Quantifying Prediction Uncertainty in Regression Using Random Fuzzy Sets: The ENNreg Model
IEEE Transactions on Fuzzy Systems(2023)
摘要
In this article, we introduce a neural network model for regression in which prediction uncertainty is quantified by Gaussian random fuzzy numbers (GRFNs), a newly introduced family of random fuzzy subsets of the real line that generalizes both Gaussian random variables and Gaussian possibility distributions. The output GRFN is constructed by combining GRFNs induced by prototypes using a combination operator that generalizes Dempster's rule of evidence theory. The three output units indicate the most plausible value of the response variable, variability around this value, and epistemic uncertainty. The network is trained by minimizing a loss function that generalizes the negative log-likelihood. Comparative experiments show that this method is competitive, both in terms of prediction accuracy and calibration error, with state-of-the-art techniques such as random forests or deep learning with Monte Carlo dropout. In addition, the model outputs a predictive belief function that can be shown to be calibrated, in the sense that it allows us to compute conservative prediction intervals with specified belief degree.
更多查看译文
关键词
prediction uncertainty,random fuzzy sets,regression
AI 理解论文
溯源树
样例
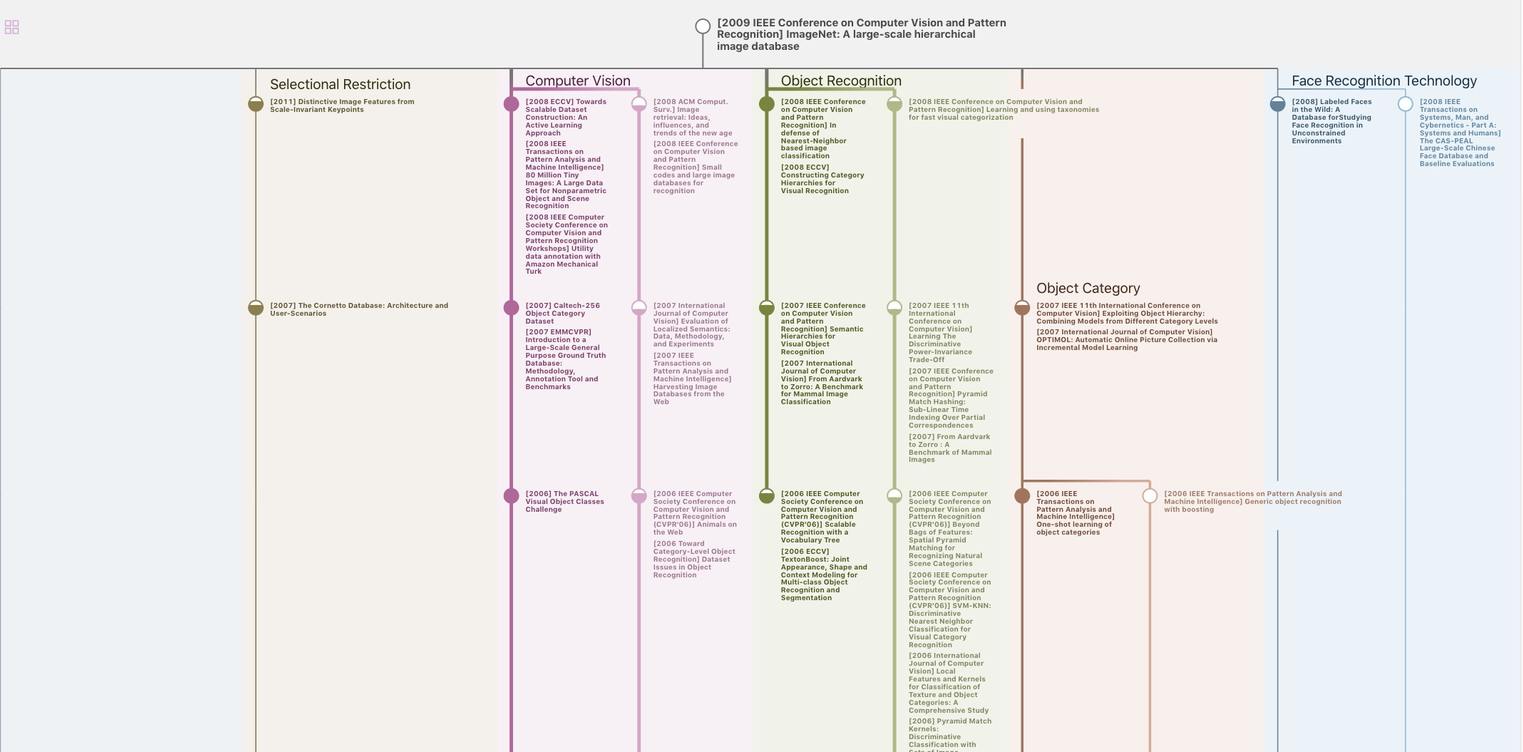
生成溯源树,研究论文发展脉络
Chat Paper
正在生成论文摘要