Toward a Foundation Model for Time Series Data
PROCEEDINGS OF THE 32ND ACM INTERNATIONAL CONFERENCE ON INFORMATION AND KNOWLEDGE MANAGEMENT, CIKM 2023(2023)
摘要
A foundation model is a machine learning model trained on a large and diverse set of data, typically using self-supervised learning-based pre-training techniques, that can be adapted to various downstream tasks. However, current research on time series pre-training has predominantly focused on models trained exclusively on data from a single domain. As a result, these models possess domain-specific knowledge that may not be easily transferable to time series from other domains. In this paper, we aim to develop an effective time series foundation model by leveraging unlabeled samples from multiple domains. To achieve this, we repurposed the publicly available UCR Archive and evaluated four existing self-supervised learning-based pre-training methods, along with a novel method, on the datasets. We tested these methods using four popular neural network architectures for time series to understand how the pre-training methods interact with different network designs. Our experimental results show that pre-training improves downstream classification tasks by enhancing the convergence of the fine-tuning process. Furthermore, we found that the proposed pre-training method, when combined with the Transformer, outperforms the alternatives. The proposed method outperforms or achieves equal performance compared to the second best method in similar to 93% of downstream tasks.
更多查看译文
关键词
time series,self-supervised learning,foundation model
AI 理解论文
溯源树
样例
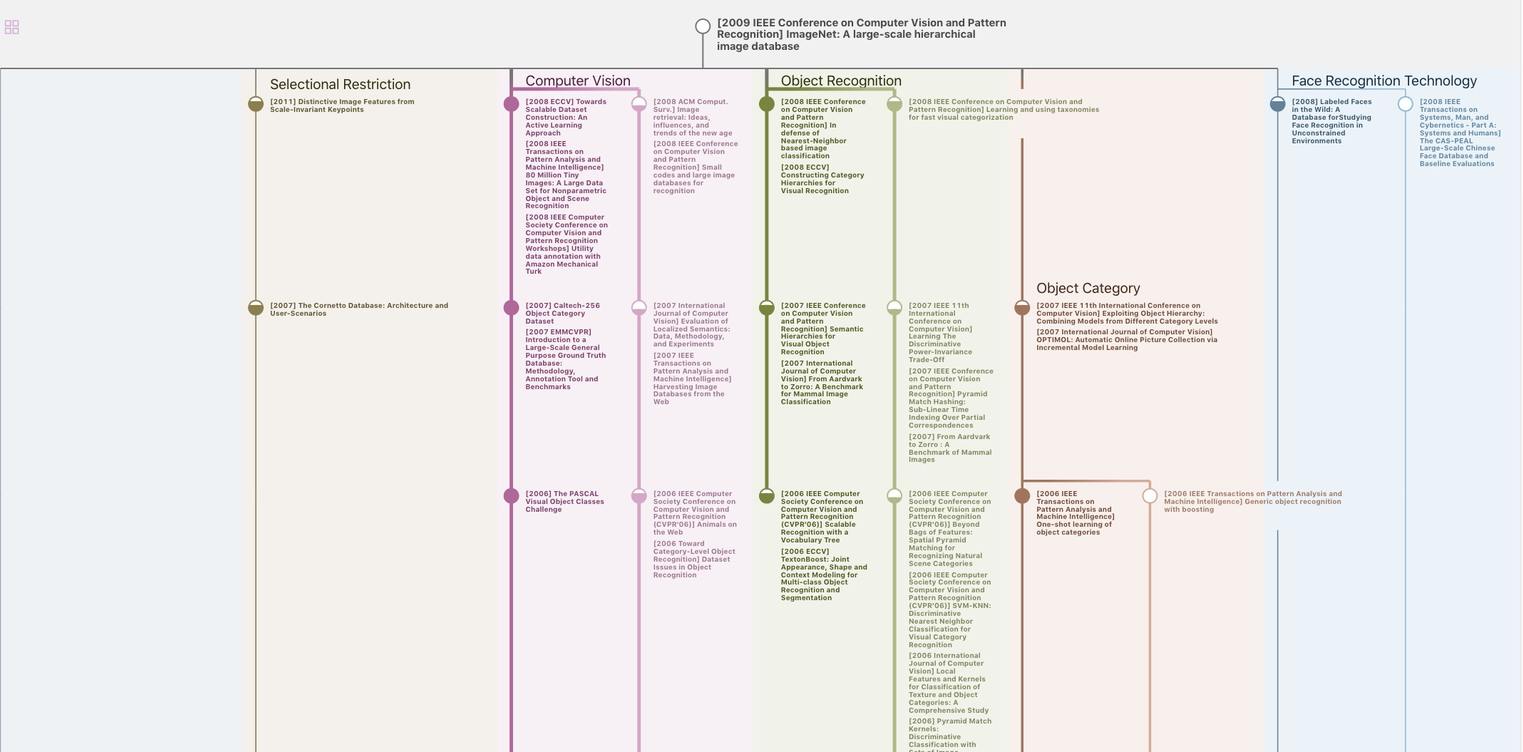
生成溯源树,研究论文发展脉络
Chat Paper
正在生成论文摘要