Global Ontology Entities Embeddings
IEEE Transactions on Knowledge and Data Engineering(2023)
摘要
Ontologies are among the most widely used types of knowledge representation formalisms. The application of deep learning techniques in the field of ontology engineering has reinforced the need to learn and generate representations of ontological data. This allows ontologies to be exploited by such models, and thus automate various ontology engineering tasks, where most of the existing tools and machine learning approaches require a numerical feature vectors associated with each concept. This paper outlines a novel approach for learning global ontology entities embeddings by exploiting the structure and the various taxonomic and semantic relationships present in ontologies, taking into account all the information present in the ontological graph and carried by the OWL/RDF triples. Thus, producing global ontology entities embeddings capturing the global ontological graph semantics and similarities enclosed in the source ontology. Three different neural network models have been proposed based on two architectures: multi-input and multi-output, trained using the contrastive estimation technique. The evaluation on OWL/RDF ontologies and word semantic similarity tasks using various graph and WordNet based similarity measures, show that our approach yields competitive results outperforming the state-of-the-art ontology and word embedding models.
更多查看译文
关键词
Concept embeddings,feature representation,neural networks,ontology embeddings,ontology entities vector representations
AI 理解论文
溯源树
样例
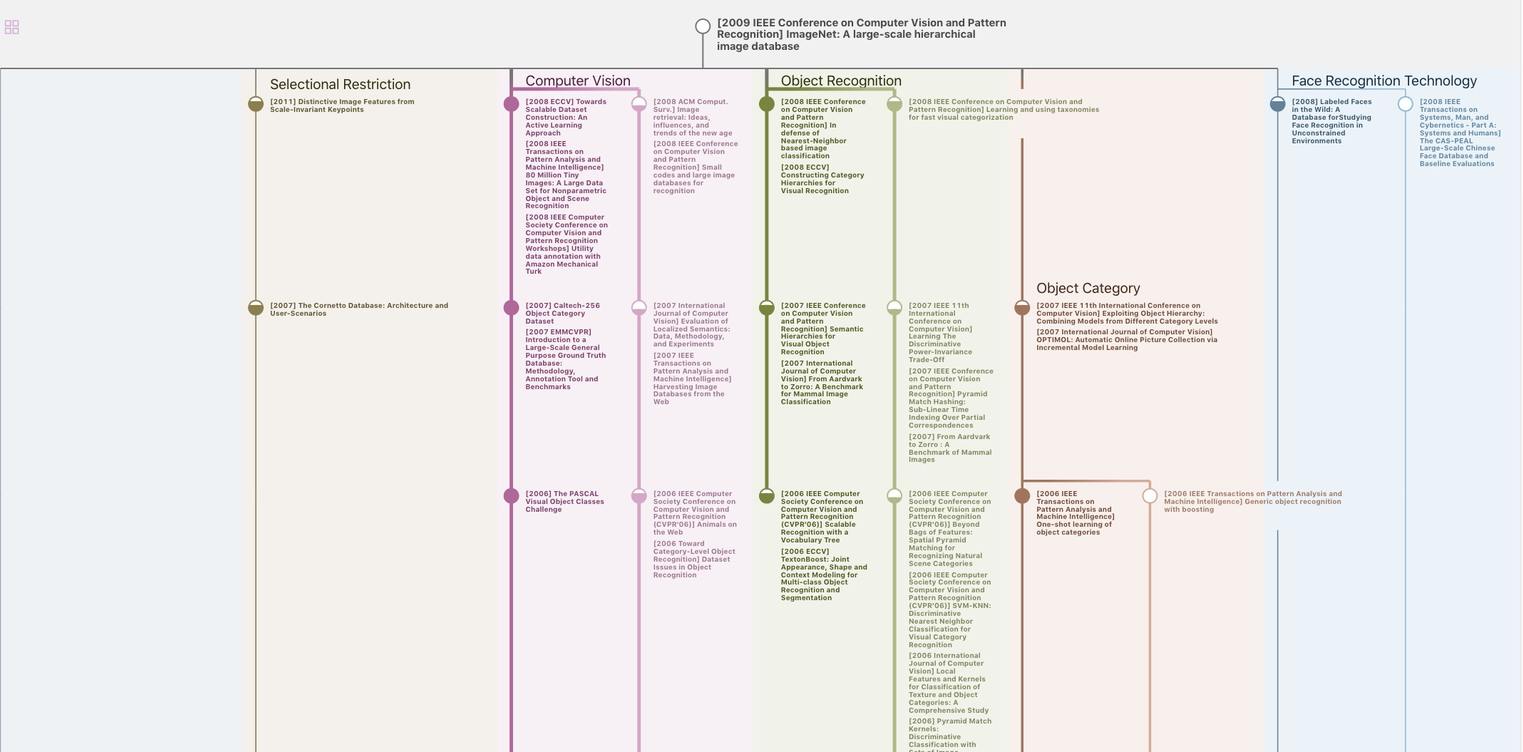
生成溯源树,研究论文发展脉络
Chat Paper
正在生成论文摘要