Iterative Learning Model Predictive Control for Thermal Management of Urban Air Mobility Vehicles*
CCTA(2023)
摘要
With increasing interests in Urban Air Mobility (UAM) vehicles, control techniques tailored to UAM must be developed to meet the demands of these electrified vehicles. By utilizing the point to point, repetitive nature of UAM vehicle flights, this paper uses a combination of iterative learning control (ILC) and model predictive control (MPC) to address a subset of the control challenges present within UAM vehicles. Due to the coupling between the mechanical, electrical, and thermal domains on these vehicles, the control of the powertrain and thermal management systems for UAM vehicles is challenging and can lead to constraint violations on component power levels or temperatures. As component temperatures may be governed by constraints rather than references, this paper presents a constraint tightening technique for MPC through an ILC update law. The constraints are iteratively tightening based on the constraint violations from the last iteration to encourage the MPC to choose trajectories which will prevent the true system from violating constraints. For a simulated case study, we demonstrate that a traditional MPC can result in thermal constraint violations for a UAM powertrain and thermal management system with fixed constraints; however, the iterative learning model predictive control approach converges to near zero constraint violations in a relatively short number of flights.
更多查看译文
关键词
component power levels,component temperatures,constraint tightening technique,control techniques,electrical, domains,electrified vehicles,fixed constraints,ILC update law,iteration,iterative learning model predictive control approach converges,mechanical domains,MPC,thermal constraint violations,thermal domains,thermal management system,UAM powertrain,UAM vehicle flights,UAM vehicles,urban air mobility vehicles,zero constraint violations
AI 理解论文
溯源树
样例
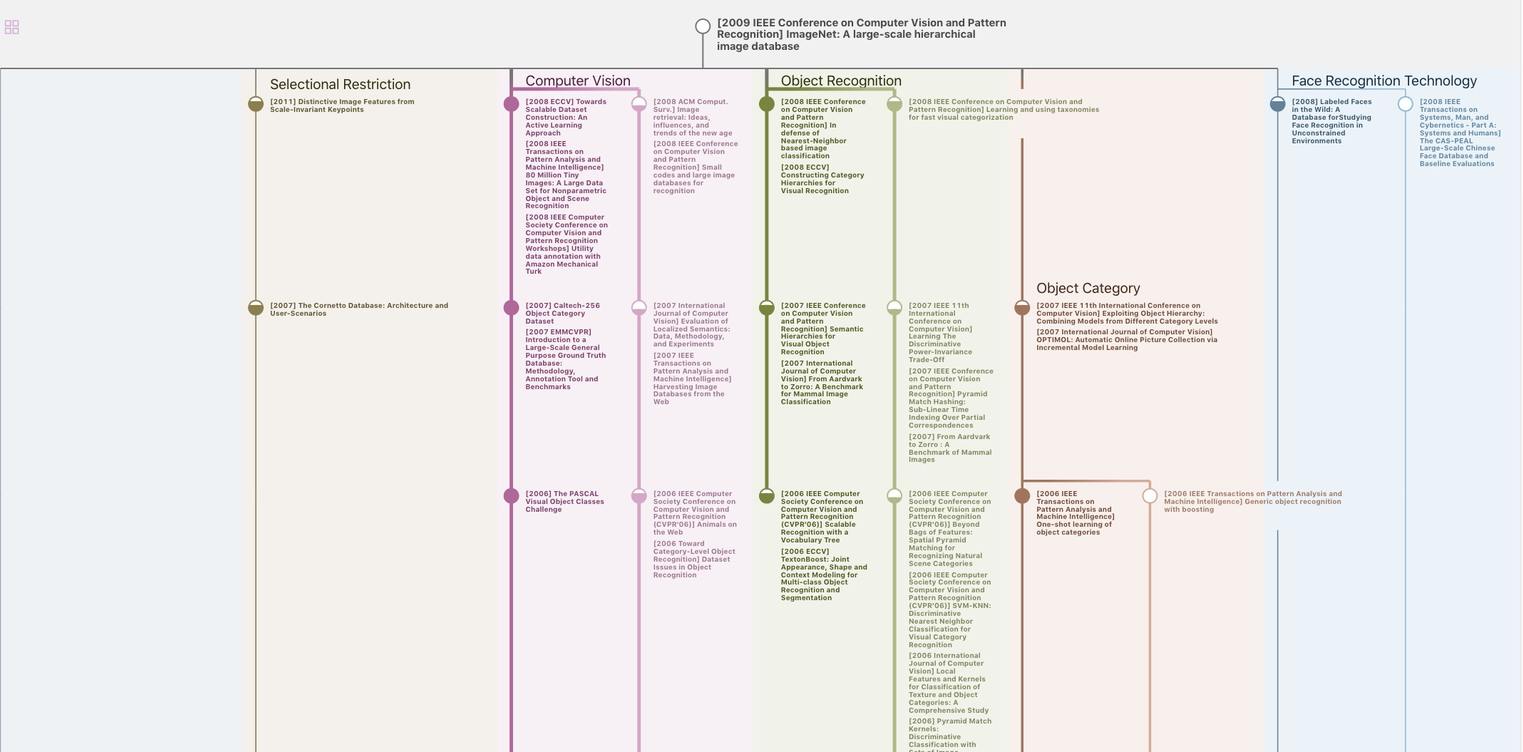
生成溯源树,研究论文发展脉络
Chat Paper
正在生成论文摘要