A Novel Smartphone-Based Human Activity Recognition Approach using Convolutional Autoencoder Long Short-Term Memory Network.
IRI(2023)
摘要
In smart and intelligent health care, smartphone sensor-based automatic recognition of human activities has evolved as an emerging field of research. In many application domains, deep learning (DL) strategies are more effective than conventional machine learning (ML) models, and human activity recognition (HAR) is no exception. In this paper, we propose a novel framework (CAEL-HAR), that combines CNN, Autoencoder and LSTM architectures for efficient smartphone-based HAR operation. There is a natural synergy between the modeling abilities of LSTMs, autoencoders, and CNNs. While AEs are used for dimensionality reduction and CNNs are the best at automating feature extraction, LSTMs excel at modeling time series. Taking advantage of their complementarity, the proposed methodology combines CNNs, AEs, and LSTMs into a single architecture. We evaluated the proposed architecture using the UCI, WISDM public benchmark datasets. The simulation and experimental results certify the merits of the proposed method and indicate that it outperforms computing time, F1-score, precision, accuracy, and recall in comparison to the current state-of-the-art methods.
更多查看译文
关键词
Human activity recognition (HAR), deep Learning, CNN, smartphone sensors, autoencoder, LSTM
AI 理解论文
溯源树
样例
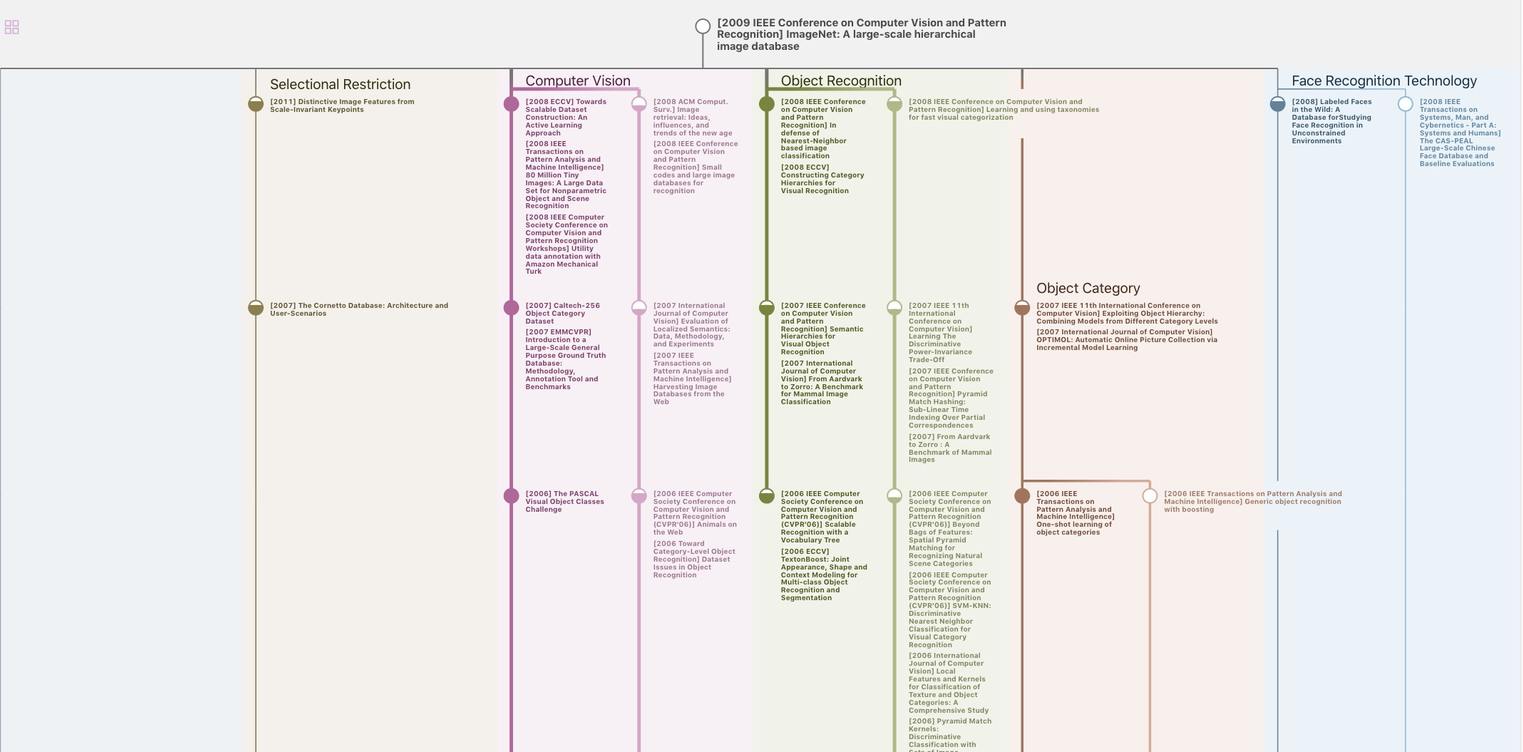
生成溯源树,研究论文发展脉络
Chat Paper
正在生成论文摘要