A New Hybrid CNN-LSTM for Wind Power Forecasting in Ethiopia.
HAIS(2023)
摘要
Renewable energies are currently experiencing promising growth as an alternative solution to minimize the emission of pollutant gases from the use of fossil fuels, which contribute to global warming. To integrate these renewable energies safely with the grid system and make the electric grid system more stable, it is vitally important to accurately forecast the amount of wind power generated at specific wind power generation sites and the timing of this generation. Deep learning approaches have shown good forecasting performance for complex and nonlinear problems, such as time series wind power data. However, further study is needed to optimize deep learning models by integrating multiple models with hyperparameter optimization, to attain optimal performance from these individual models. In this paper, we propose a hybrid CNN-LSTM model for wind power forecasting in Ethiopia. Bayesian optimization is applied to tune the hyperparameters of the individual learners, including 1D-CNN and LSTM models, before building the hybrid CNN-LSTM model. The proposed model is tested on three case study wind power datasets obtained from the Ethiopian Electric Power Corporation. According to the MAE, RMSE, and MAPE evaluation metrics, the hybrid model performs significantly better than benchmark models, including ANN, RNN, BiLSTM, CNN, and LSTM models, for all case study data.
更多查看译文
关键词
wind power forecasting,cnn-lstm
AI 理解论文
溯源树
样例
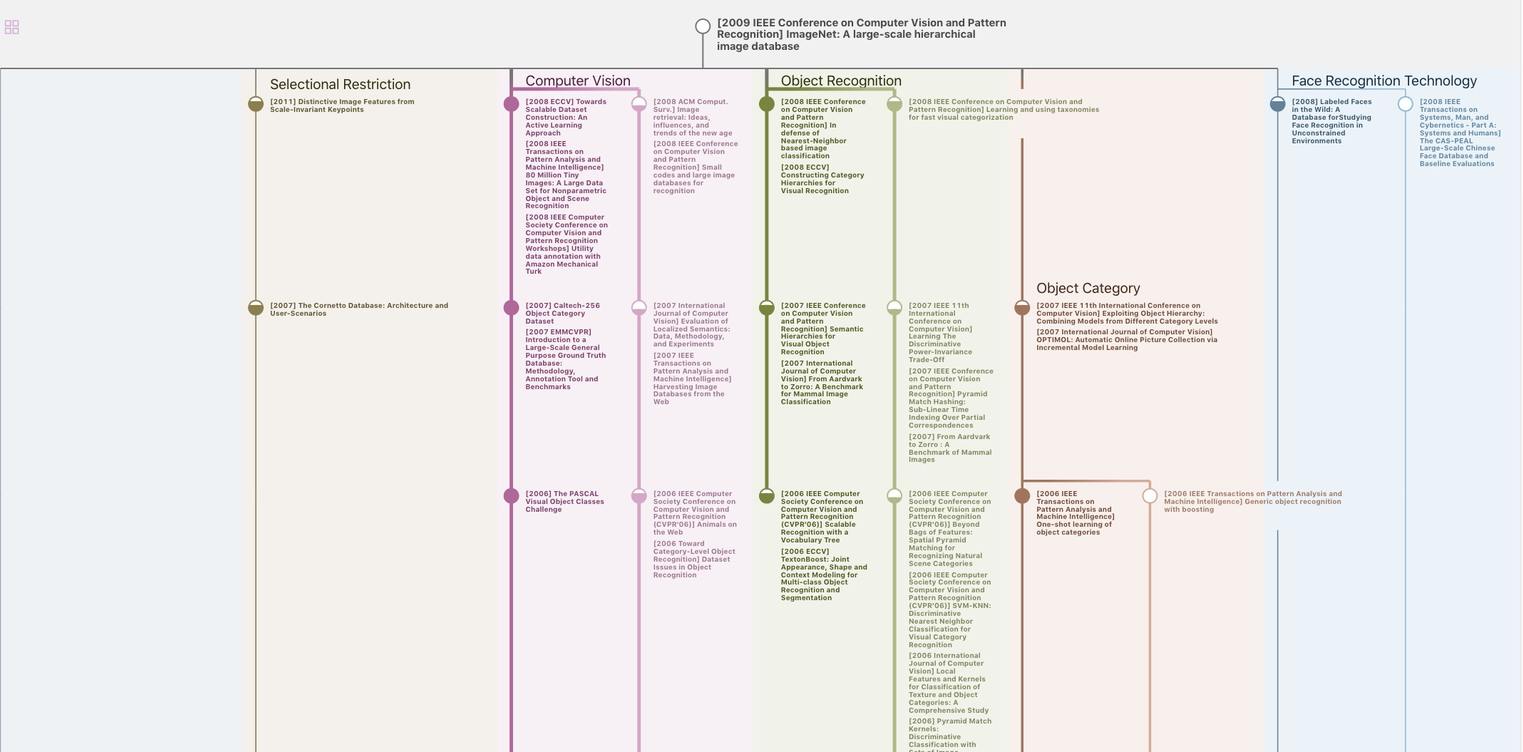
生成溯源树,研究论文发展脉络
Chat Paper
正在生成论文摘要