Controllable Multi-document Summarization: Coverage & Coherence Intuitive Policy with Large Language Model Based Rewards
CoRR(2023)
摘要
Memory-efficient large language models are good at refining text input for better readability. However, controllability is a matter of concern when it comes to text generation tasks with long inputs, such as multi-document summarization. In this work, we investigate for a generic controllable approach for multi-document summarization that leverages the capabilities of LLMs to refine the text. In particular, we train a controllable content extraction scheme to extract the text that will be refined by an LLM. The scheme is designed with a novel coverage and coherence intuitive policy, which is duly rewarded by a passively trained LLM. Our approach yields competitive results in the evaluation using ROUGE metrics and outperforms potential baselines in coherence, as per human evaluation.
更多查看译文
关键词
intuitive policy,language,multi-document
AI 理解论文
溯源树
样例
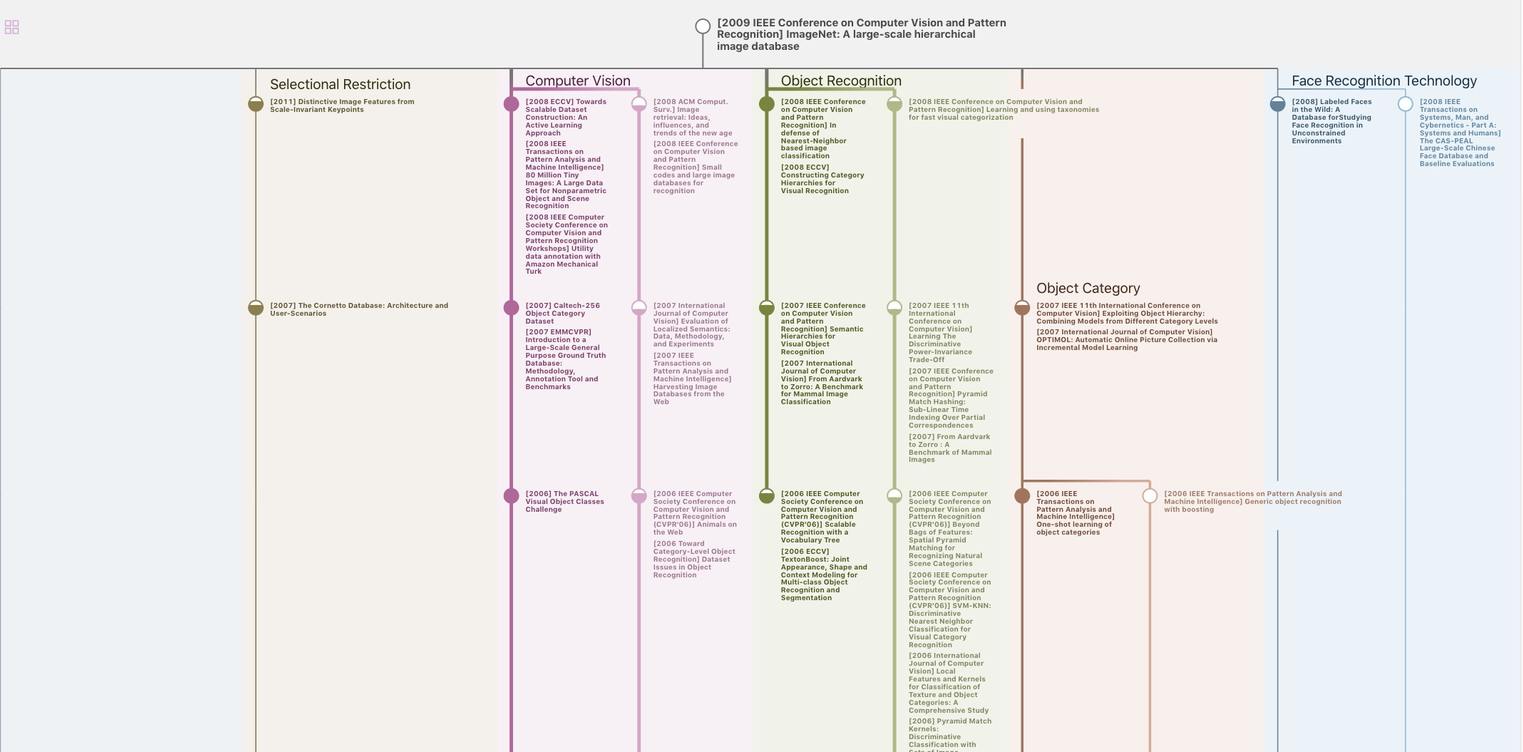
生成溯源树,研究论文发展脉络
Chat Paper
正在生成论文摘要