COSA:Co-Operative Systolic Arrays for Multi-head Attention Mechanism in Neural Network using Hybrid Data Reuse and Fusion Methodologies
DAC(2023)
摘要
Attention mechanism acceleration is becoming increasingly vital to achieve superior performance in deep learning tasks. Existing accelerators are commonly devised dedicatedly by exploring the potential sparsity in neural network (NN) models, which suffer from complicated training, tuning processes, and accuracy degradation. By systematically analyzing the inherent dataflow characteristics of attention mechanism, we propose the Co-Operative Systolic Array (COSA) to pursue higher computational efficiency for its acceleration. In COSA, two systolic arrays that can be dynamically configured into weight or output stationary modes are cascaded to enable efficient attention operation. Thus, hybrid dataflows are simultaneously supported in COSA. Furthermore, various fusion methodologies and an advanced softmax unit are designed. Experimental results show that the COSA-based accelerator can achieve 2.95-28.82x speedup compared with the existing designs, with up to 97.4% PE utilization rate and less memory access.
更多查看译文
关键词
advanced softmax unit,attention mechanism acceleration,cooperative systolic array,COSA-based accelerator,deep learning tasks,efficient attention operation,fusion methodologies,higher computational efficiency,hybrid data reuse,hybrid dataflows,inherent dataflow characteristics,multihead attention mechanism,neural network models,output stationary modes
AI 理解论文
溯源树
样例
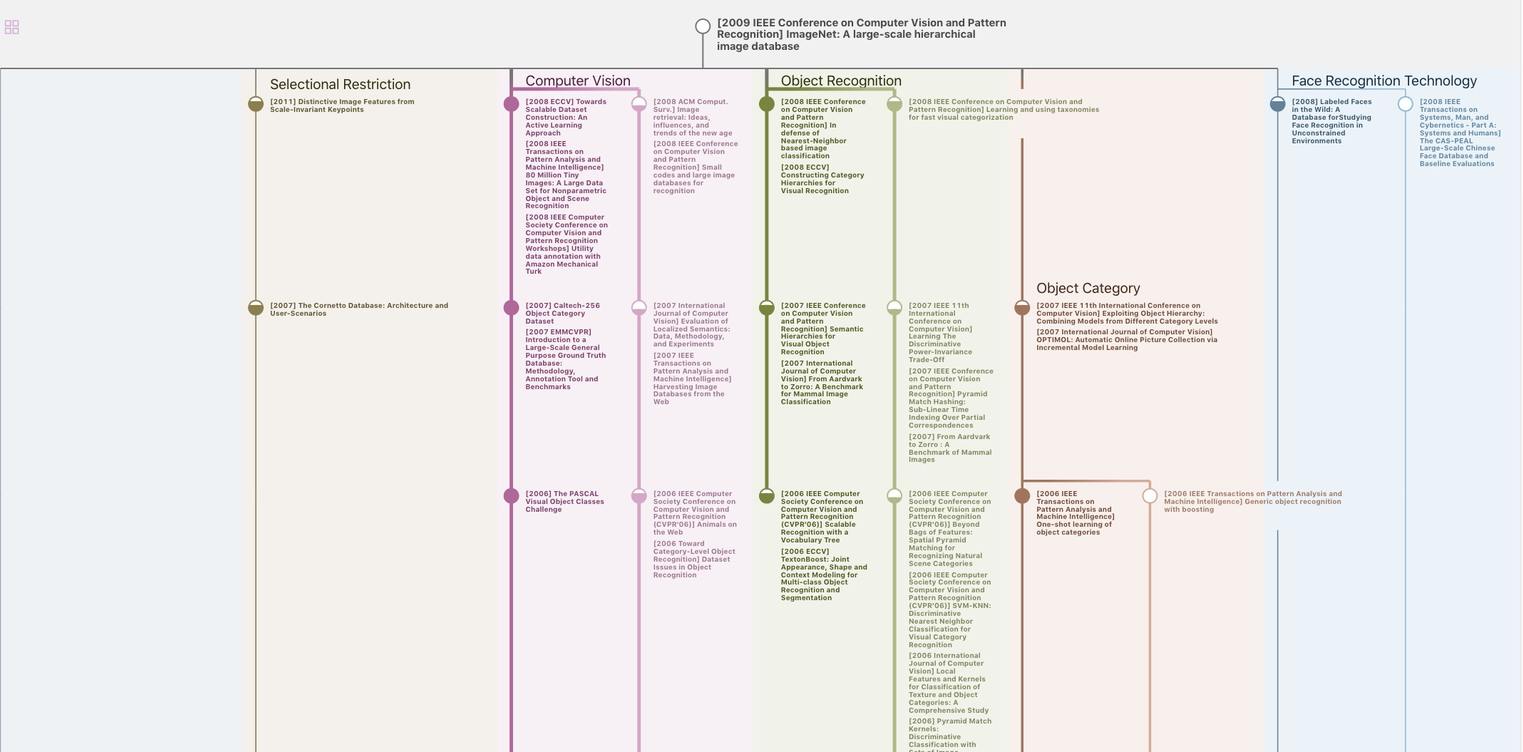
生成溯源树,研究论文发展脉络
Chat Paper
正在生成论文摘要