Improving the accuracy of polar motion prediction using a hybrid least squares and long short-term memory model
EARTH PLANETS AND SPACE(2023)
摘要
Earth rotation parameters (ERPs) are essential for transforming between the celestial and terrestrial reference frames, and for high-precision space navigation and positioning. Among the ERPs, polar motion (PM) is a critical parameter for analyzing and understanding the dynamic interaction between the solid Earth, atmosphere, ocean, and other geophysical fluids. Traditional methods for predicting the change in ERPs rely heavily on linear models, such as the least squares (LS) and the autoregressive (AR) model (LS + AR). However, variations in ERP partly reflect non-linear effects in the Earth system, such that the predictive accuracy of linear models is not always optimal. In this paper, long short-term memory (LSTM), a non-linear neural network, is employed to improve the prediction of ERPs. Polar motion prediction experiments in this study are conducted using the LSTM model and a hybrid method LS + LSTM model based on the IERS EOP14C04 time series. Compared with Bulletin A, the PMX and PMY prediction accuracy can reach a maximum of 33.7% and 31.9%, respectively, with the LS + LSTM model. The experimental results show that the proposed hybrid model displays a better performance in mid- and long-term (120–365 days) prediction of polar motion. Graphical Abstract
更多查看译文
关键词
Earth rotation parameters,Polar motion,Long short-term memory,Least squares and the autoregressive model,Bulletin A,LS plus LSTM model
AI 理解论文
溯源树
样例
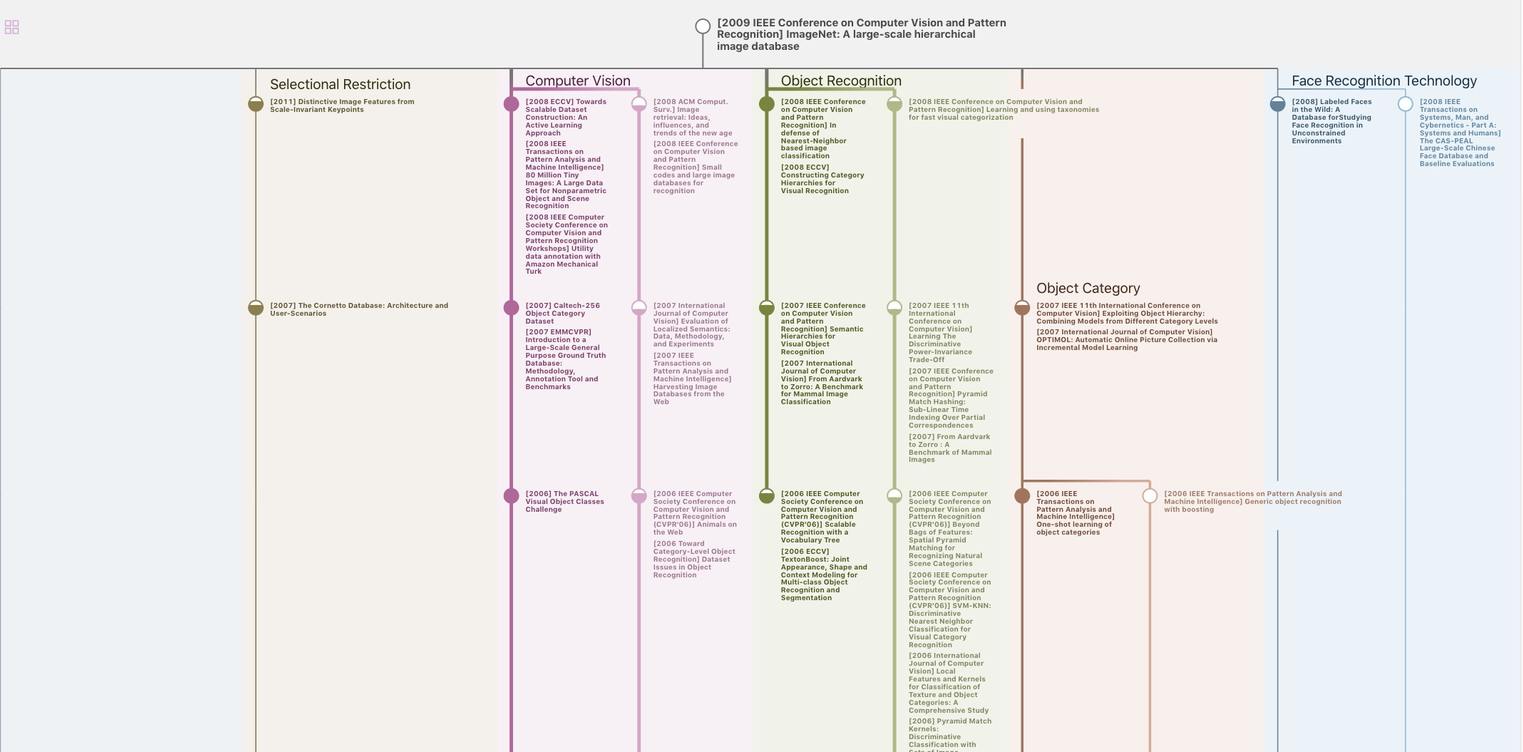
生成溯源树,研究论文发展脉络
Chat Paper
正在生成论文摘要