Accelerated Affine-Invariant Convergence Rates of the Frank-Wolfe Algorithm with Open-Loop Step-Sizes
arXiv (Cornell University)(2023)
摘要
Recent papers have shown that the Frank-Wolfe algorithm (FW) with open-loop step-sizes exhibits rates of convergence faster than the iconic $\mathcal{O}(t^{-1})$ rate. In particular, when the minimizer of a strongly convex function over a polytope lies in the relative interior of a feasible region face, the FW with open-loop step-sizes $\eta_t = \frac{\ell}{t+\ell}$ for $\ell \in \mathbb{N}_{\geq 2}$ has accelerated convergence $\mathcal{O}(t^{-2})$ in contrast to the rate $\Omega(t^{-1-\epsilon})$ attainable with more complex line-search or short-step step-sizes. Given the relevance of this scenario in data science problems, research has grown to explore the settings enabling acceleration in open-loop FW. However, despite FW's well-known affine invariance, existing acceleration results for open-loop FW are affine-dependent. This paper remedies this gap in the literature by merging two recent research trajectories: affine invariance (Wirth et al., 2023b) and open-loop step-sizes (Pena, 2021). In particular, we extend all known non-affine-invariant convergence rates for FW with open-loop step-sizes to affine-invariant results.
更多查看译文
关键词
algorithm,affine-invariant,frank-wolfe,open-loop,step-sizes
AI 理解论文
溯源树
样例
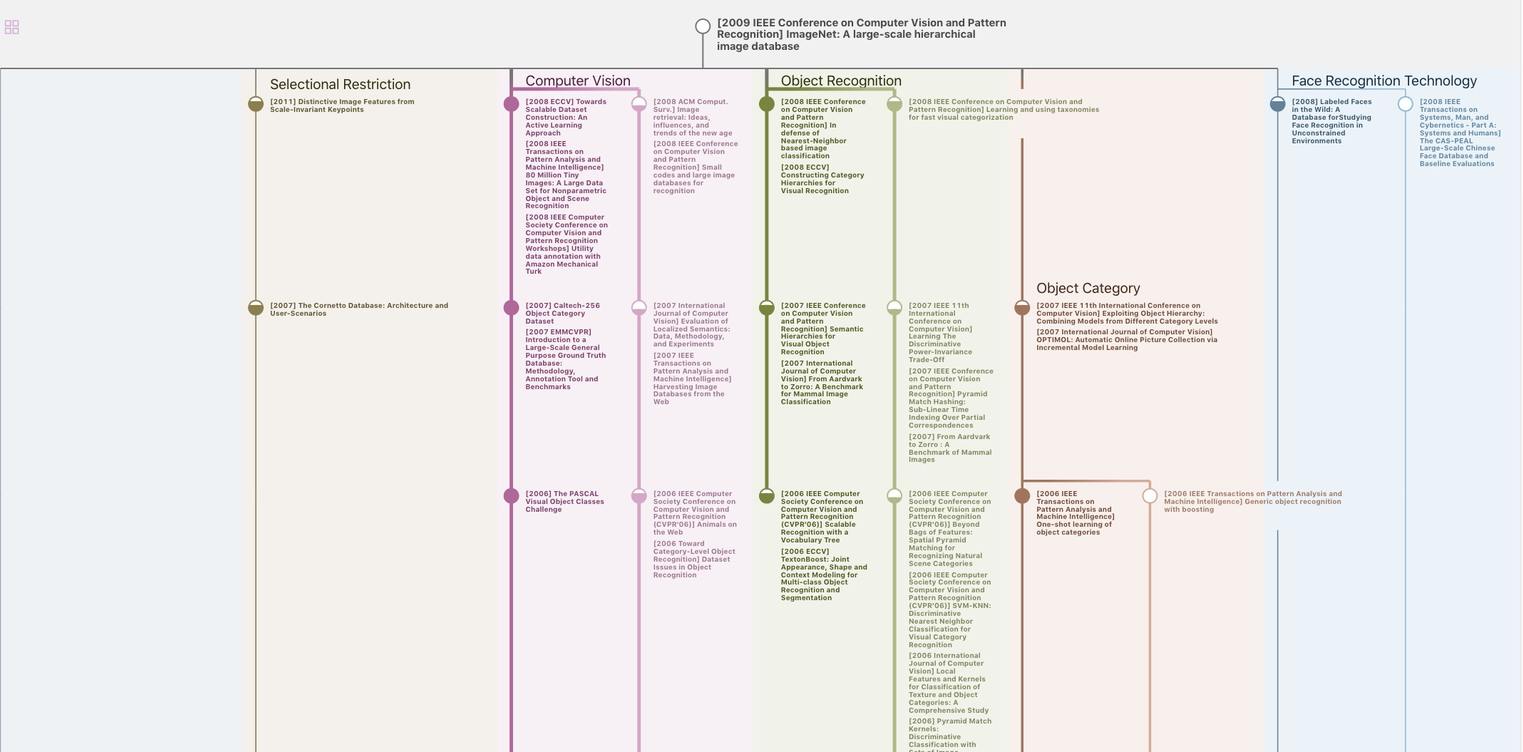
生成溯源树,研究论文发展脉络
Chat Paper
正在生成论文摘要