Uncovering Invariant Representations in Functional Neuroimaging with Deep Metric Learning
bioRxiv (Cold Spring Harbor Laboratory)(2023)
摘要
With the increasing ability to record neuroimaging with higher spatial and temporal resolution, there is a growing need for methods that reduce these high-dimensional representations into latent low-dimensional structures that are discriminative and/or predictive of behavior, disease, or in general experimental context. We propose a metric learning framework to extract meaningful latent structures from high-dimensional fMRI data. This method learns the latent embeddings that reduce the intra-group variability while maximizing the inter-group variability. In addition, our method leverages advances in few-shot learning approaches to adapt to small sample-size fMRI datasets, allowing one to learn the latent structure from just a few samples per context. We evaluate our work on two publicly available fMRI datasets and report superior results compared to popular alternative approaches such as Principal Component Analysis (84.7% vs. 60%; 21.8% vs. 8.3%). We provide the Python code as open-source at Github.
### Competing Interest Statement
The authors have declared no competing interest.
更多查看译文
关键词
invariant representations,functional,learning
AI 理解论文
溯源树
样例
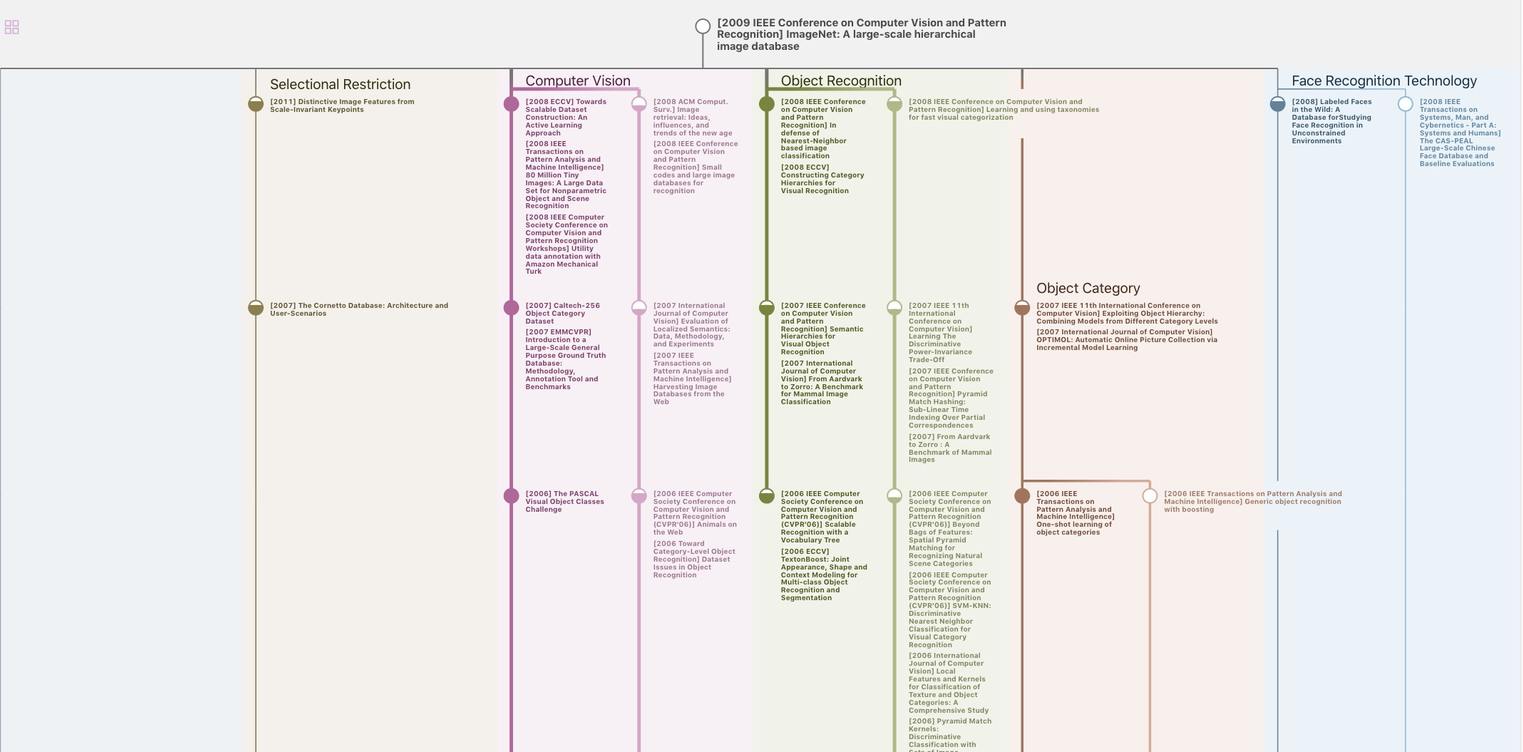
生成溯源树,研究论文发展脉络
Chat Paper
正在生成论文摘要