Kill Two Birds With One Stone: A Multi-View Multi-Adversarial Learning Approach for Joint Air Quality and Weather Prediction
IEEE Transactions on Knowledge and Data Engineering(2023)
摘要
Accurate and timely air quality and weather predictions are of great importance to urban governance and human livelihood. Though many efforts have been made for air quality or weather prediction, most of them simply employ one another as feature input, which ignores the inner-connection between two predictive tasks. On one hand, the accurate prediction of one task can help improve another task's performance. On the other hand, geospatially distributed air quality and weather monitoring stations provide additional hints for city-wide spatiotemporal dependency modeling. Inspired by the above two insights, in this paper, we propose a multi-view multi-adversarial approach, entitled MasterGNN
$^{+}$
, to jointly predict air quality and weather conditions. First, we devise a multi-view graph learning block to model spatial autocorrelation based on geographical distance and environmental context. Then, a dedicated evolved recurrent network is proposed to dynamically capture the long-range and independent temporal autocorrelation for each monitoring station and time slot. After that, we develop a multi-adversarial graph learning framework to against observation noise propagation introduced by spatiotemporal modeling. Moreover, we present an adaptive training strategy by formulating multi-adversarial learning as a multi-task learning problem. Finally, extensive experiments on two real-world datasets show that MasterGNN
$^{+}$
achieves the best performance compared with seven baselines on both air quality and weather prediction tasks.
更多查看译文
关键词
Adversarial learning, air quality forecasting, graph neural network, weather forecasting
AI 理解论文
溯源树
样例
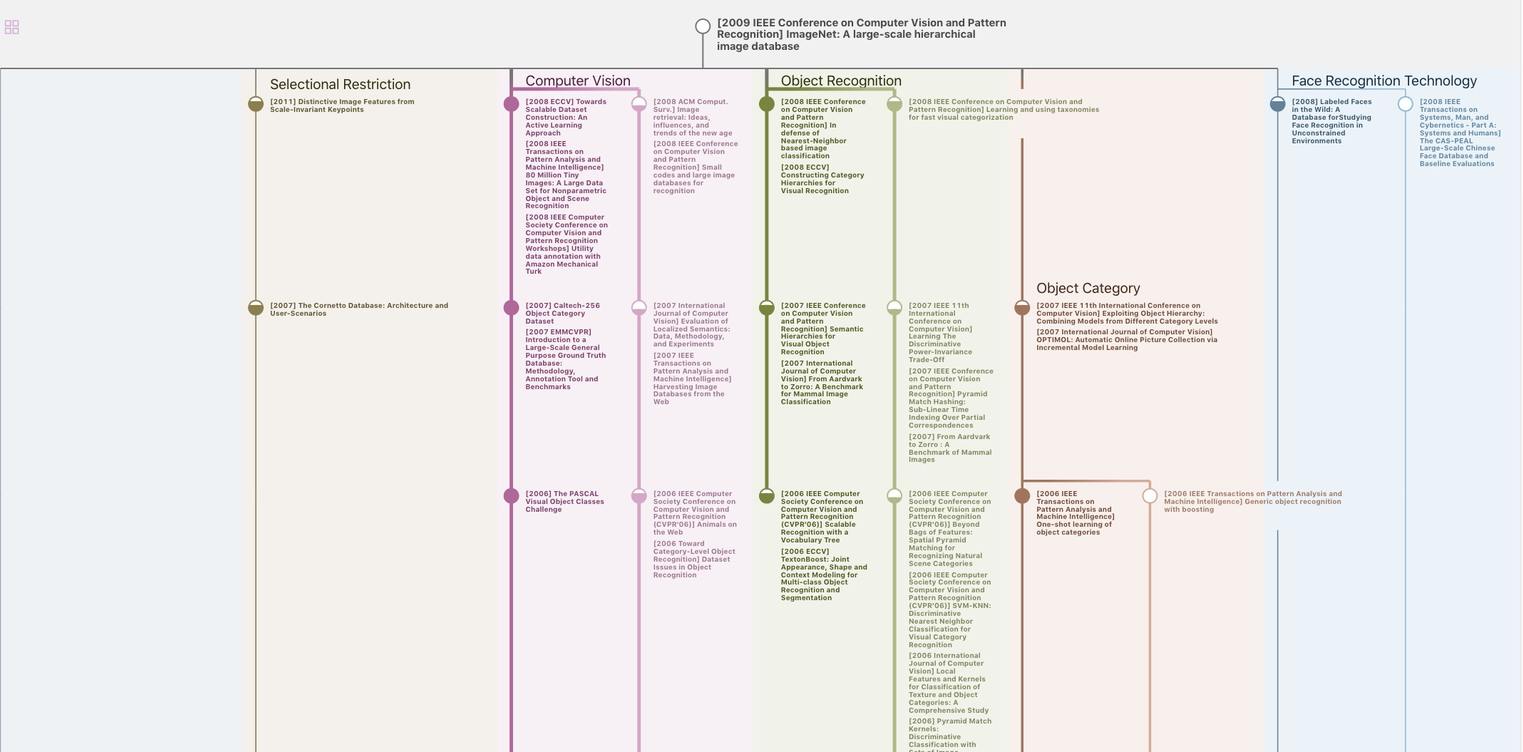
生成溯源树,研究论文发展脉络
Chat Paper
正在生成论文摘要