PhyGCN: Pre-trained Hypergraph Convolutional Neural Networks with Self-supervised Learning
bioRxiv : the preprint server for biology(2023)
摘要
Hypergraphs are powerful tools for modeling complex interactions across various domains, including biomedicine. However, learning meaningful node representations from hypergraphs remains a challenge. Existing supervised methods often lack generalizability, thereby limiting their real-world applications. We propose a new method, Pre-trained Hypergraph Convolutional Neural Networks with Self-supervised Learning (PhyGCN), which leverages hypergraph structure for self-supervision to enhance node representations. PhyGCN introduces a unique training strategy that integrates variable hyperedge sizes with self-supervised learning, enabling improved generalization to unseen data. Applications on multi-way chromatin interactions and polypharmacy side-effects demonstrate the effectiveness of PhyGCN. As a generic framework for high-order interaction datasets with abundant unlabeled data, PhyGCN holds strong potential for enhancing hypergraph node representations across various domains.
### Competing Interest Statement
The authors have declared no competing interest.
更多查看译文
关键词
hypergraph convolutional neural networks,convolutional neural networks,neural networks,learning,pre-trained,self-supervised
AI 理解论文
溯源树
样例
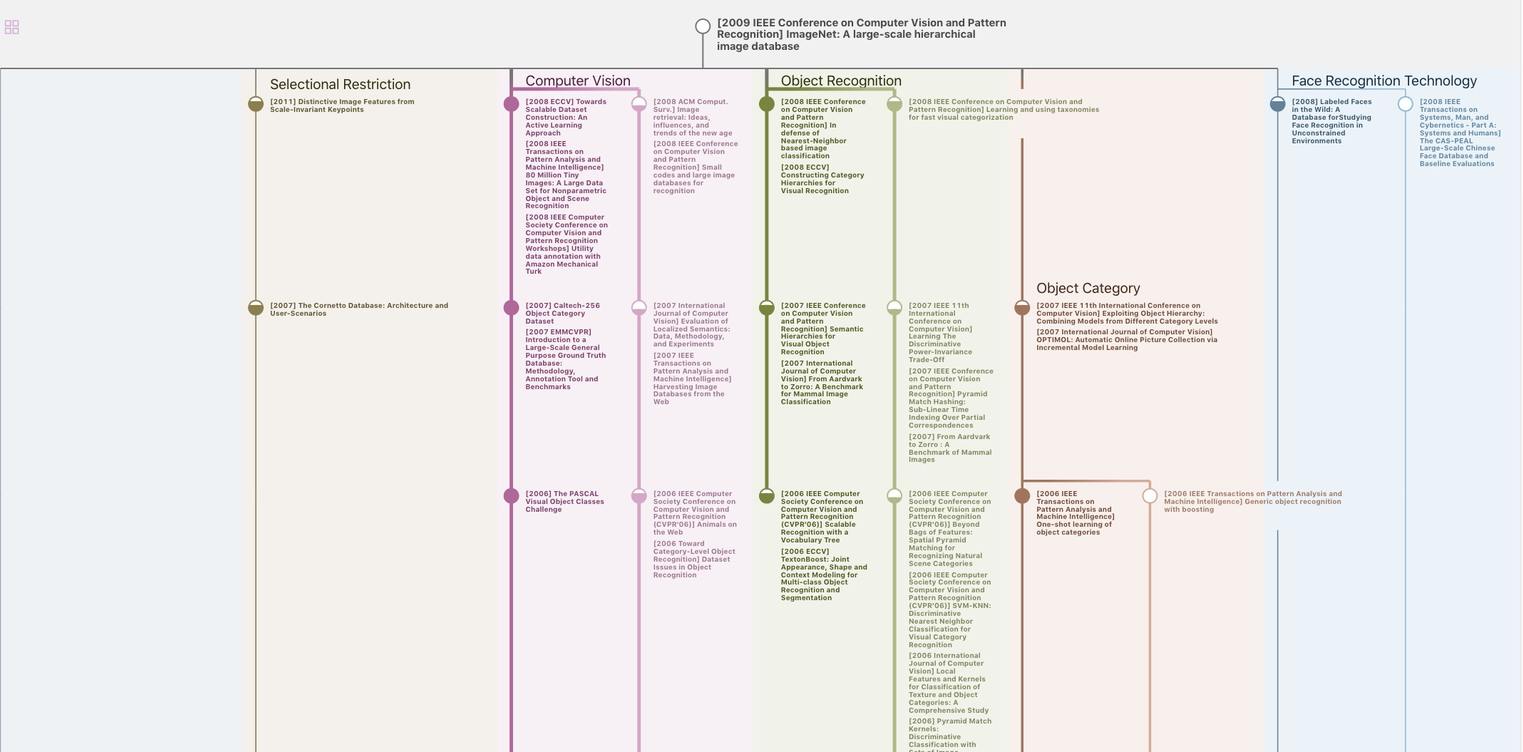
生成溯源树,研究论文发展脉络
Chat Paper
正在生成论文摘要