The winner’s curse under dependence: repairing empirical Bayes and a comparative study
bioRxiv (Cold Spring Harbor Laboratory)(2023)
摘要
Motivation The winner’s curse is a form of selection bias that arises when estimates are obtained for a large number of features, but only a subset of most extreme estimates is reported. It occurs in large scale significance testing as well as in rank-based selection, and imperils reproducibility of findings and follow-up study design. Several methods correcting for this selection bias have been proposed, but questions remain on their relative performance and susceptibility to dependence between features since comparative studies are missing.
Results We demonstrate how empirical Bayes methods tackling selection bias require a custom density estimator for competitive performance under dependence. Furthermore, we perform a comprehensive simulation study comparing different classes of correction methods for point estimates as well as confidence intervals, while studying the effect of dependence. We find a bootstrap method by Tan et al. [2015] and empirical Bayes methods to perform best overall for correcting the selection bias, although this correction does not always improve the feature ranking. We apply selection bias correction methods to a comparison of the best single-feature prediction model versus the multi-feature model in predicting Brassica napus phenotypes from gene expression data, demonstrating that the superiority of the best single-feature model may be illusory.
Availability All R-code used for the analysis is included in the Supplementary information.
Contact steven.maere{at}psb.vib-ugent.be
Supplementary information Supplementary data are available at Bioinformatics online.
### Competing Interest Statement
The authors have declared no competing interest.
更多查看译文
关键词
empirical bayes,dependence
AI 理解论文
溯源树
样例
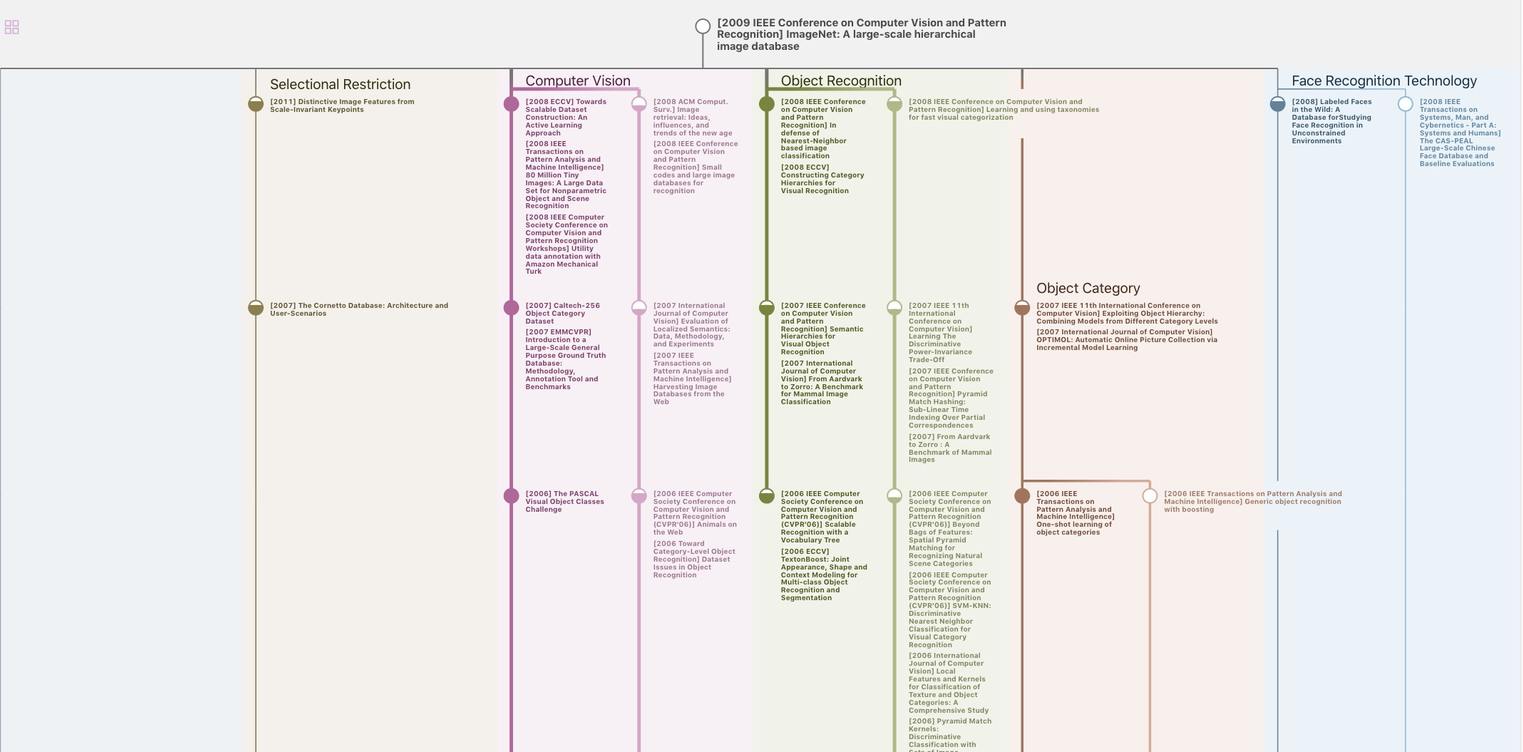
生成溯源树,研究论文发展脉络
Chat Paper
正在生成论文摘要