Cryogenic In-Memory Matrix-Vector Multiplication using Ferroelectric Superconducting Quantum Interference Device (FE-SQUID).
DAC(2023)
摘要
Next-generation quantum computing (QC) systems, comprising thousands of qubits, are envisioned to accommodate the quantum substrate (qubits) and classical components (control processor, and a digital memory block) in a cryogenic (< 4 Kelvin) environment. Such homogeneous integration will pave the way for superconducting interconnects and reduce the noise arising from thermal gradient. However, in the existing QC systems, cryogenic control processors and memory blocks are still operated following the von Neumann architecture. This leads to significant performance overhead due to the repetitive data movement between physically distinct memory and processing units. Thus, it becomes challenging to implement computationally expensive machine learning (ML) algorithms for efficient error correction and control of qubits in a QC. In-memory implementation of ML algorithms at cryogenic temperature can be a game- changer for a practical QC. Here, we demonstrate a unique technique for cryogenic in-memory matrix vector multiplication (MVM), the most frequently performed operation in ML algorithms, utilizing a ferroelectric superconducting quantum interference device (FE-SQUID)-based memory array. FE-SQUID is a promising cryogenic memory device thanks to its non-volatile nature, voltage-controlled switching, scalability, and compatibility with commercially available superconducting device fabrication processes. Moreover, due to having separate read-write paths, the read operation can be optimized without imposing any limit on the read bias and hence, multiple levels of read current with notable separation can be used to map the inputs for the MVM operation. We use an experimentally-calibrated compact model for FE-SQUID to design and test our proposed system. We evaluate FE-SQUID-based in-memory MVM by performing several classification tasks using MNIST handwritten digits, fashion, and emotion datasets. We achieve 93.83%, 80.49%, and 92.5% accuracy for handwritten digits, fashion, and sentiment classifications, respectively.
更多查看译文
关键词
cryogenic,dot product,ferroelectric SQUID,in-memory computing,matrix-vector-multiplication
AI 理解论文
溯源树
样例
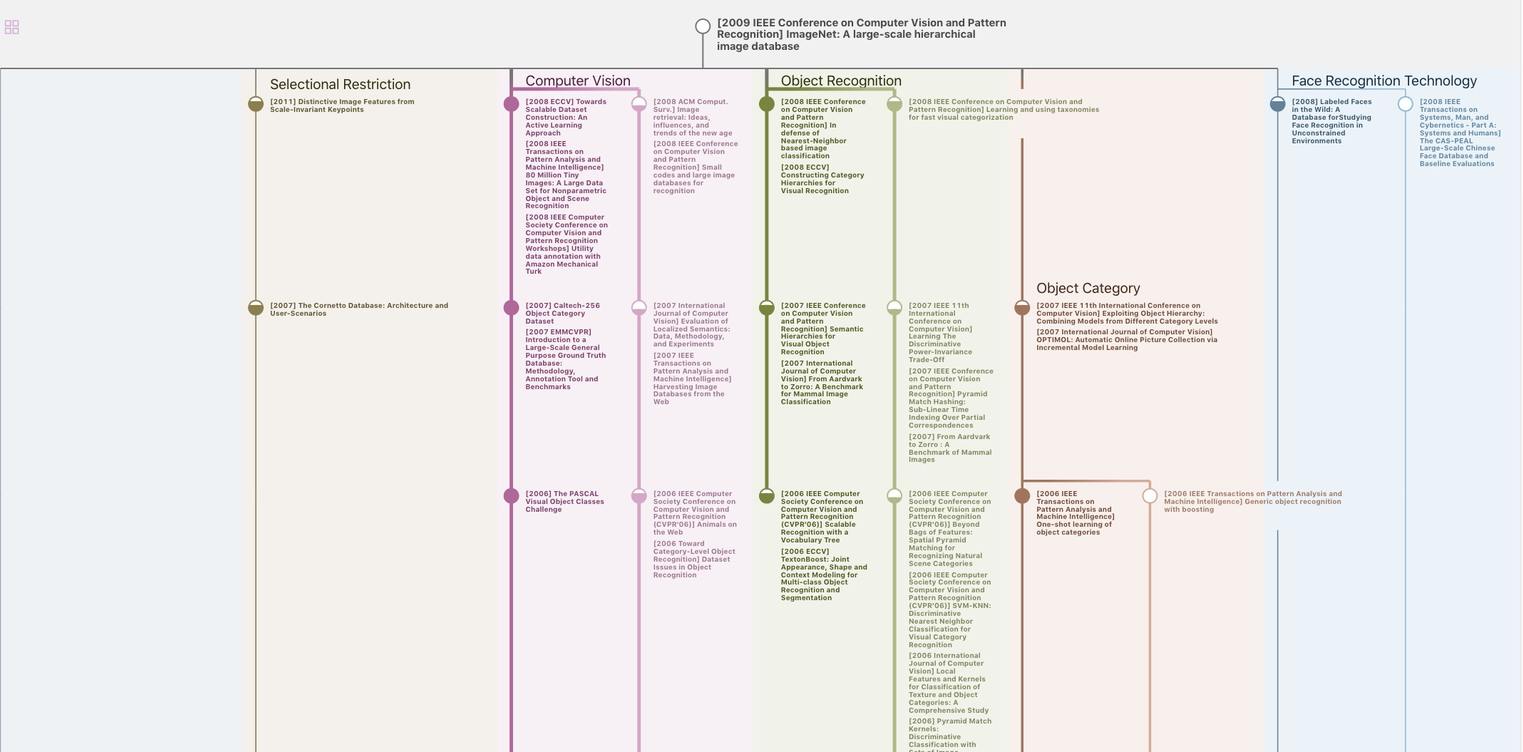
生成溯源树,研究论文发展脉络
Chat Paper
正在生成论文摘要