Intermittent-Aware Neural Network Pruning.
DAC(2023)
摘要
Deep neural network inference on energy harvesting tiny devices has emerged as a solution for sustainable edge intelligence. However, compact models optimized for continuously-powered systems may become suboptimal when deployed on intermittently-powered systems. This paper presents the pruning criterion, pruning strategy, and prototype implementation of iPrune, the first framework which introduces intermittency into neural network pruning to produce compact models adaptable to intermittent systems. The pruned models are deployed and evaluated on a Texas Instruments device with various power strengths and TinyML applications. Compared to an energy-aware pruning framework, iPrune can speed up intermittent inference by 1.1 to 2 times while achieving comparable model accuracy.
更多查看译文
关键词
Neural network pruning,deep learning,intermittent computing,battery-less devices
AI 理解论文
溯源树
样例
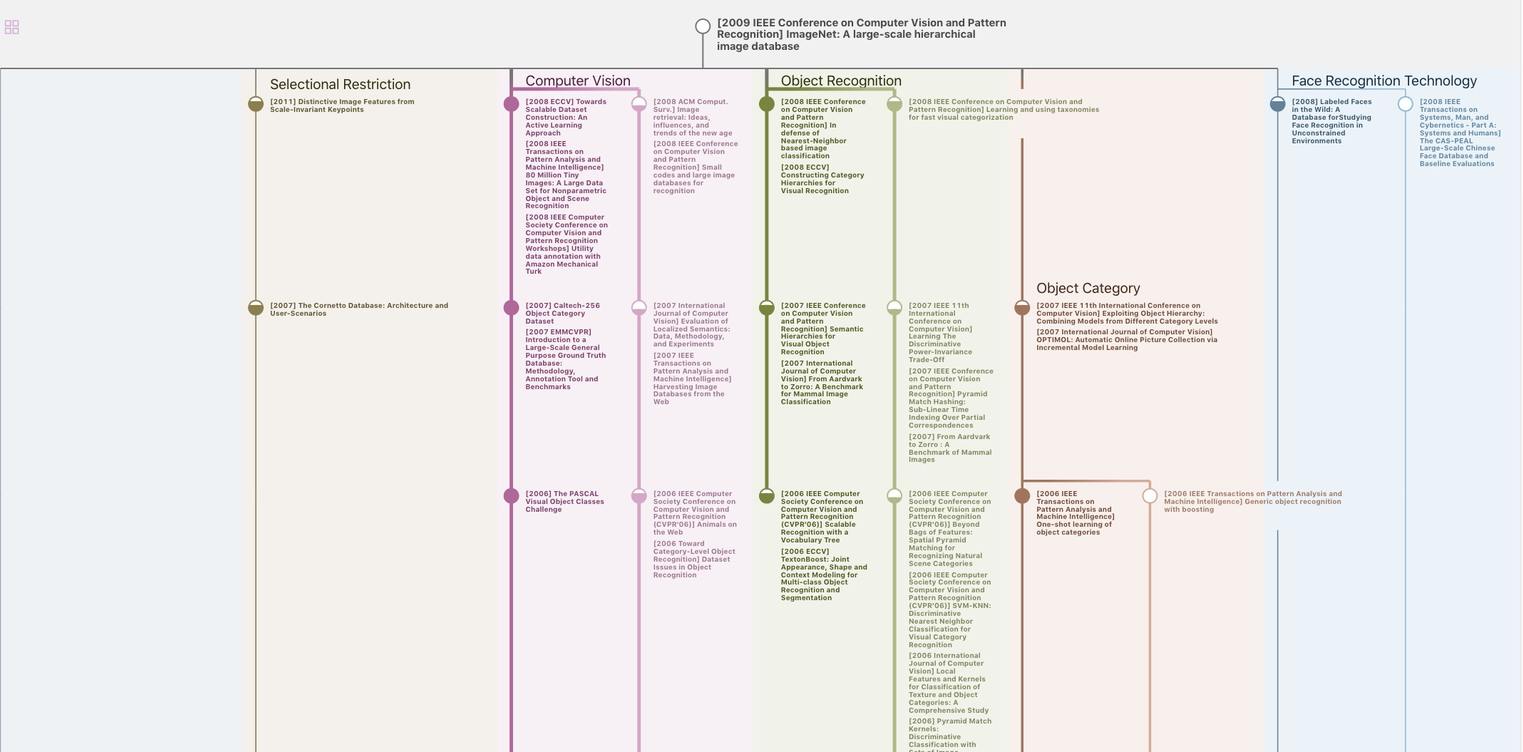
生成溯源树,研究论文发展脉络
Chat Paper
正在生成论文摘要