Fourier Descriptor Loss and Polar Coordinate Transformation for Pericardium Segmentation
COMPUTER ANALYSIS OF IMAGES AND PATTERNS, CAIP 2023, PT II(2023)
摘要
Epicardial adipose tissue (EAT) located inside the pericardium is a marker for increased risk of many cardiovascular diseases. Automatic segmentation methods for pericardium or EAT are necessary to support the otherwise extremely time-consuming manual delineation in CT scans. Powerful deep learning-based methods have been applied to such segmentation tasks. However, existing methods primarily rely on region-based or distribution-based loss functions, such as Dice loss or cross-entropy loss. Unfortunately, these approaches overlook the informative anatomical priors, such as the shape of the pericardium. In light of this, our work introduces an innovative approach by proposing and comparing a shape-based loss that leverages anatomical priors derived from Fourier descriptors. By incorporating the anatomical prior, we aim to enhance the accuracy and effectiveness of pericardium or EAT segmentation. The Fourier descriptor loss can be used individually or as a regularizer with region-based losses such as the Dice loss for higher accuracy and faster convergence. As a regularizer, the proposed loss obtains the highest mean intersection of union (96.76%), Dice similarity coefficient (98.20%), and sensitivity (98.55%) outperforming the Dice and cross-entropy loss. We show the effect of the Fourier descriptor loss with fewer and weighted descriptors. The results show the efficiency and flexibility of the Fourier descriptor loss and its potential for segmenting shapes.
更多查看译文
关键词
Fourier descriptors,segmentation neural networks,pericardium segmentation,shape-based loss functions
AI 理解论文
溯源树
样例
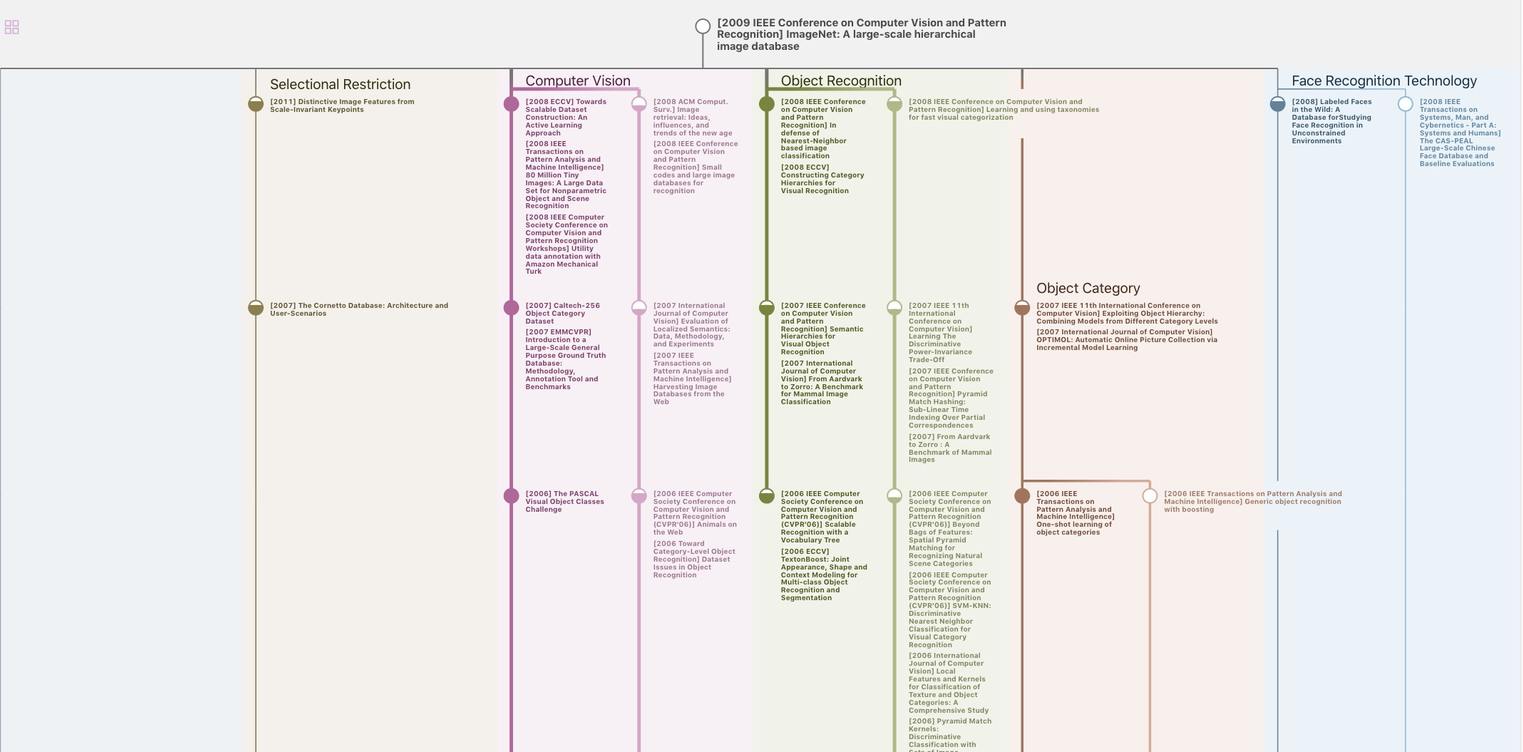
生成溯源树,研究论文发展脉络
Chat Paper
正在生成论文摘要