Paper
正在生成论文摘要