GoLLIE: Annotation Guidelines improve Zero-Shot Information-Extraction
ICLR 2024(2023)
摘要
Large Language Models (LLMs) combined with instruction tuning have made significant progress when generalizing to unseen tasks. However, they have been less successful in Information Extraction (IE), lagging behind task-specific models. Typically, IE tasks are characterized by complex annotation guidelines which describe the task and give examples to humans. Previous attempts to leverage such information have failed, even with the largest models, as they are not able to follow the guidelines out-of-the-box. In this paper we propose GoLLIE (Guideline-following Large Language Model for IE), a model able to improve zero-shot results on unseen IE tasks by virtue of being fine-tuned to comply with annotation guidelines. Comprehensive evaluation empirically demonstrates that GoLLIE is able to generalize to and follow unseen guidelines, outperforming previous attempts at zero-shot information extraction. The ablation study shows that detailed guidelines is key for good results.
更多查看译文
关键词
Information Extraction,Zero-Shot,Annotation Guidelines,Large Language Models,LLM,prompt
AI 理解论文
溯源树
样例
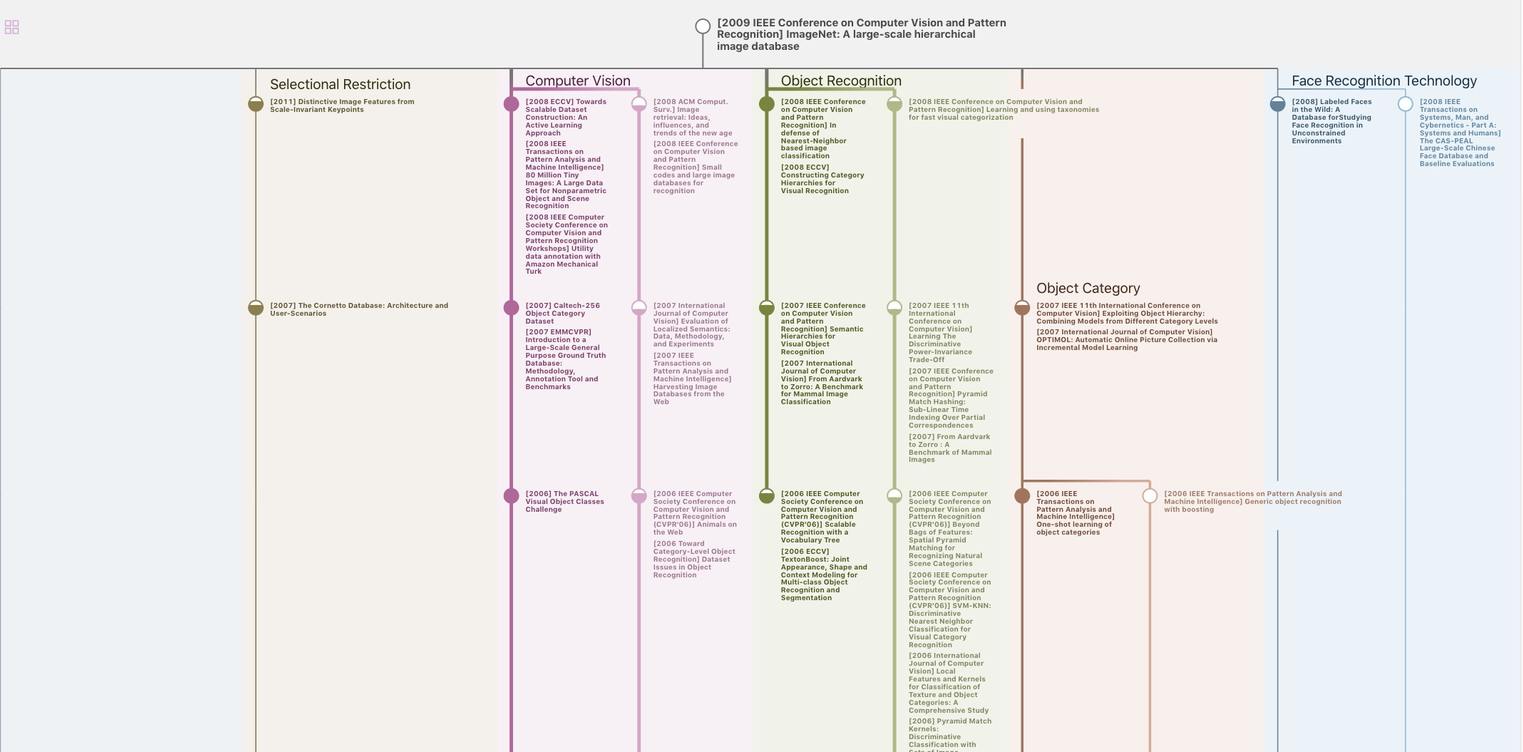
生成溯源树,研究论文发展脉络
Chat Paper
正在生成论文摘要