Character Encoding-Based Motor Imagery EEG Classification Using CNN
IEEE Sensors Letters(2023)
摘要
This letter proposes a simple and effective EEG classification method using convolutional neural networks (CNNs). The EEG signals are first converted to character sequences in order to simplify feature extraction and meanwhile reduce data dimensionality. The character sequences are then transformed according to binary encoding and finally fed into a specific CNN, which gives consideration to both classification accuracy and computation efficiency. Experimental simulations show that the proposed method has the advantages of simple preprocessing and lightweight network structure and that the average accuracy on two BCI Competition datasets can reach 94.36% and 81.8%, whereas the average accuracy on the self-made dataset is 71.67%, outperforming traditional methods. Furthermore, the proposed algorithm has been successfully applied to control the robotic arm of an EEG-based manipulator system, further validating its feasibility.
更多查看译文
关键词
Electroencephalography, Convolution, Sensors, Convolutional neural networks, Classification algorithms, Feature extraction, Brain modeling, Sensor applications, brain-computer interface, character encoding, convolutional neural network (CNN), motor imagery
AI 理解论文
溯源树
样例
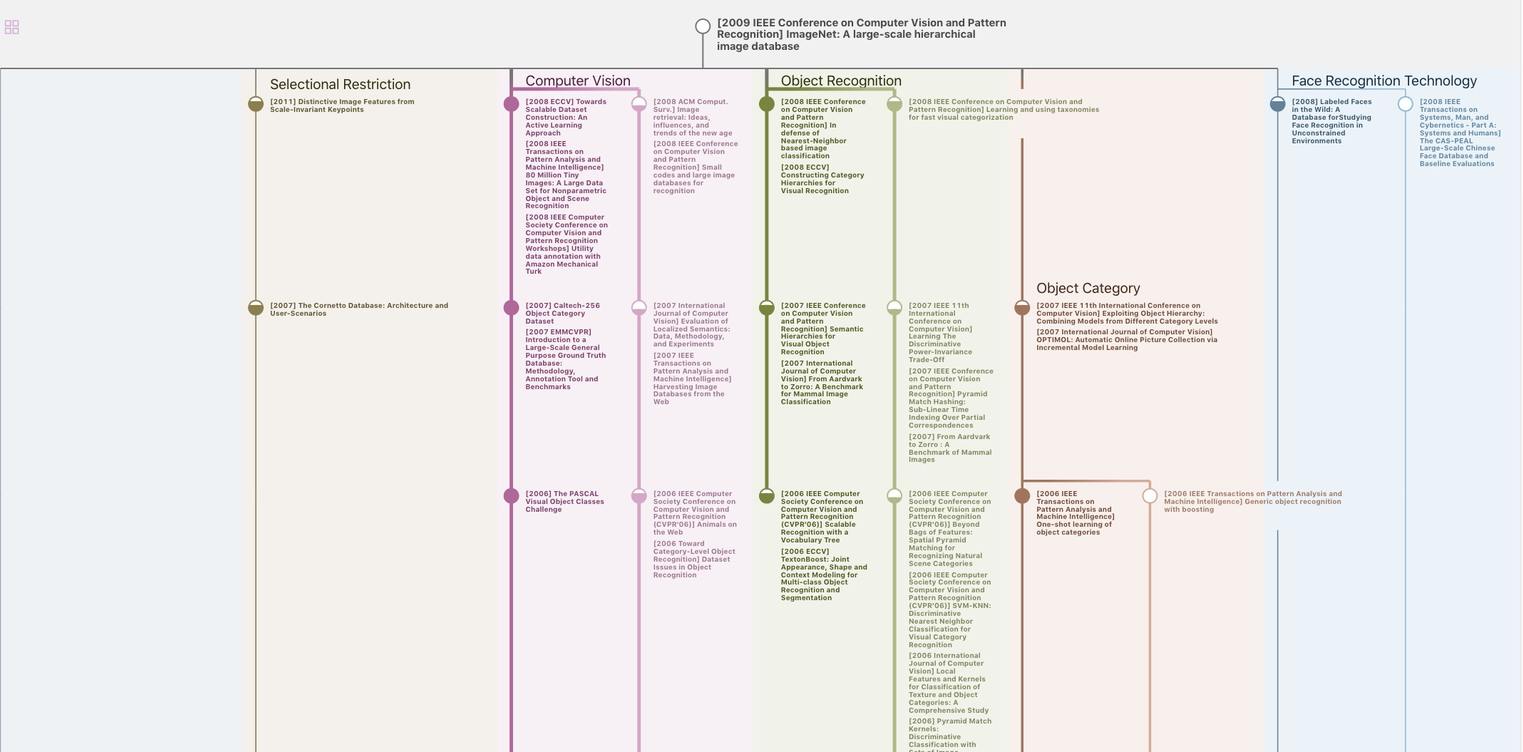
生成溯源树,研究论文发展脉络
Chat Paper
正在生成论文摘要