Proteome-scale prediction of molecular mechanisms underlying dominant genetic diseases
bioRxiv (Cold Spring Harbor Laboratory)(2024)
摘要
Many dominant genetic disorders result from protein-altering mutations, acting primarily through dominant-negative (DN), gain-of-function (GOF), and loss-of-function (LOF) mechanisms. Deciphering the mechanisms by which dominant diseases exert their effects is often experimentally challenging and resource intensive, but is essential for developing appropriate therapeutic approaches. Diseases that arise via a LOF mechanism are more amenable to be treated by conventional gene therapy, whereas DN and GOF mechanisms may require gene editing or targeting by small molecules. Moreover, pathogenic missense mutations that act via DN and GOF mechanisms are more difficult to identify than those that act via LOF using nearly all currently available variant effect predictors. Here, we introduce a tripartite statistical model made up of support vector machine binary classifiers trained to predict whether human protein coding genes are likely to be associated with DN, GOF, or LOF molecular disease mechanisms. We test the utility of the predictions by examining biologically and clinically meaningful properties known to be associated with the mechanisms. Our results strongly support that the models are able to generalise on unseen data and offer insight into the functional attributes of proteins associated with different mechanisms. We hope that our predictions will serve as a springboard for researchers studying novel variants and those of uncertain clinical significance, guiding variant interpretation strategies and experimental characterisation. Predictions for the human UniProt reference proteome are available at https://osf.io/z4dcp/.
### Competing Interest Statement
The authors have declared no competing interest.
更多查看译文
关键词
molecular mechanisms,diseases,proteome-scale
AI 理解论文
溯源树
样例
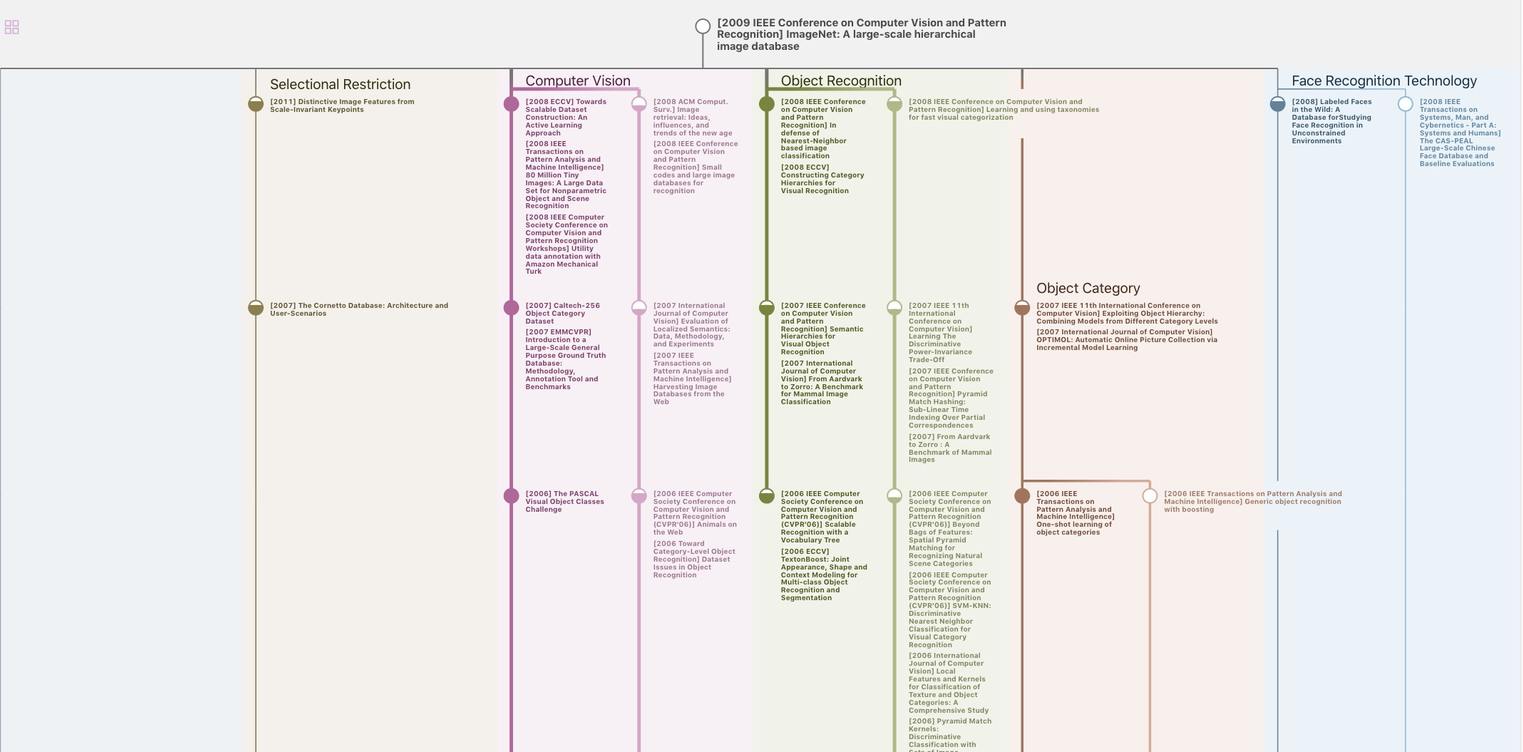
生成溯源树,研究论文发展脉络
Chat Paper
正在生成论文摘要