Interpreting frequentist hypothesis tests: insights from Bayesian inference
Canadian journal of anaesthesia = Journal canadien d'anesthesie(2023)
摘要
Randomized controlled trials are one of the best ways of quantifying the effectiveness of medical interventions. Therefore, when the authors of a randomized superiority trial report that differences in the primary outcome between the intervention group and the control group are “significant” (i.e., P ≤ 0.05), we might assume that the intervention has an effect on the outcome. Similarly, when differences between the groups are “not significant,” we might assume that the intervention does not have an effect on the outcome. Nevertheless, both assumptions are frequently incorrect. In this article, we explore the relationship that exists between real treatment effects and declarations of statistical significance based on P values and confidence intervals. We explain why, in some circumstances, the chance an intervention is ineffective when P ≤ 0.05 exceeds 25% and the chance an intervention is effective when P > 0.05 exceeds 50%. Over the last decade, there has been increasing interest in Bayesian methods as an alternative to frequentist hypothesis testing. We provide a robust but nontechnical introduction to Bayesian inference and explain why a Bayesian posterior distribution overcomes many of the problems associated with frequentist hypothesis testing. Notwithstanding the current interest in Bayesian methods, frequentist hypothesis testing remains the default method for statistical inference in medical research. Therefore, we propose an interim solution to the “significance problem” based on simplified Bayesian metrics (e.g., Bayes factor, false positive risk) that can be reported along with traditional P values and confidence intervals. We calculate these metrics for four well-known multicentre trials. We provide links to online calculators so readers can easily estimate these metrics for published trials. In this way, we hope decisions on incorporating the results of randomized trials into clinical practice can be enhanced, minimizing the chance that useful treatments are discarded or that ineffective treatments are adopted.
更多查看译文
关键词
frequentist hypothesis tests,bayesian inference,interpreting
AI 理解论文
溯源树
样例
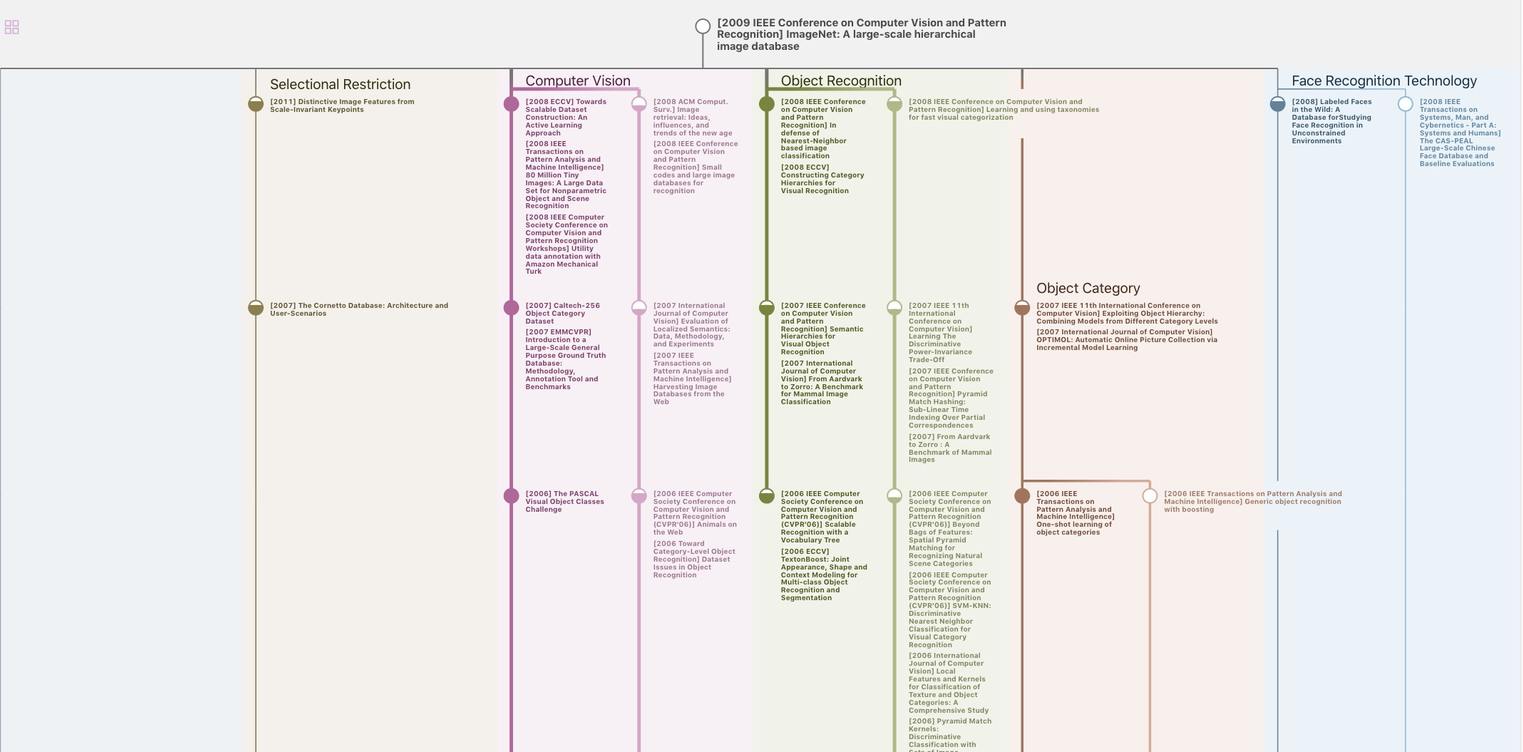
生成溯源树,研究论文发展脉络
Chat Paper
正在生成论文摘要