Convenient intelligent diagnosis for rotating machinery: An improved deep forest method based on feature reconstruction
ISA TRANSACTIONS(2023)
摘要
Due to the advantages of feature autoextraction and deep architecture, deep learning-based intelligent fault diagnosis has attracted increasing attention. However, a variety of complex hyper-parameter settings greatly limit its practical applications. Moreover, it is more critical and difficult to diagnose multiple mixed faults of rotating machinery under small training samples. To bridge these gaps, this paper proposes a convenient intelligent diagnostic method based on the improved deep forest, where a feature reconstruction algorithm is used to address the high computational cost and feature submergence caused by the long time series characteristics of vibration data. Comparison experiments with typical deep neural network-based methods are implemented, and the results validate the effectiveness and superiority of the proposed method, as well as the robustness of the hyper-parameters.
更多查看译文
关键词
Small training samples,Intelligent diagnosis,Multiple mixed faults,Reliability
AI 理解论文
溯源树
样例
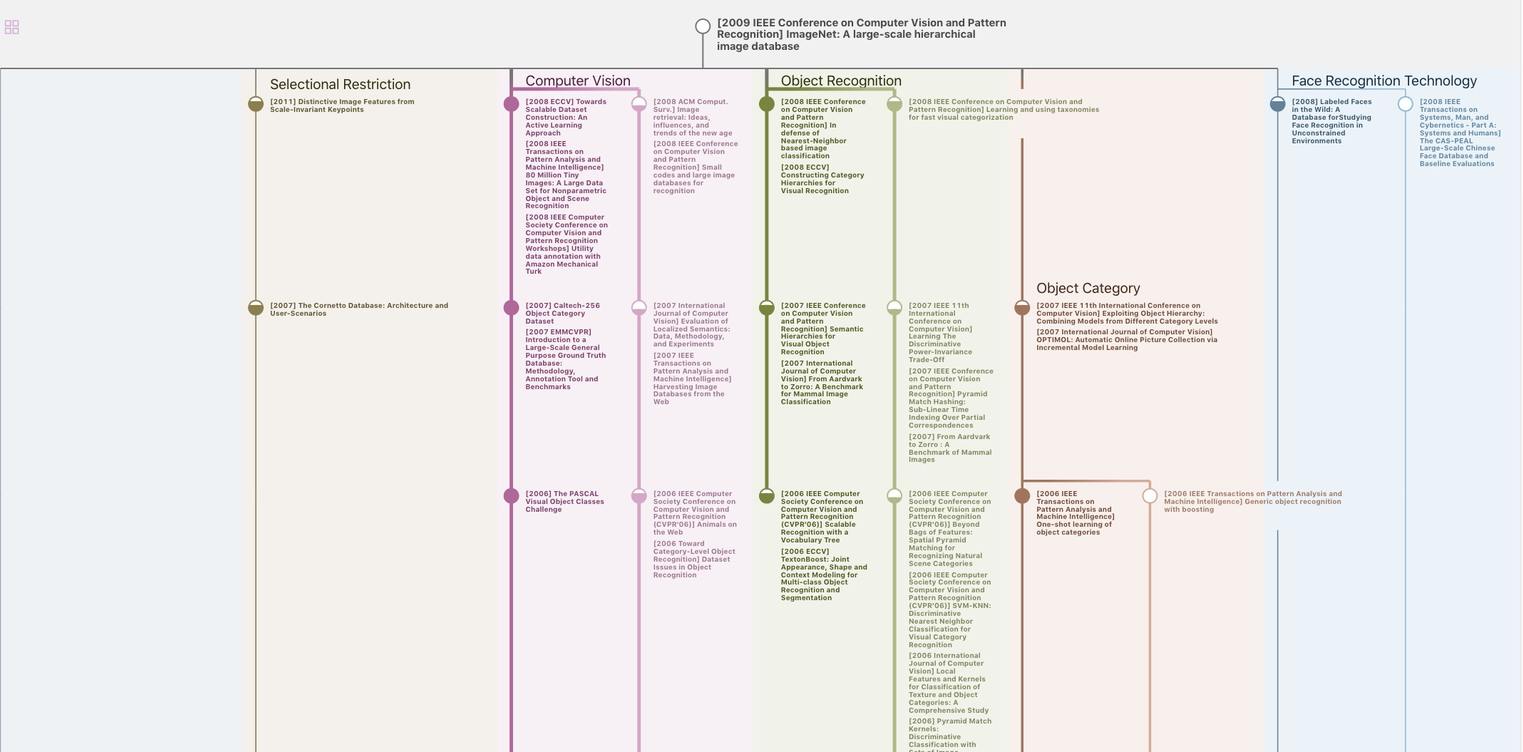
生成溯源树,研究论文发展脉络
Chat Paper
正在生成论文摘要