CELLFUSION: Multipath Vehicle-to-Cloud Video Streaming with Network Coding in the Wild
PROCEEDINGS OF THE 2023 ACM SIGCOMM 2023 CONFERENCE, SIGCOMM 2023(2023)
摘要
This paper presents CELLFUSION, a system designed for high-quality, real-time video streaming from vehicles to the cloud. It leverages an innovative blend of multipath QUIC transport and network coding. Surpassing the limitations of individual cellular carriers, CELLFUSION uses a unique last-mile overlay that integrates multiple cellular networks into a single, unified cloud connection. This integration is made possible through the use of in-vehicle Customer Premises Equipment (CPEs) and edge-cloud proxy servers. In order to effectively handle unstable cellular connections prone to intense burst losses and unexpected latency spikes as a vehicle moves, CELLFUSION introduces XNC. This innovative network coding-based transport solution enables efficient and resilient multipath transport. XNC aims to accomplish low latency, minimal traffic redundancy, and reduced computational complexity all at once. CELLFUSION is secure and transparent by nature and does not require modifications for vehicular apps connecting to it. We tested CELLFUSION on 100 self-driving vehicles for over six months with our cloud-native back-end running on 50 CDN PoPs. Through extensive road tests, we show that XNC reduced video packet delay by 71.53% at the 99th percentile versus 5G. At 30Mbps, CELLFUSION achieved 66.11% similar to 80.62% reduction in video stall ratio versus state-of-the-art multipath transport solutions with less than 10% traffic redundancy.
更多查看译文
关键词
Multipath QUIC,Network Coding,Video Streaming,Vehicular Networks,Self-driving
AI 理解论文
溯源树
样例
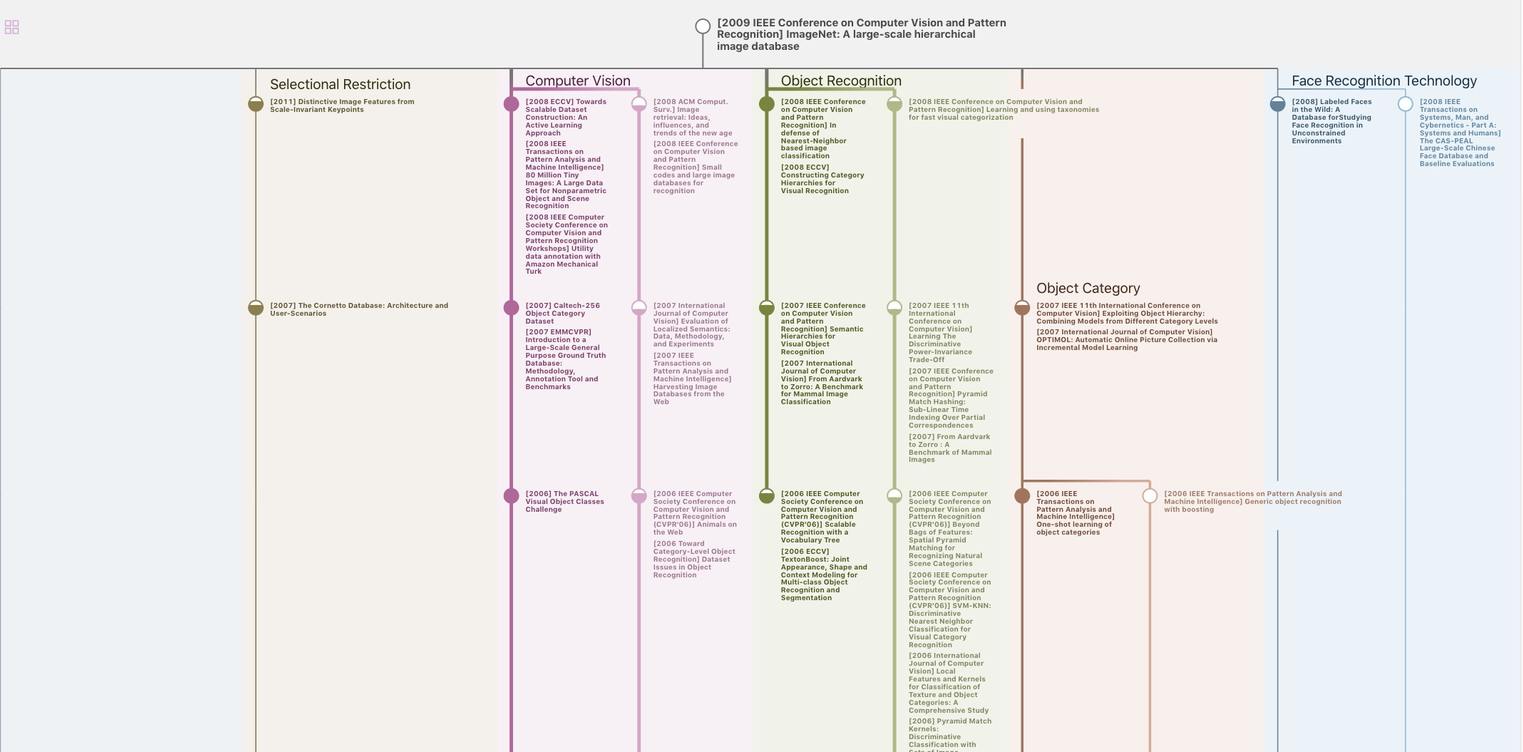
生成溯源树,研究论文发展脉络
Chat Paper
正在生成论文摘要