Ballot Tabulation Using Deep Learning.
IRI(2023)
摘要
Currently deployed election systems that scan and process hand-marked ballots are not sophisticated enough to handle marks insufficiently filled in (e.g., partially filled-in), improper marks (e.g., using check marks or crosses instead of filling in bubbles), or marks outside of bubbles, other than setting a threshold to detect whether the pixels inside bubbles are dark and dense enough to be counted as a vote. The current works along this line are still largely limited by their degree of automation and require substantial manpower for annotation and adjudication. In this study, we propose a highly automated deep learning (DL) mark segmentation model-based ballot tabulation assistant able to accurately identify legitimate ballot marks. For comparison purposes, a highly customized traditional computer vision (T-CV) mark segmentation-based method has also been developed to compare with the DL-based tabulator, with a detailed discussion included. Our experiments conducted on two real election datasets achieved the highest accuracy of 99.984% on ballot tabulation. In order to further enhance our DL model's capability of detecting the marks that are underrepresented in training datasets, e.g., insufficiently or improperly filled marks, we propose a Siamese network architecture that enables our DL model to exploit the contrasting features between a hand-marked ballot image and its corresponding blank template image to detect marks. Without the need for extra data collection, by incorporating this novel network architecture, our DL model-based tabulation method not only achieved a higher accuracy score but also substantially reduced the overall false negative rate.
更多查看译文
关键词
Deep learning, Ballot tabulation, Computer vision
AI 理解论文
溯源树
样例
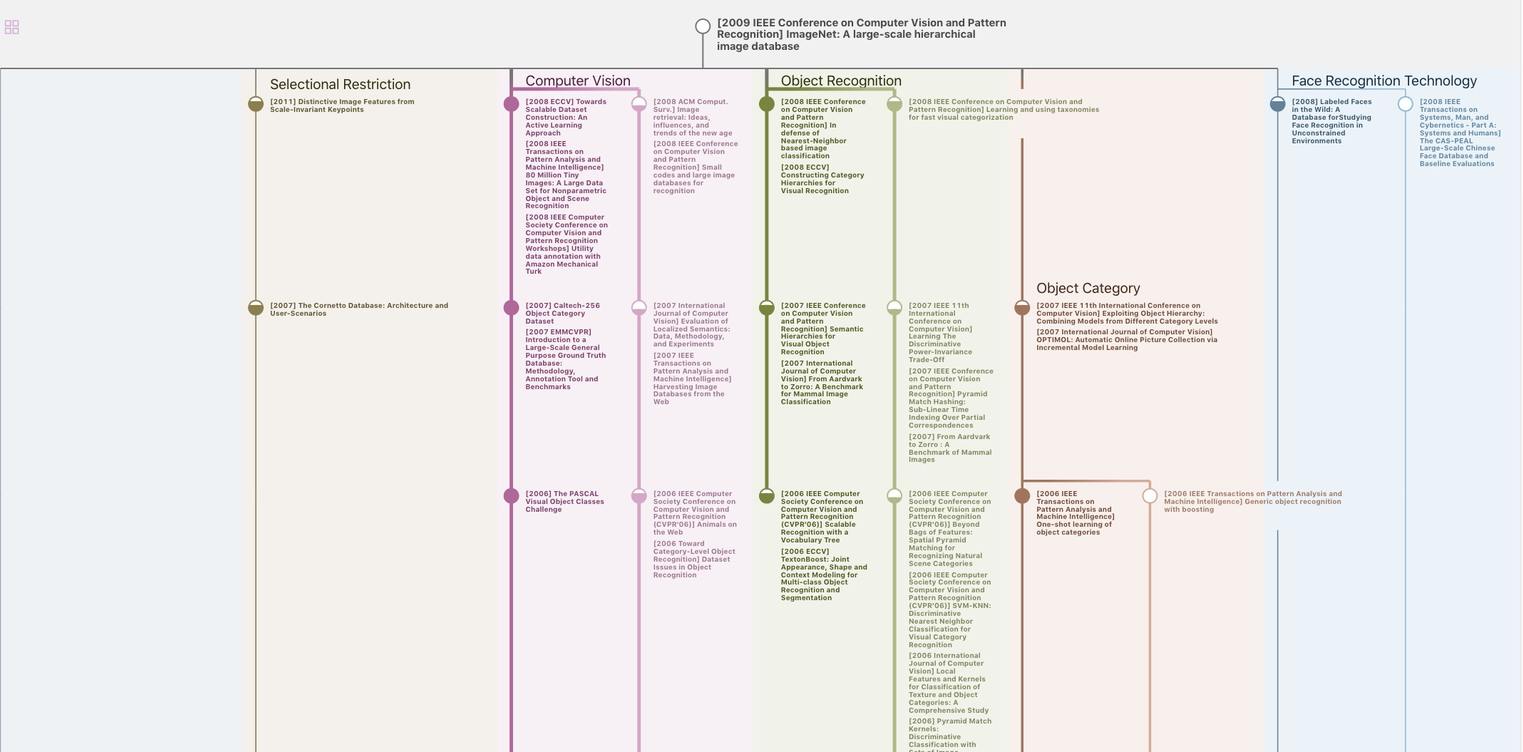
生成溯源树,研究论文发展脉络
Chat Paper
正在生成论文摘要