Energy Aware Collaborative Machine Learning on Energy-Harvesting Devices
2023 International Conference on Consumer Electronics - Taiwan (ICCE-Taiwan)(2023)
摘要
Performing machine learning tasks on low end devices enables the development of various smart applications. Especially, these low end devices are often equipped with ultra-low-power microcontroller units (MCUs) that have weak computation power and few memory resources. It is a more challenging work to put these machine learning tasks on those end devices powered by harvested ambient energy, which are often referred to as energy-harvesting (EH) devices, since the unstable ambient energy can lead to the execution failure of the machine learning tasks. This paper proposes an adaptive energy-aware design to coordinate multiple EH devices to accomplish multi-class classification computation. It also leverages the concept of the One-vs-All (OVA) strategy turning a multi-class classification into multiple binary classifications. The experimental results show our work performs better than the widely used round-robin policy and self-greedy policy in consideration of time and energy consumption.
更多查看译文
关键词
TinyML,Energy Harvesting,IoT,self-powered system
AI 理解论文
溯源树
样例
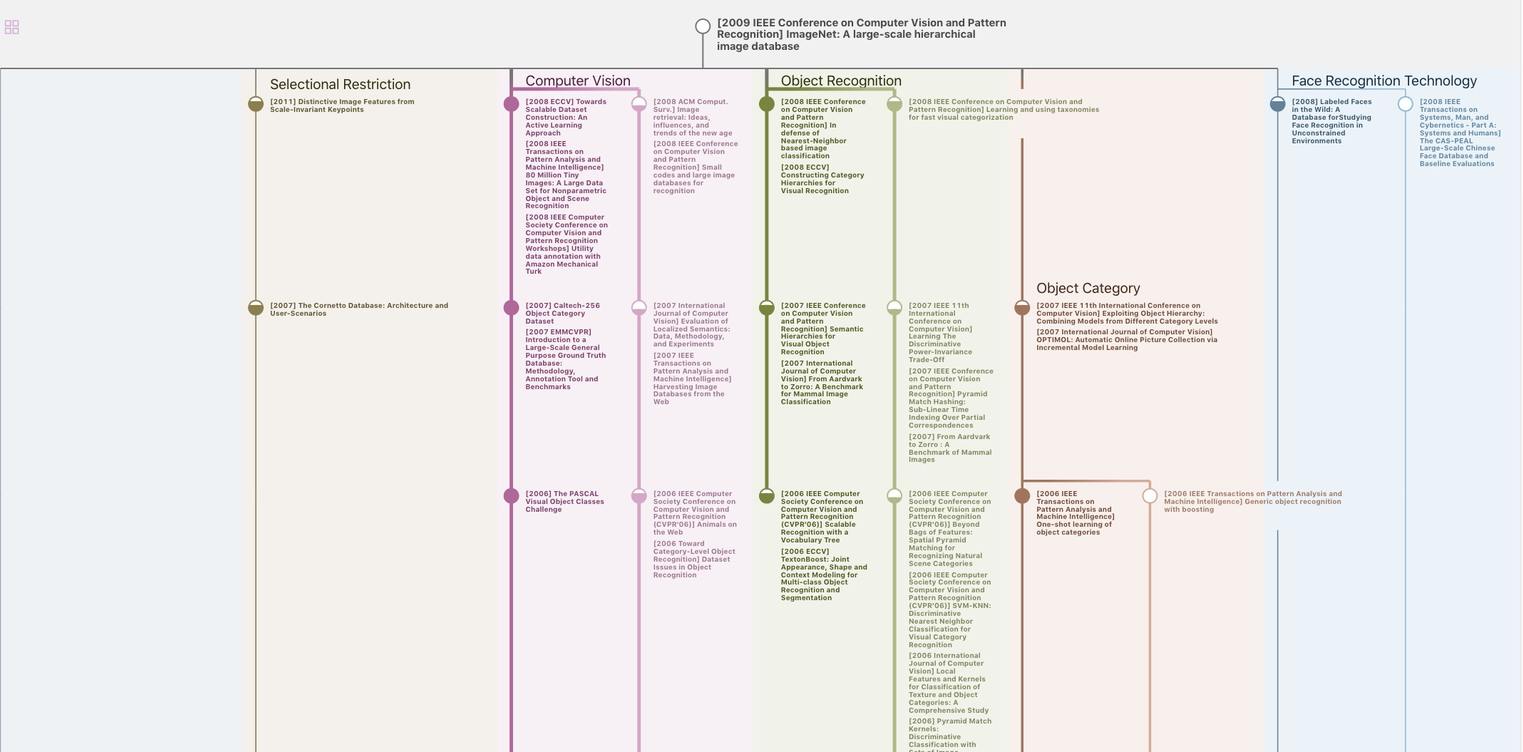
生成溯源树,研究论文发展脉络
Chat Paper
正在生成论文摘要