Improving the Reusability of Pre-trained Language Models in Real-world Applications.
IRI(2023)
摘要
The reusability of state-of-the-art Pre-trained Language Models (PLMs) is often limited by their generalization problem, where their performance drastically decreases when evaluated on examples that differ from the training dataset, known as Out-of-Distribution (OOD)/unseen examples. This limitation arises from PLMs' reliance on spurious correlations, which work well for frequent example types but not for general examples. To address this issue, we propose a training approach called Mask-tuning, which integrates Masked Language Modeling (MLM) training objectives into the fine-tuning process to enhance PLMs' generalization. Comprehensive experiments demonstrate that Mask-tuning surpasses current state-of-the-art techniques and enhances PLMs' generalization on OOD datasets while improving their performance on in-distribution datasets. The findings suggest that Mask-tuning improves the reusability of PLMs on unseen data, making them more practical and effective for real-world applications.
更多查看译文
关键词
NLP applications, Pre-trained language models' reusability, Transfer learning, Integrated training
AI 理解论文
溯源树
样例
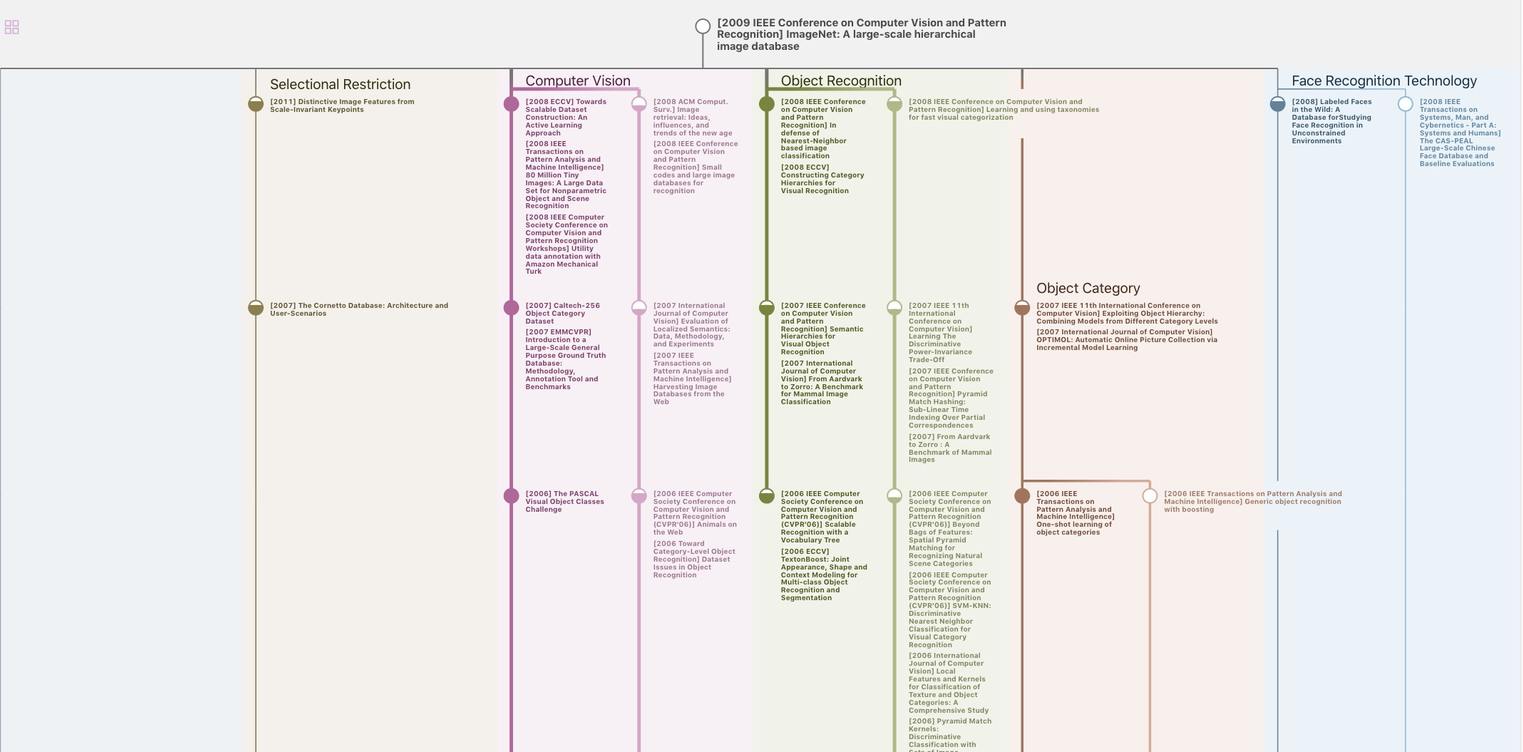
生成溯源树,研究论文发展脉络
Chat Paper
正在生成论文摘要