From Bounded to Unbounded: Privacy Amplification via Shuffling with Dummies.
CSF(2023)
摘要
In recent years, the shuffling model has been garnering attention in the realm of differential privacy (DP). This study focuses on the fact that the shuffling model follows bounded DP rather than unbounded DP. This characteristic causes a privacy issue in which participation itself is not protected. To address this issue, we propose a framework, called unbounded shuffling, which follows unbounded DP in addition to bounded DP under the trust assumption of the shuffling model. The main difference from the conventional shuffling model is the inclusion of dummies, which some users add to pose that perturbed records are sent by other users. We also analyze the privacy and utility of our proposed framework. The analysis shows that our framework achieves almost the same utility and privacy as that of the traditional shuffling model while guaranteeing unbounded DP. Additionally, we apply the technique of individual privacy accounting, which is built solely on unbounded DP, to stochastic gradient descent (SGD) using our framework. This approach approximately halves the value of
$\varepsilon$
of a baseline.
更多查看译文
关键词
differential privacy, shuffling model
AI 理解论文
溯源树
样例
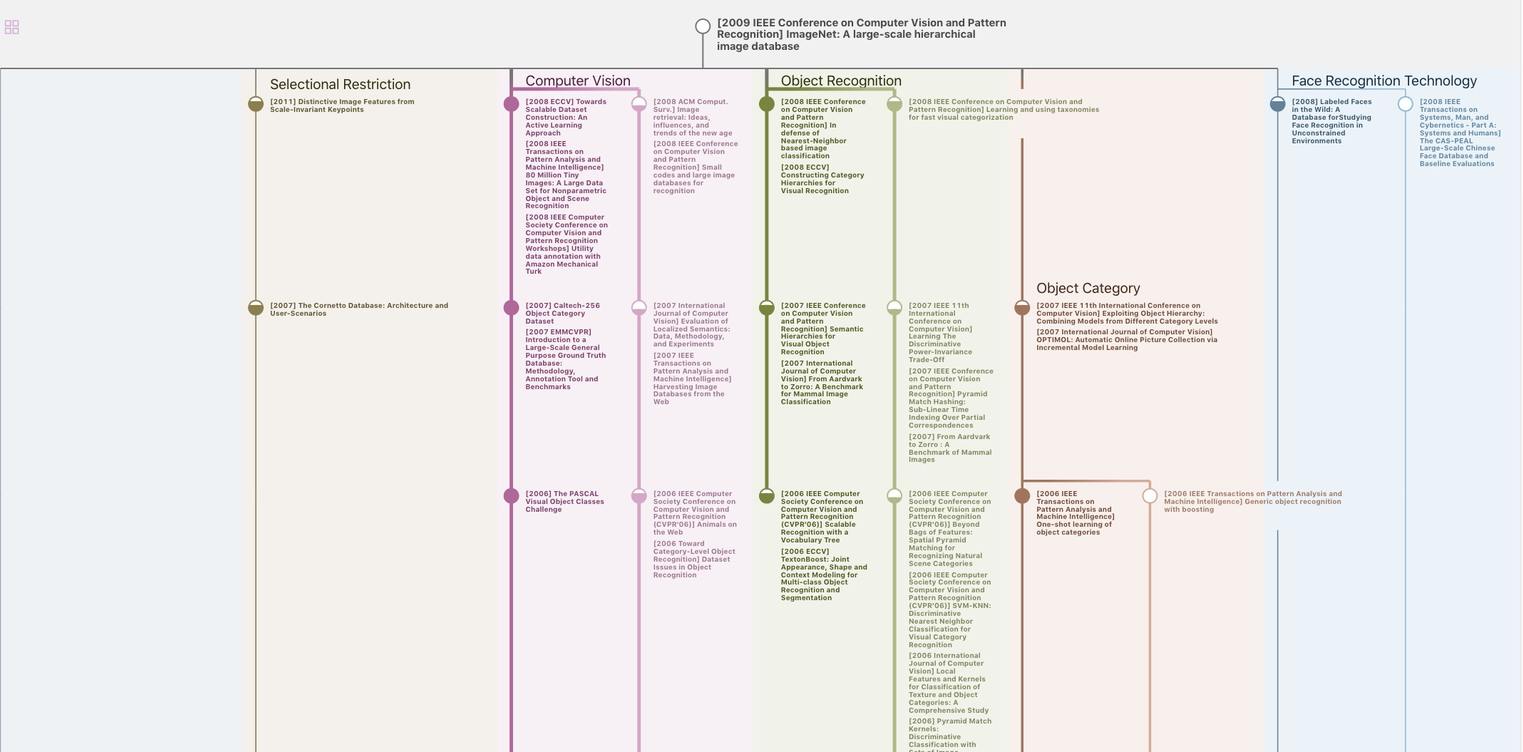
生成溯源树,研究论文发展脉络
Chat Paper
正在生成论文摘要