SIFT – File Fragment Classification Without Metadata
CoRR(2023)
摘要
A vital issue of file carving in digital forensics is type classification of file fragments when the filesystem metadata is missing. Over the past decades, there have been several efforts for developing methods to classify file fragments. In this research, a novel sifting approach, named SIFT (Sifting File Types), is proposed. SIFT outperforms the other state-of-the-art techniques by at least 8%. (1) One of the significant differences between SIFT and others is that SIFT uses a single byte as a separate feature, i.e., a total of 256 (0×00 – 0×FF) features. We also call this a lossless feature (information) extraction, i.e., there is no loss of information. (2) The other significant difference is the technique used to estimate inter-Classes and intra-Classes information gain of a feature. Unlike others, SIFT adapts TF-IDF for this purpose, and computes and assigns weight to each byte (feature) in a fragment (sample). With these significant differences and approaches, SIFT produces promising (better) results compared to other works.
更多查看译文
关键词
Digital forensics,File fragmentation,File types,Classification
AI 理解论文
溯源树
样例
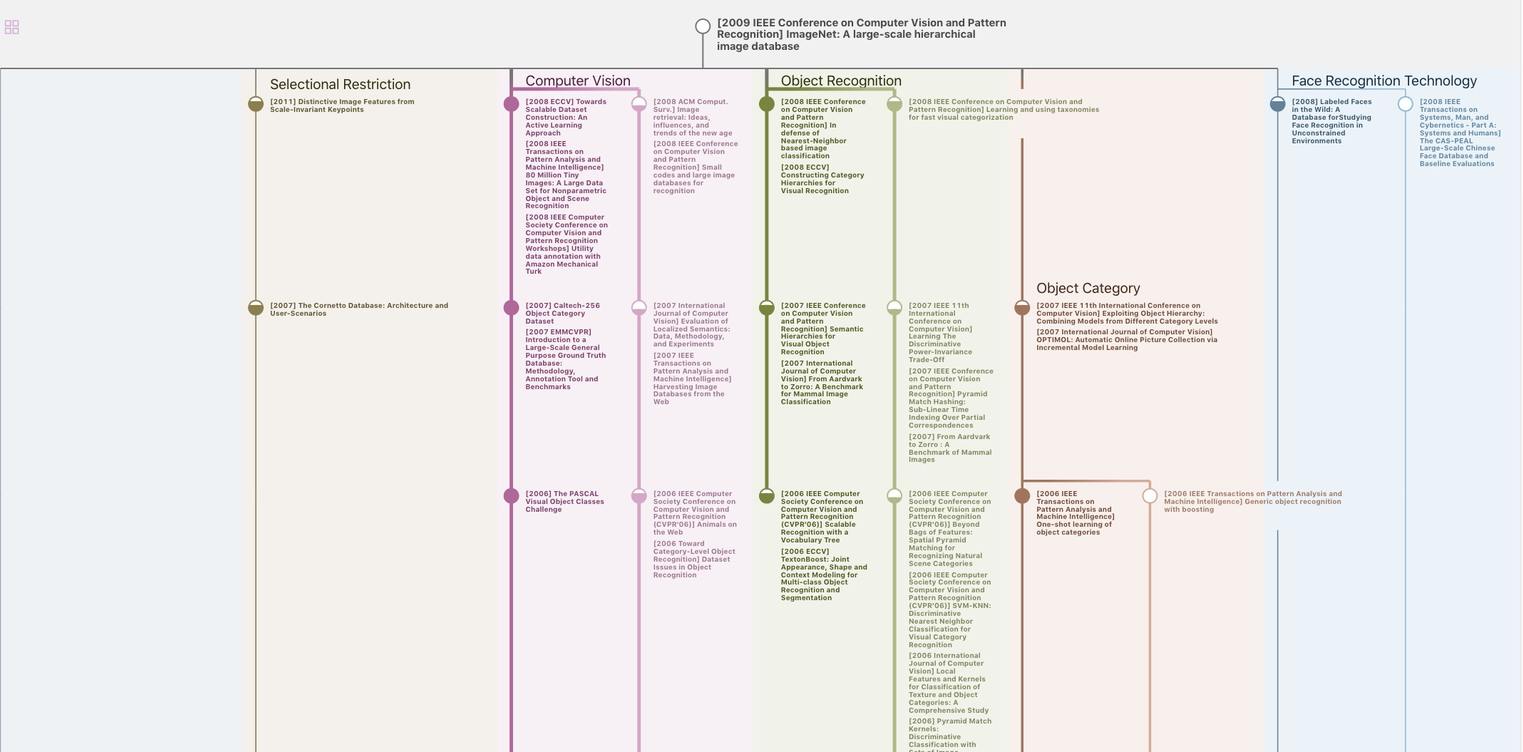
生成溯源树,研究论文发展脉络
Chat Paper
正在生成论文摘要