Late Breaking Results: Fast Fair Medical Applications? Hybrid Vision Models Achieve the Fairness on the Edge.
DAC(2023)
摘要
As edge devices become readily available and indispensable, there is an urgent need for effective and efficient intelligent applications to be deployed widespread. However, fairness has always been an issue, especially in edge medical applications. Compared to convolutional neuron networks (CNNs), Vision Transformer (ViT) has a better ability to extract global information, which will contribute to alleviating the unfairness problem. Typically, ViTs consume large amounts of computational and memory resources, which hinders their usage on edge. In this work, we propose a novel hardware-efficient Vision Model search framework for the fair dermatology classification, namely HeViFa. Experimental results show that HeViFa could search for a hybrid ViT model that reaches 173.1 FPS on a Samsung S21 mobile phone with 85.71% accuracy on the light skin dataset and 80.85% accuracy on the dark skin dataset. Note that HeViFa can reach both the highest accuracy and fairness under similar latency constrain on multiple edge devices (Samsung S21 mobile phone, iPhone 13 Pro and Raspberry PI).
更多查看译文
关键词
computational resource,edge devices,edge medical applications,fair dermatology classification,fast fair medical applications,global information extraction,hardware-efficiency vision model search framework,HeViFa,hybrid vision transformer model,hybrid ViT model,intelligent applications,iPhone 13 Pro,memory resource,Raspberry PI,Samsung S21 mobile phone
AI 理解论文
溯源树
样例
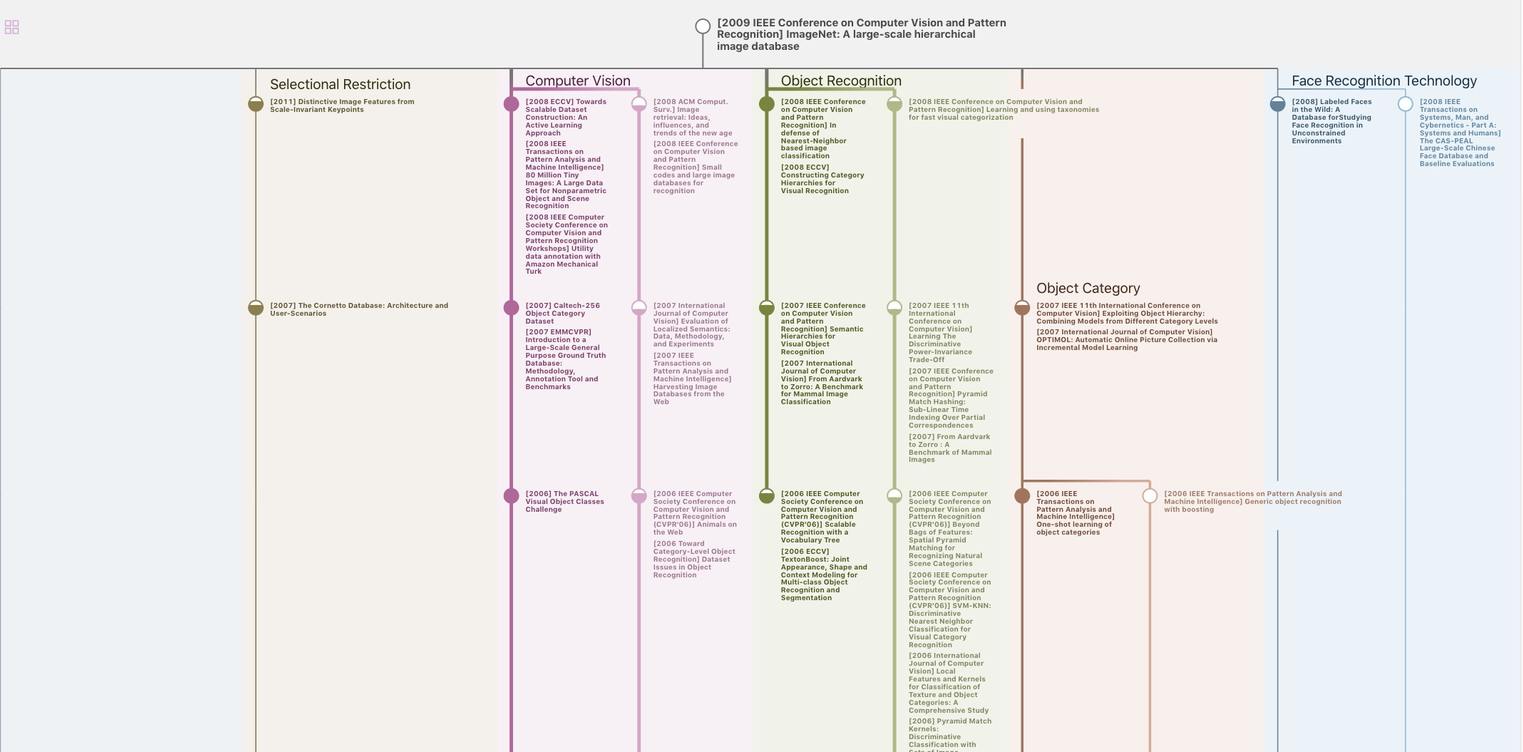
生成溯源树,研究论文发展脉络
Chat Paper
正在生成论文摘要