Missing view completion for multi-timepoints omics data via representation disentanglement and temporal knowledge transfer
bioRxiv (Cold Spring Harbor Laboratory)(2023)
摘要
Longitudinal multi-view omics data offer unique insights into the temporal dynamics of individual-level physiology that is instrumental to advancements in personalized healthcare. However, the common occurrence of incomplete view makes inference tasks difficult, and there is a lack of tailored methods for solving this critical issue. Here, we introduce LEOPARD, an approach specifically designed to complete missing views for multi-timepoint omics data. By disentangling longitudinal omics data into content and temporal representations, LEOPARD transfers temporal knowledge to omics-specific content to complete missing views. Compared to generic imputation methods, LEOPARD gives the most robust and reliable results across three longitudinal proteomics and metabolomics datasets. LEOPARD-imputed data also achieve the closest performance to observed data in our regression and classification analyses. Our work takes the first step towards a principled and generalized treatment of missing views in longitudinal omics data, enabling comprehensive exploration of temporal changes within omics data.
### Competing Interest Statement
The authors have declared no competing interest.
更多查看译文
关键词
missing view completion,representation disentanglement,multi-timepoints
AI 理解论文
溯源树
样例
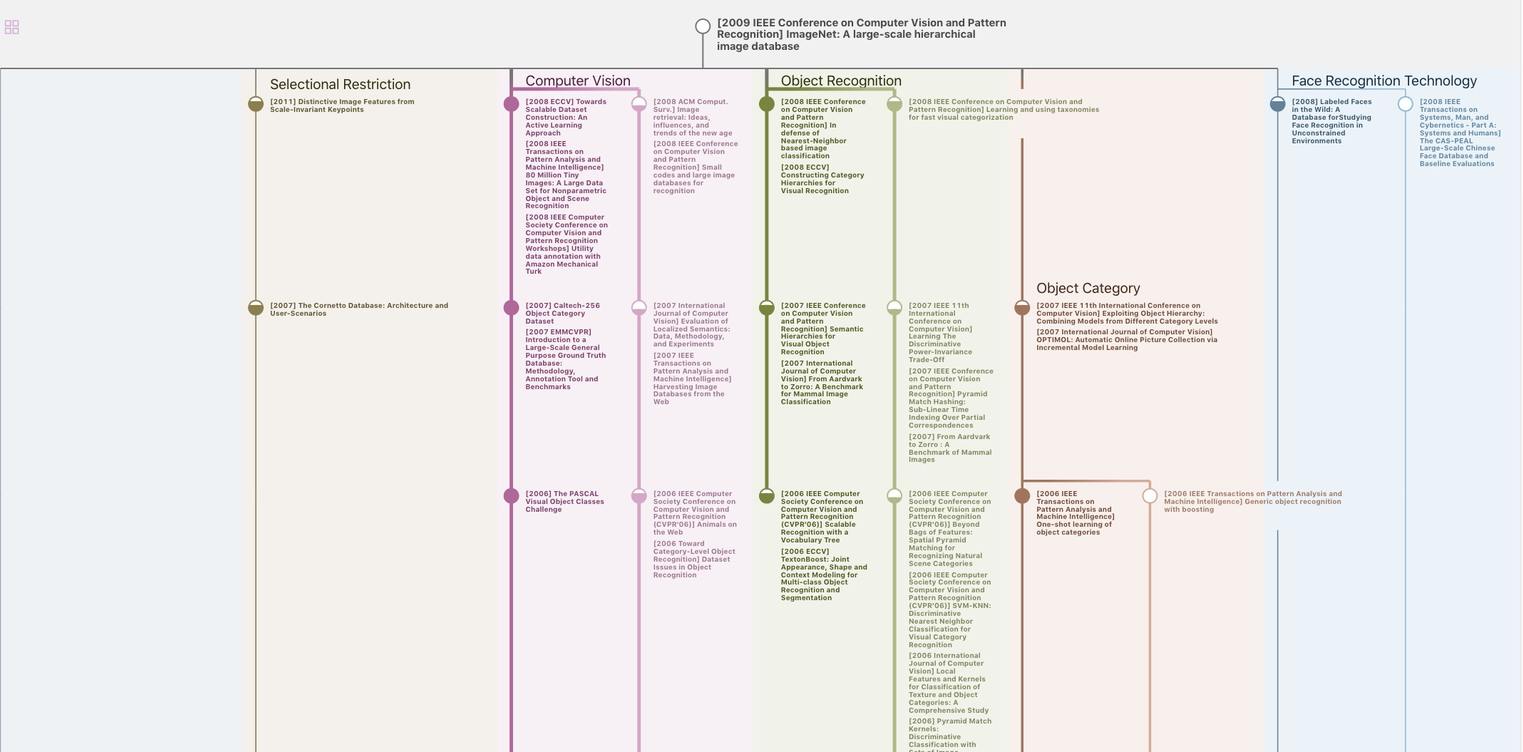
生成溯源树,研究论文发展脉络
Chat Paper
正在生成论文摘要