One-class Classification Using Autoencoder Feature Residuals for Improved IoT Network Intrusion Detection.
ICCCN(2023)
摘要
Networks are rapidly evolving to include more Internet of Things devices as we grow to rely on them for smart home services, health infrastructure, and industrial development. Along with this proliferation, these networks are often the target of cyberattacks that seek to take advantage of their low processing power and the complexity that is involved in protecting heterogeneous networks. We seek to improve our network intrusion detection capabilities for Internet of Things networks by developing methods of detection that utilize the power of deep learning and have the ability to detect zero-day attacks. In this work, we outline a novel feature generation process using autoencoder feature residuals that can be combined with one-class classifiers to effectively detect network attacks on Internet of Things networks using no attack data during the training process. Moreover, we show that our novel feature sets are able to outperform using an original feature set leading to a reduction of typical model hyperparameter tuning activities.
更多查看译文
关键词
autoencoder,network intrusion detection,internet of things,one-class classification
AI 理解论文
溯源树
样例
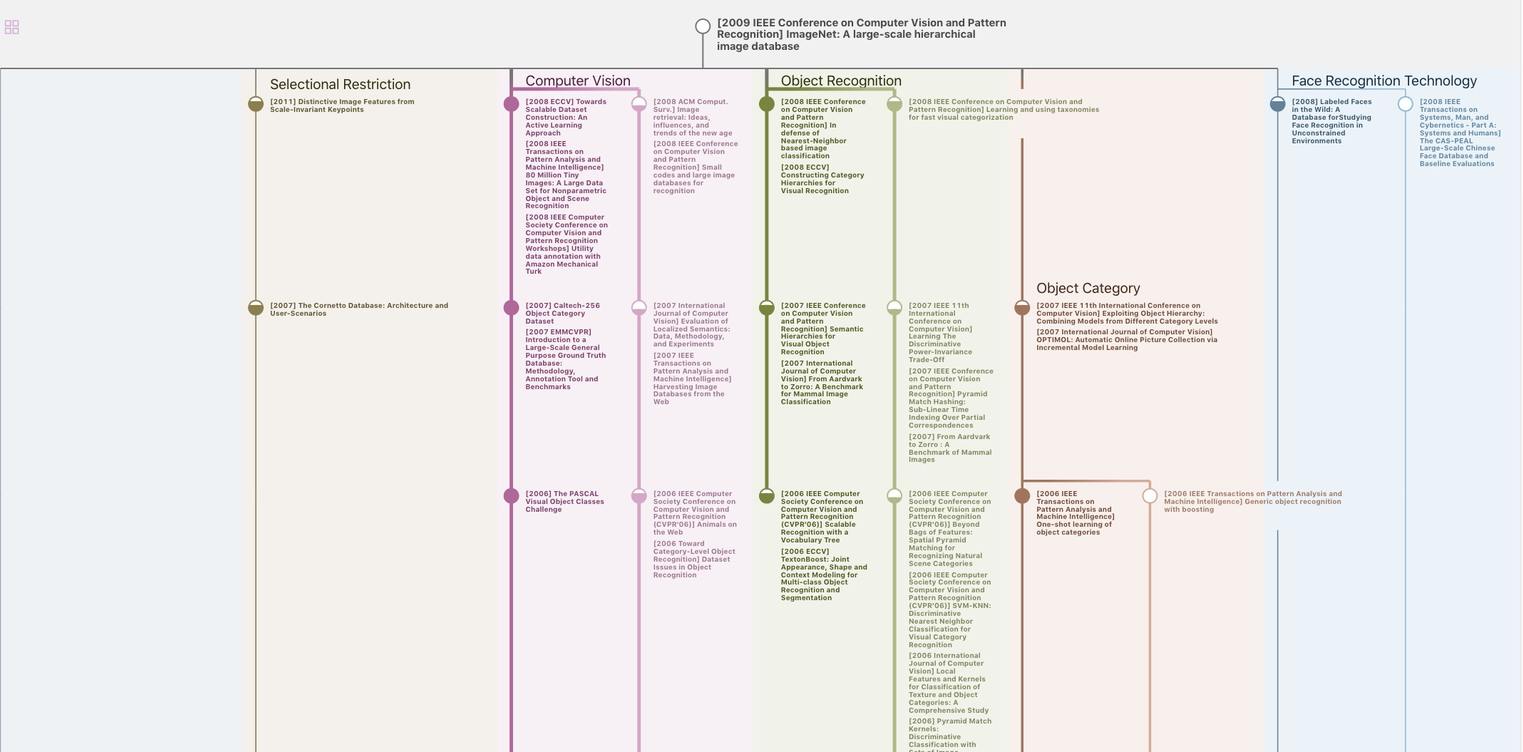
生成溯源树,研究论文发展脉络
Chat Paper
正在生成论文摘要