Towards deep-learning (DL) based fully automated target delineation for rectal cancer neoadjuvant radiotherapy using a divide-and-conquer strategy: a study with multicenter blind and randomized validation
Radiation Oncology(2023)
摘要
Purpose Manual clinical target volume (CTV) and gross tumor volume (GTV) delineation for rectal cancer neoadjuvant radiotherapy is pivotal but labor-intensive. This study aims to propose a deep learning (DL)-based workflow towards fully automated clinical target volume (CTV) and gross tumor volume (GTV) delineation for rectal cancer neoadjuvant radiotherapy. Materials & methods We retrospectively included 141 patients with Stage II-III mid-low rectal cancer and randomly grouped them into training (n = 121) and testing (n = 20) cohorts. We adopted a divide-and-conquer strategy to address CTV and GTV segmentation using two separate DL models with DpuUnet as backend-one model for CTV segmentation in the CT domain, and the other for GTV in the MRI domain. The workflow was validated using a three-level multicenter-involved blind and randomized evaluation scheme. Dice similarity coefficient (DSC) and 95th percentile Hausdorff distance (95HD) metrics were calculated in Level 1, four-grade expert scoring was performed in Level 2, and head-to-head Turing test in Level 3. Results For the DL-based CTV contours over the testing cohort, the DSC and 95HD (mean ± SD) were 0.85 ± 0.06 and 7.75 ± 6.42 mm respectively, and 96.4% cases achieved clinical viable scores (≥ 2). The positive rate in the Turing test was 52.3%. For GTV, the DSC and 95HD were 0.87 ± 0.07 and 4.07 ± 1.67 mm respectively, and 100% of the DL-based contours achieved clinical viable scores (≥ 2). The positive rate in the Turing test was 52.0%. Conclusion The proposed DL-based workflow exhibited promising accuracy and excellent clinical viability towards automated CTV and GTV delineation for rectal cancer neoadjuvant radiotherapy.
更多查看译文
关键词
Rectal cancer radiotherapy,Deep learning,CTV,GTV,Segmentation
AI 理解论文
溯源树
样例
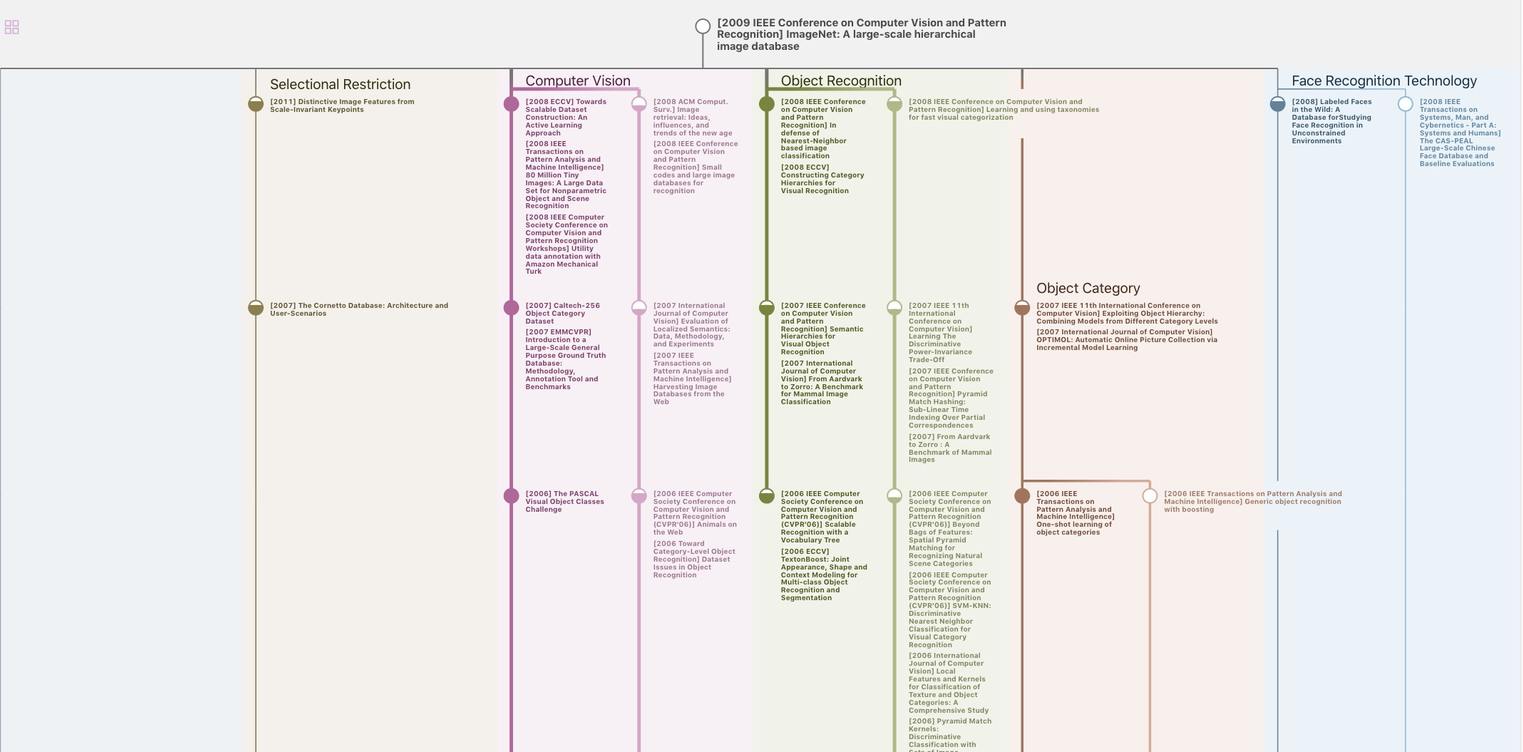
生成溯源树,研究论文发展脉络
Chat Paper
正在生成论文摘要