Tissue-specific transfer-learning enables retasking of a general comprehensive model to a specific domain
bioRxiv (Cold Spring Harbor Laboratory)(2023)
摘要
Machine learning (ML) has proven successful in biological data analysis. However, may require massive training data. To allow broader use of ML in the full spectrum of biology and medicine, including sample-sparse domains, re-directing established models to specific tasks by add-on training via a moderate sample may be promising. Transfer learning (TL), a technique migrating pre-trained models to new tasks, fits in this requirement. Here, by TL, we retasked Enformer, a comprehensive model trained by massive data, tailored to breast cancers using breast-specific data. Its performance has been validated through statistical accuracy of predictions, annotation of genetic variants, and mapping of variants associated with breast cancer. By allowing the flexibility of adding dedicated training data, our TL protocol unlocks future discovery within specific domains with moderate add-on samples by standing on the shoulders of giant models.
### Competing Interest Statement
The authors have declared no competing interest.
更多查看译文
关键词
transfer-learning transfer-learning,tissue-specific
AI 理解论文
溯源树
样例
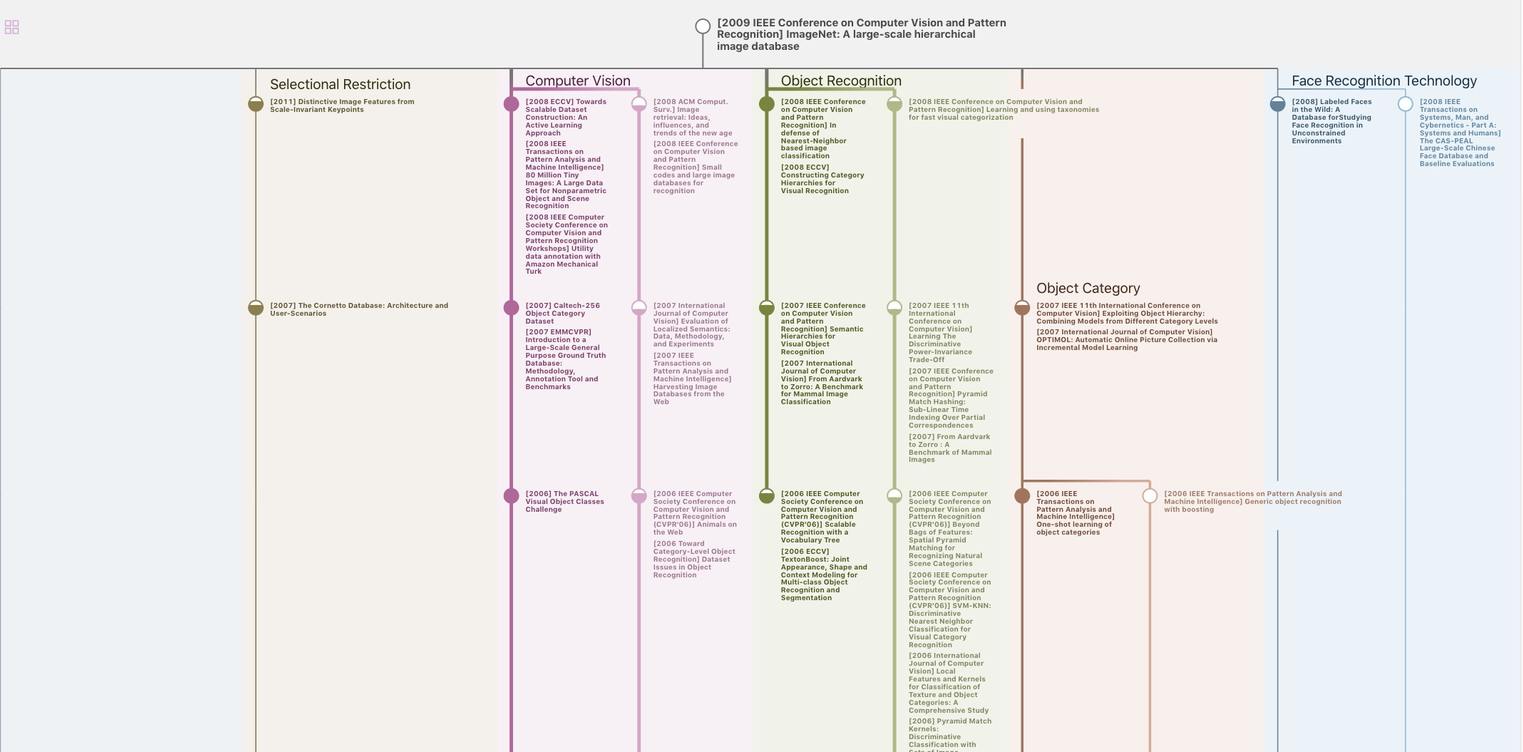
生成溯源树,研究论文发展脉络
Chat Paper
正在生成论文摘要