Optimal Motion Control using Neural Networks Applied to a Slider-Crank Mechanism.
CCTA(2023)
摘要
Given the rising performance targets and increasing system complexity of mechatronic systems, techniques which solve control tasks using optimization, so called Optimal Control Problems (OCPs), have become increasingly adopted. However, to achieve the targeted control performance, accurate models are key. Classical physical models are often used, however, deriving these is laborious and requires expert knowledge, which substantially limits the industrial uptake. In contrast to these physical models, machine learning approaches, specifically neural networks, have been shown to accurately model even complex dynamic effects with limited effort and expert knowledge. With this work, we present an approach to solve OCPs in which neural networks are used to model the system dynamics, thereby enabling high-performance control with reduced modeling effort, paving the way for increased industrial usage. The approach is validated on a simulated slider-crank mechanism to showcase its applicability to complex non-linear dynamic systems. Beyond this case it is expected to generalize to a wide variety of systems and control tasks.
更多查看译文
关键词
expert knowledge,high-performance control,increased industrial usage,machine learning approaches,mechatronic systems,neural networks,nonlinear dynamic systems,OCPs,optimal control problems,optimal motion control,optimization,reduced modeling effort,simulated slider-crank mechanism,system dynamics
AI 理解论文
溯源树
样例
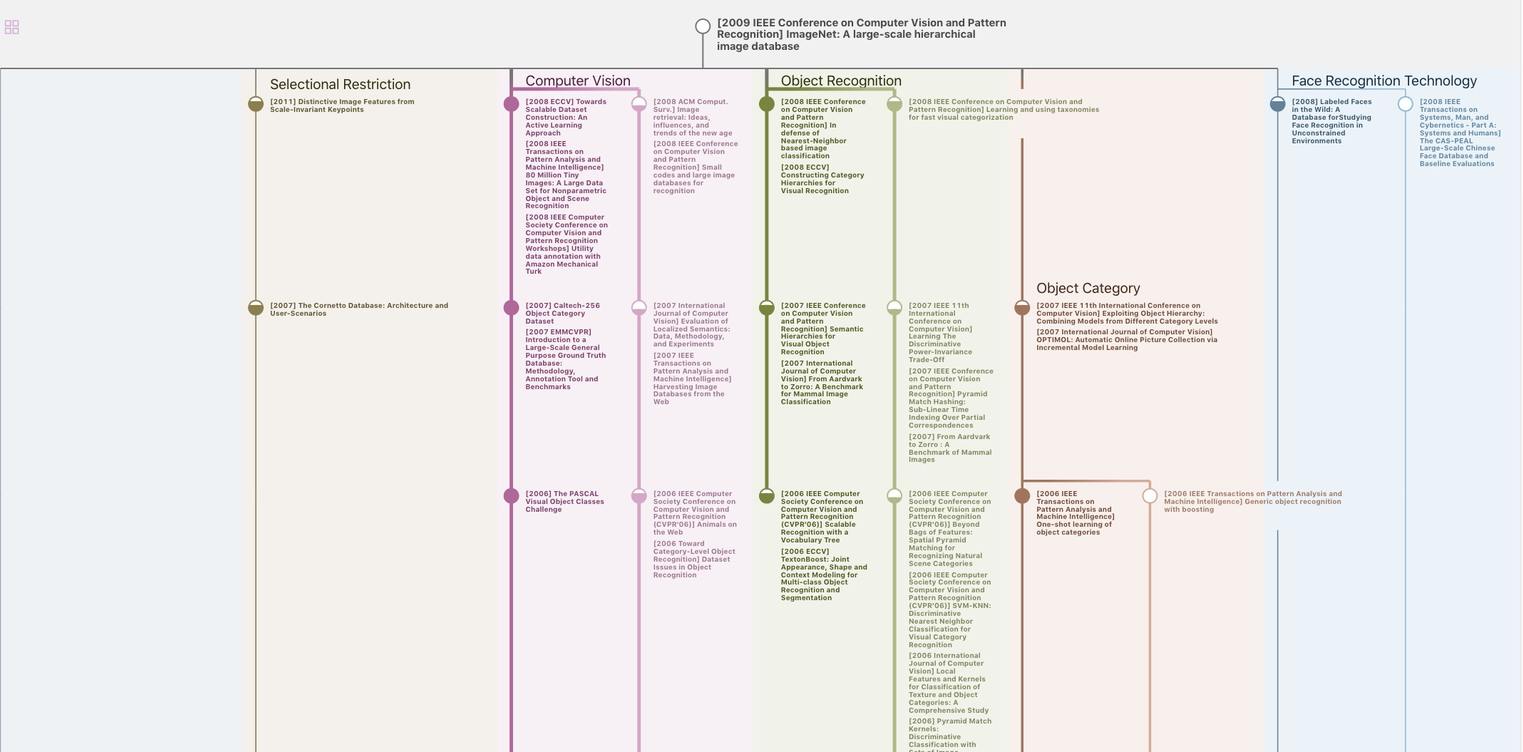
生成溯源树,研究论文发展脉络
Chat Paper
正在生成论文摘要