Performance Optimization for Noise Interference Privacy Protection in Federated Learning
IEEE Transactions on Cognitive Communications and Networking(2023)
摘要
The data security issue in federated learning is critical. While federated learning allows clients to collaboratively participate in the global model training without sharing private data, external eavesdroppers may intercept the model uploaded by the client to the server, revealing some sensitive information. Noise interference, i.e., adding noise to the client model before transmission, is an effective and efficient privacy-preserving method, but it degrades the learning performance of the system at the same time. In this paper, to address the challenge of system performance degradation caused by noise interference, we propose the FedNoise algorithm, which adopts two separate learning rates at the client and server respectively. By carefully tuning these learning rates, the global model can converge to the optimum. We provide theoretical proofs of the convergence of FedNoise for both strongly convex and non-convex loss functions and conduct simulations on real tasks. Numerical experimental results demonstrate that, under the same privacy protection level, FedNoise significantly outperforms the state-of-art scheme on datasets MNIST, Fashion-MNIST, and CIFAR10.
更多查看译文
关键词
Federated learning,privacy protection,noise interference,convergence analysis
AI 理解论文
溯源树
样例
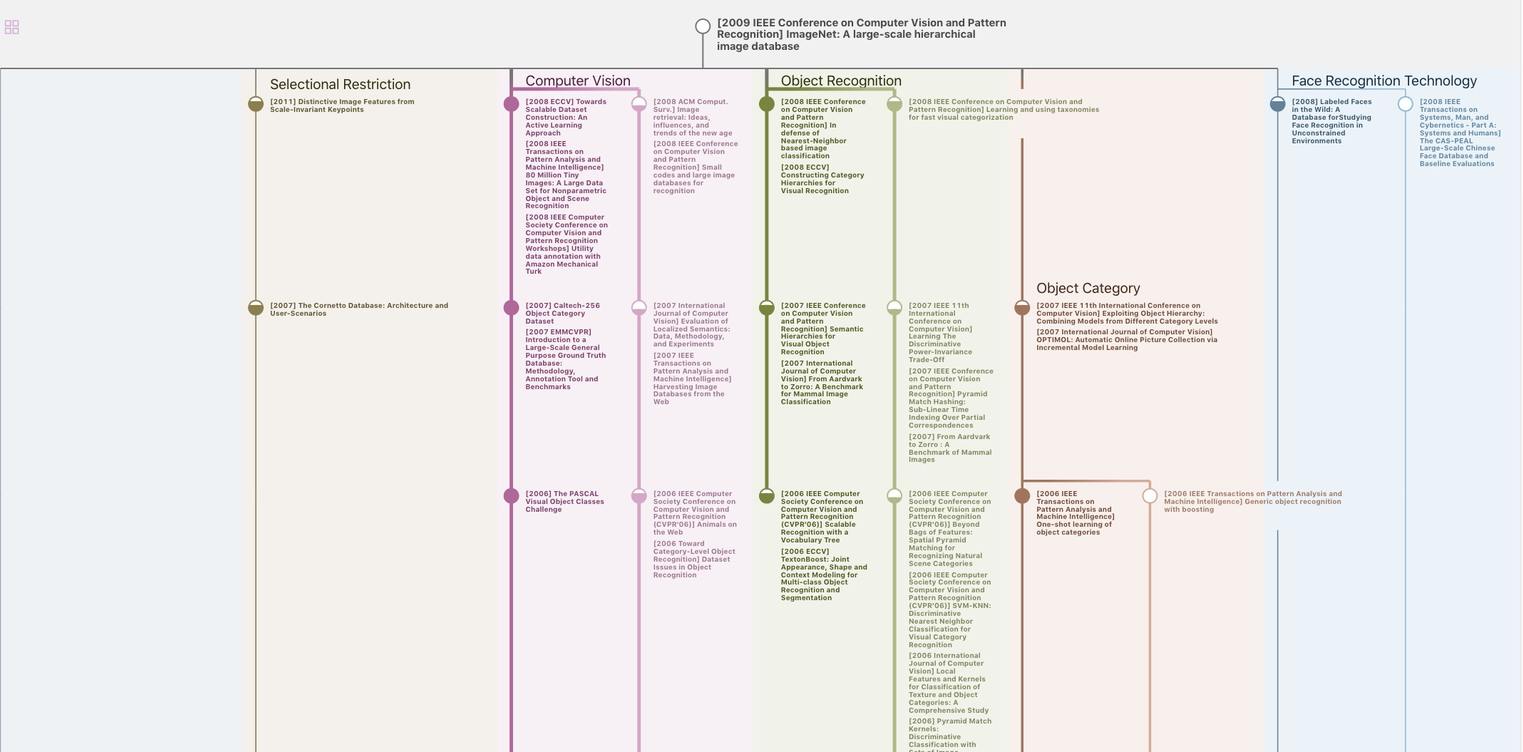
生成溯源树,研究论文发展脉络
Chat Paper
正在生成论文摘要