A Multi-channel EEG Data Analysis for Poor Neuro-prognostication in Comatose Patients with Self and Cross-channel Attention Mechanism
CoRR(2023)
摘要
This work investigates the predictive potential of bipolar electroencephalogram (EEG) recordings towards efficient prediction of poor neurological outcomes. A retrospective design using a hybrid deep learning approach is utilized to optimize an objective function aiming for high specificity, i.e., true positive rate (TPR) with reduced false positives (< 0.05). A multi-channel EEG array of 18 bipolar channel pairs from a randomly selected 5-minute segment in an hour is kept. In order to determine the outcome prediction, a combination of a feature encoder with 1-D convolutional layers, learnable position encoding, a context network with attention mechanisms, and finally, a regressor and classifier blocks are used. The feature encoder extricates local temporal and spatial features, while the following position encoding and attention mechanisms attempt to capture global temporal dependencies. Results: The proposed framework by our team, OUS IVS, when validated on the challenge hidden validation data, exhibited a score of 0.57.
更多查看译文
关键词
Deep Learning,PhysioNet,Attention Mechanism,Neuro-prognosis,Cerebral Performance Category,Transformers
AI 理解论文
溯源树
样例
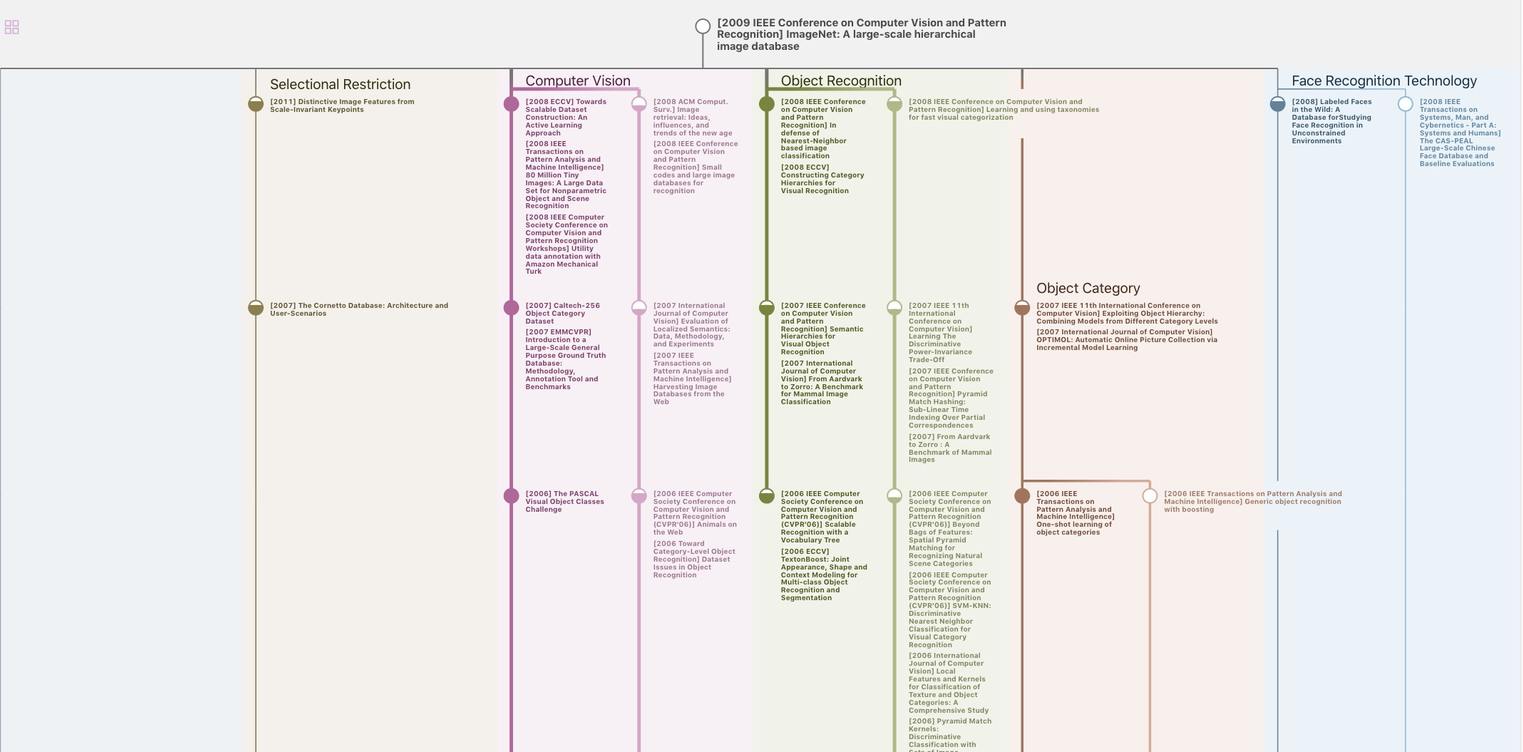
生成溯源树,研究论文发展脉络
Chat Paper
正在生成论文摘要