Federated Learning-Based Credit Card Fraud Detection: Performance Analysis with Sampling Methods and Deep Learning Algorithms
CSR(2023)
摘要
The exponential technological advancement is turning everyone towards an easy and efficient way of financial transactions. Consequently, the use of credit cards is rising sub-stantively, creating a more incredible opportunity for fraudsters which is an alarming concern nowadays since a fraudster may use several tools, techniques and tactics to make a fraudulent transaction. As a countermeasure, an effective fraud detection mechanism and highly sensitive data privacy preservation are imperative to detect fraudulent transactions. This paper proposes a Federated Learning (FL)-based fraud detection system since its key feature preserves the privacy of highly sensitive data, wherein the model could be trained without sharing the credit card data in the cloud. We contemplate three Deep Learning (DL) models: Convolutional Neural Network (CNN), Multi-Layer Perceptron (MLP), and Long Short-Term Memory (LSTM) regarding the FL approach. Subsequently, to overcome the data imbalance issue, four distinct sampling techniques are explored to inspect the impact on the traditional centralized and FL approaches. Finally, we further investigate and compare FL-based detection systems with diversified state-of-the-art models. Our experimental results demonstrate that the proposed method is superior compared with state-of-the-art methods and achieves high detection rate of 99.51%, 98.77% and 98.20% respectively for CNN, MLP and LSTM models.
更多查看译文
关键词
Credit card fraud,fraud detection system,federated learning,CNN,MLP,LSTM
AI 理解论文
溯源树
样例
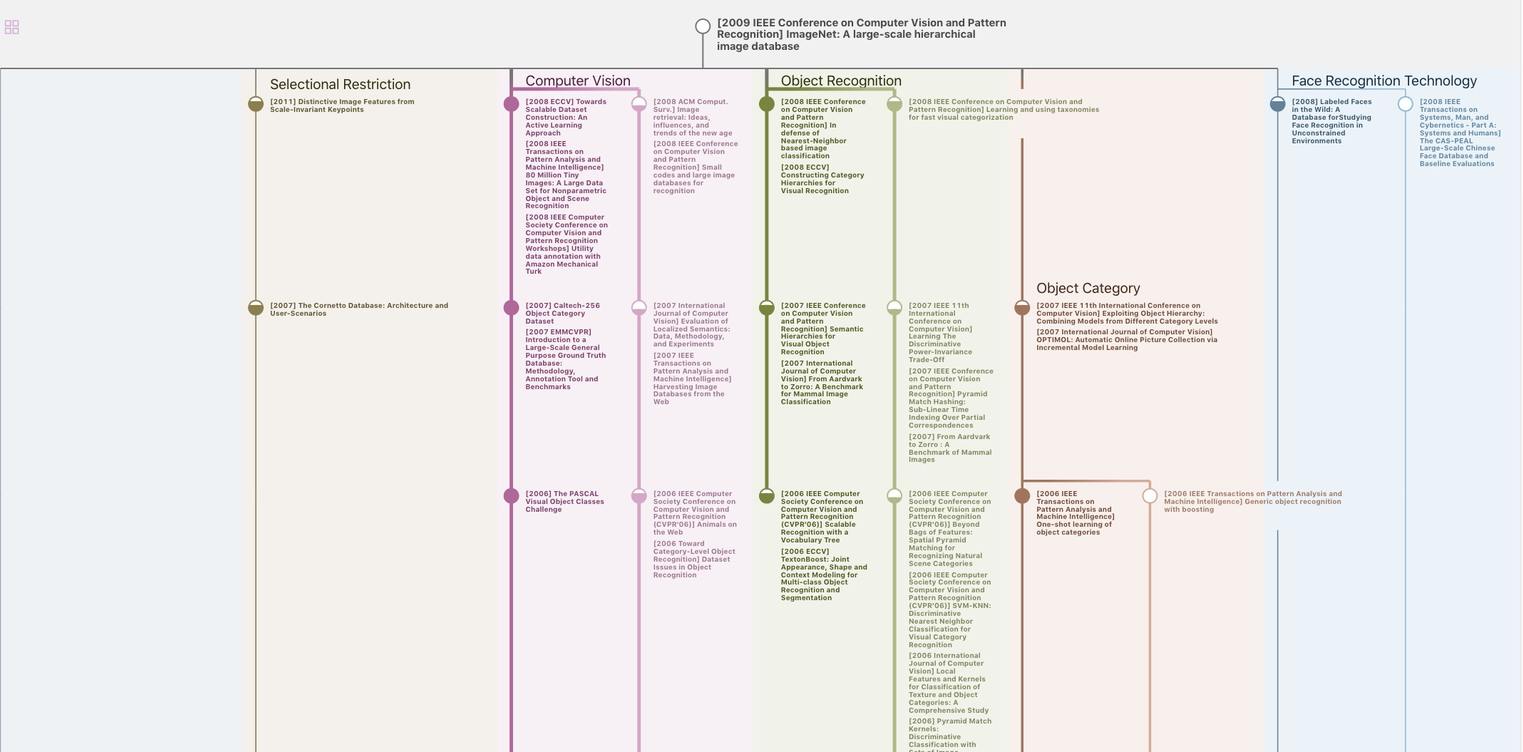
生成溯源树,研究论文发展脉络
Chat Paper
正在生成论文摘要