Accelerating optimization over the space of probability measures
CoRR(2023)
摘要
Acceleration of gradient-based optimization methods is an issue of significant practical and theoretical interest, particularly in machine learning applications. Most research has focused on optimization over Euclidean spaces, but given the need to optimize over spaces of probability measures in many machine learning problems, it is of interest to investigate accelerated gradient methods in this context too. To this end, we introduce a Hamiltonian-flow approach that is analogous to moment-based approaches in Euclidean space. We demonstrate that algorithms based on this approach can achieve convergence rates of arbitrarily high order. Numerical examples illustrate our claim.
更多查看译文
关键词
measures,optimization
AI 理解论文
溯源树
样例
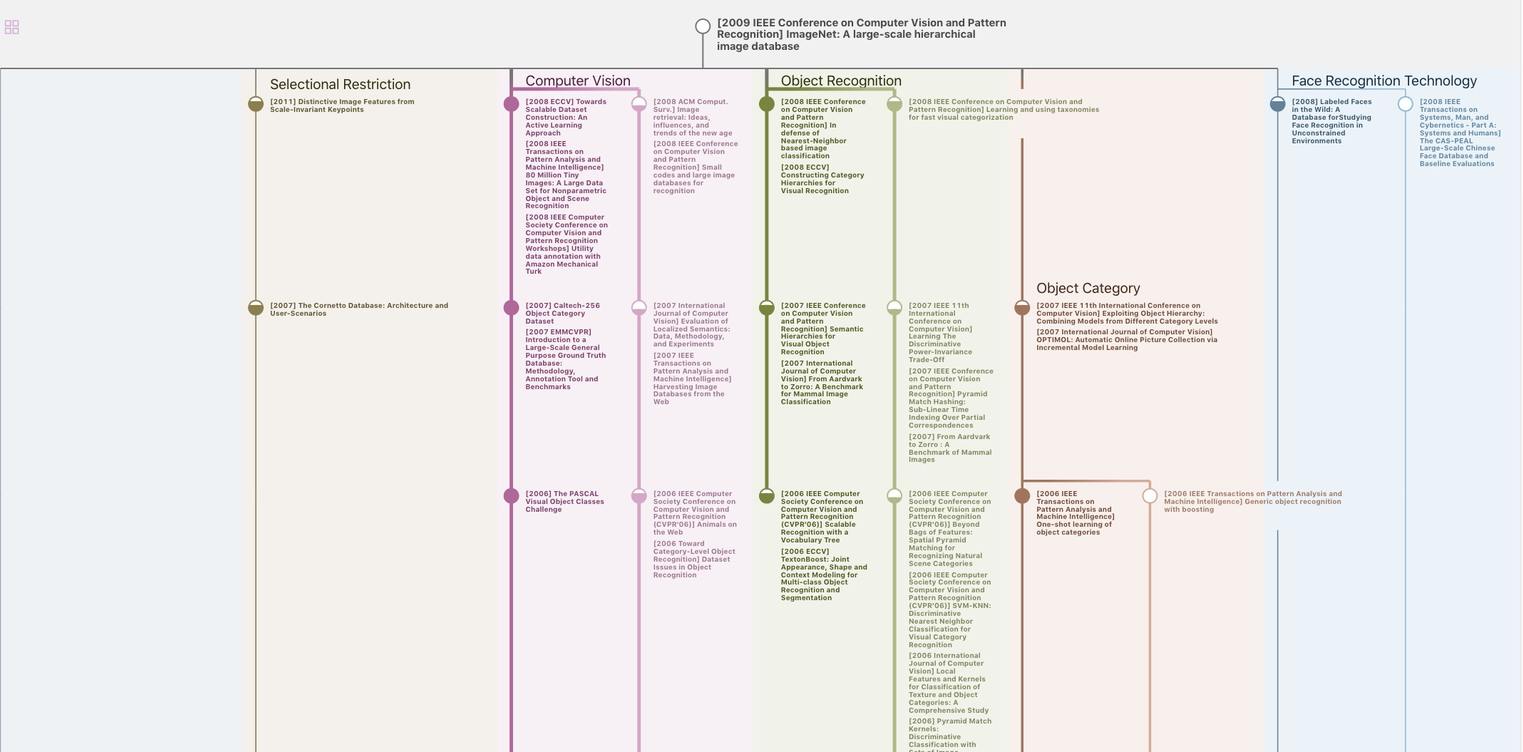
生成溯源树,研究论文发展脉络
Chat Paper
正在生成论文摘要