Towards Low-Cost Learning-based Camera ISP via Unrolled Optimization.
CRV(2023)
摘要
Recently, learning-based image signal processor (ISP) pipelines modeled using convolutional neural networks (CNNs) have been able to provide higher quality images over traditional model-based ISPs at the expense of significant memory, energy, and computation overhead. We propose an unrolled optimization network that models the ISP pipeline with considerably lower number of parameters and computation overhead. The unrolled optimization solves the image reconstruction problem of the ISP by leveraging both model-based and learning-based methods. In the proposed ISP model, the image formation operators namely, blur kernels and sensor sampling functions are formulated with learnable parameters such that the physical constraints are respected during conventional training. A CNN that is shared across the iterations of the unrolled model plays the role of the prior and performs denoising. An efficient tone mapper network is also utilized to further improve the quality of the resulting images. The entire pipeline is then trained in an end-to-end fashion using perceptual loss. The proposed ISP has over 34x fewer parameters in comparison to the state-of-the art deep ISPs.
更多查看译文
关键词
ISP, Unrolled Optimization, Image Degradation
AI 理解论文
溯源树
样例
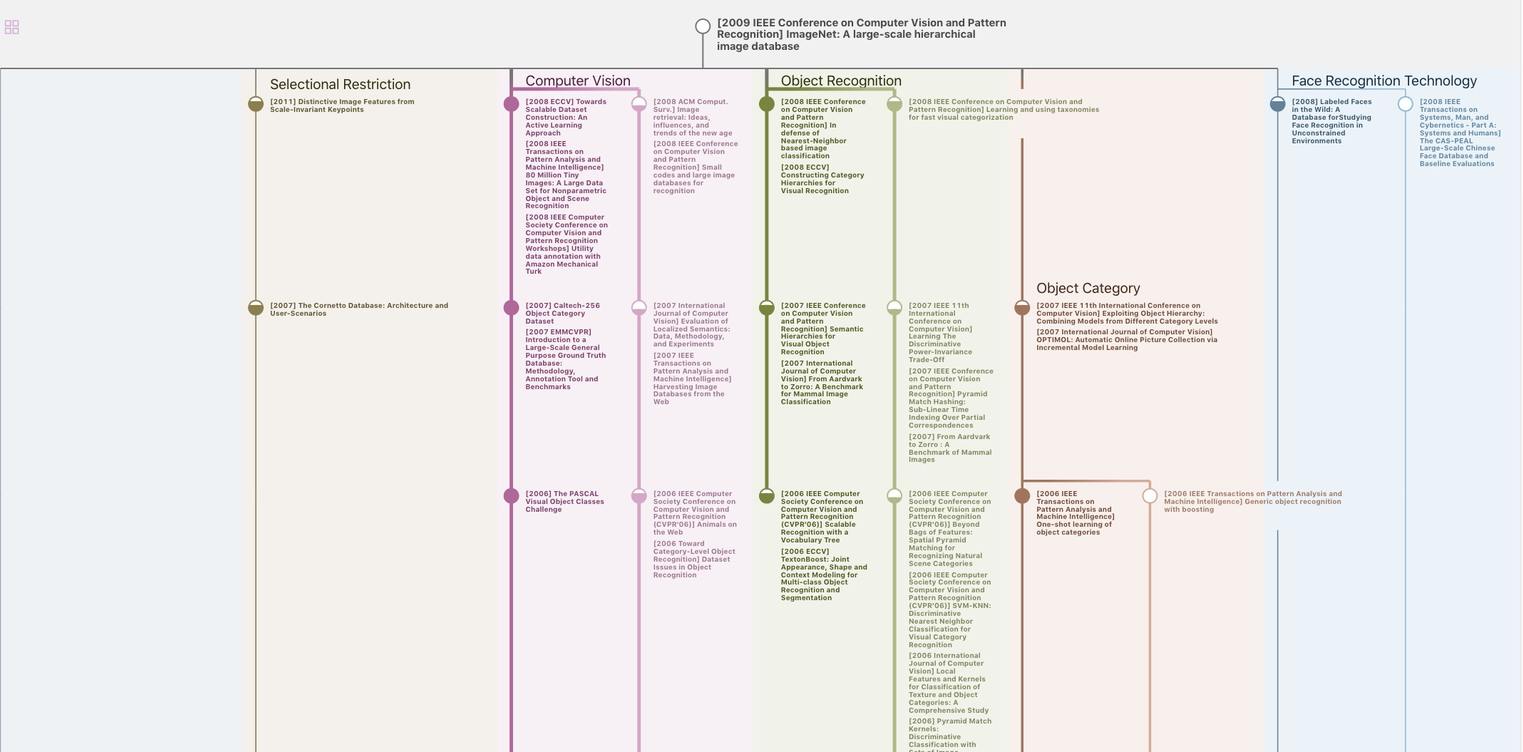
生成溯源树,研究论文发展脉络
Chat Paper
正在生成论文摘要